Correlation Based Feature Subset Selection for Multivariate Time-Series Data
arxiv(2021)
摘要
Correlations in streams of multivariate time series data means that typically, only a small subset of the features are required for a given data mining task. In this paper, we propose a technique which we call Merit Score for Time-Series data (MSTS) that does feature subset selection based on the correlation patterns of single feature classifier outputs. We assign a Merit Score to the feature subsets which is used as the basis for selecting 'good' feature subsets. The proposed technique is evaluated on datasets from the UEA multivariate time series archive and is compared against a Wrapper approach for feature subset selection. MSTS is shown to be effective for feature subset selection and is in particular effective as a data reduction technique. MSTS is shown here to be computationally more efficient than the Wrapper strategy in selecting a suitable feature subset, being more than 100 times faster for some larger datasets while also maintaining a good classification accuracy.
更多查看译文
关键词
time-series
AI 理解论文
溯源树
样例
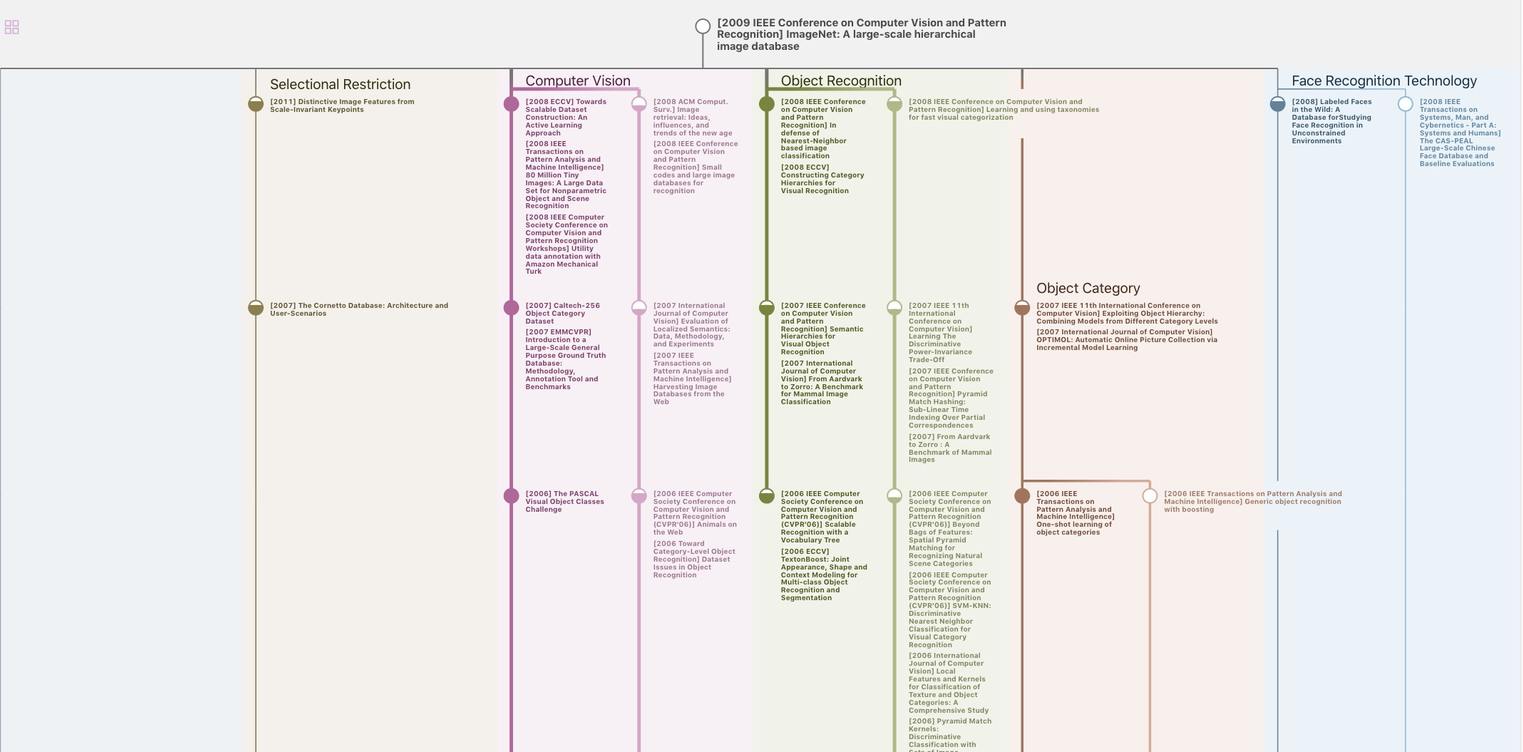
生成溯源树,研究论文发展脉络
Chat Paper
正在生成论文摘要