Forecasting Brain Activity Based on Models of Spatio-Temporal Brain Dynamics: A Comparison of Graph Neural Network Architectures
arXiv (Cornell University)(2021)
摘要
Comprehending the interplay between spatial and temporal characteristics of neural dynamics can contribute to our understanding of information processing in the human brain. Graph neural networks (GNNs) provide a new possibility to interpret graph structured signals like those observed in complex brain networks. In our study we compare different spatio-temporal GNN architectures and study their ability to model neural activity distributions obtained in functional MRI (fMRI) studies. We evaluate the performance of the GNN models on a variety of scenarios in MRI studies and also compare it to a VAR model, which is currently often used for directed functional connectivity analysis. We show that by learning localized functional interactions on the anatomical substrate, GNN based approaches are able to robustly scale to large network studies, even when available data are scarce. By including anatomical connectivity as the physical substrate for information propagation, such GNNs also provide a multi-modal perspective on directed connectivity analysis, offering a novel possibility to investigate the spatio-temporal dynamics in brain networks.
更多查看译文
关键词
Brain connectivity,Graph neural networks,Structure-function relationship,Directed connectivity
AI 理解论文
溯源树
样例
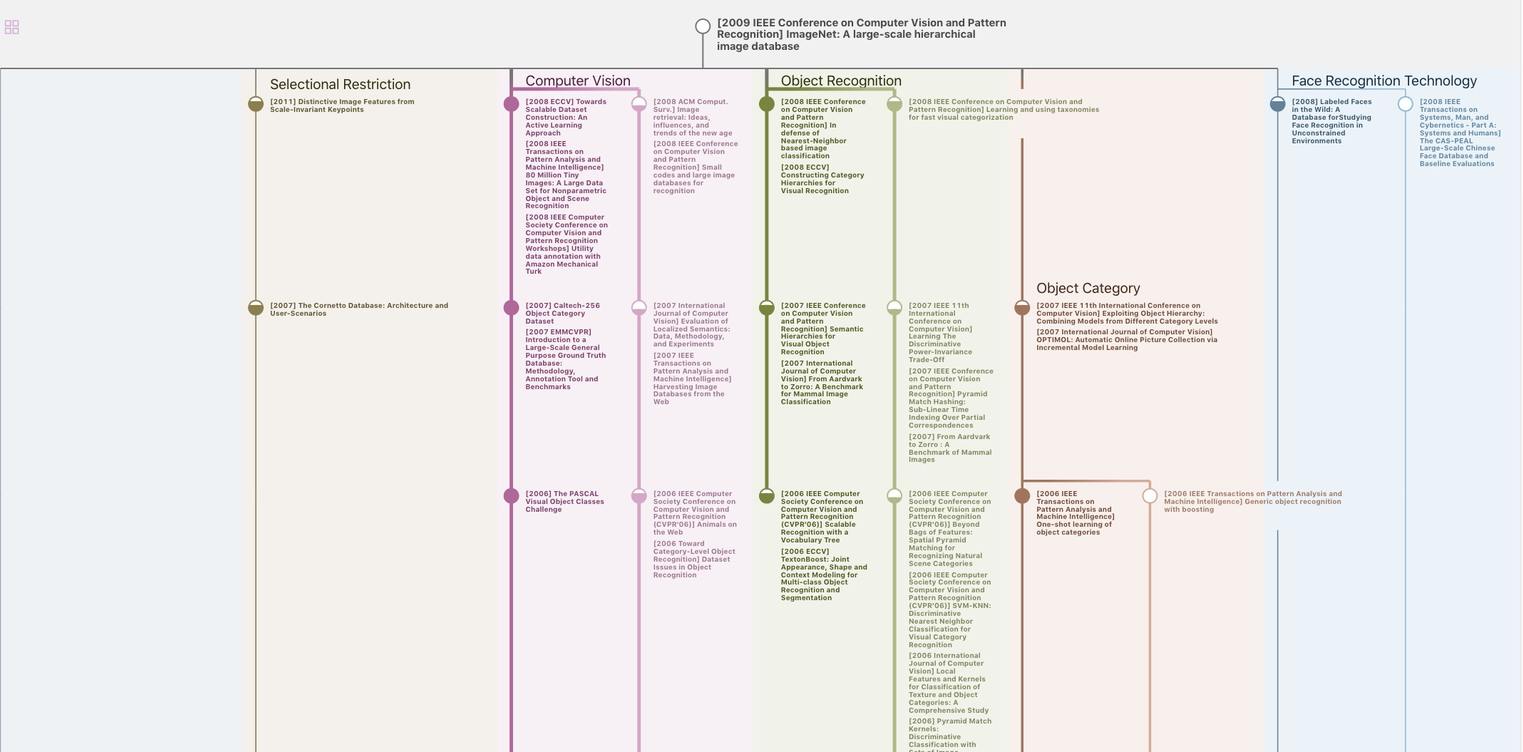
生成溯源树,研究论文发展脉络
Chat Paper
正在生成论文摘要