Multi-view Spectral Clustering with Adaptive Graph Learning and Tensor Schatten p-norm
Neurocomputing(2022)
摘要
Although the promising clustering performance, existing graph-based multi-view clustering methods still have the following deficiencies. (1) They cannot well exploit the salient difference between graphs of different views, resulting in suboptimal graphs. (2) They minimize the divergence between graphs of different views by one-dimensional, element by element metric model. Thus, they cannot well exploit the complementary information embedded in graphs. (3) They fail to simultaneously take local and global intrinsic geometric structures into account, resulting in suboptimal clustering performance. To handle the aforementioned problems, we propose Multi-view Spectral Clustering with Adaptive Graph Learning and Tensor Schatten p-norm. Specifically, we present an adaptive weighted strategy that directly takes into account the contribution among different views for clustering. To well learn a good graph, which well characterizes cluster structure, we integrate local and global structure learning into a unified framework and leverage tensor Schatten p-norm regularizer to minimize the divergence between graphs of different views. Extensive experiments on benchmark datasets have demonstrated the superiority of the proposed method.
更多查看译文
关键词
Unsupervised learning,Multi-view spectral clustering,Graph learning
AI 理解论文
溯源树
样例
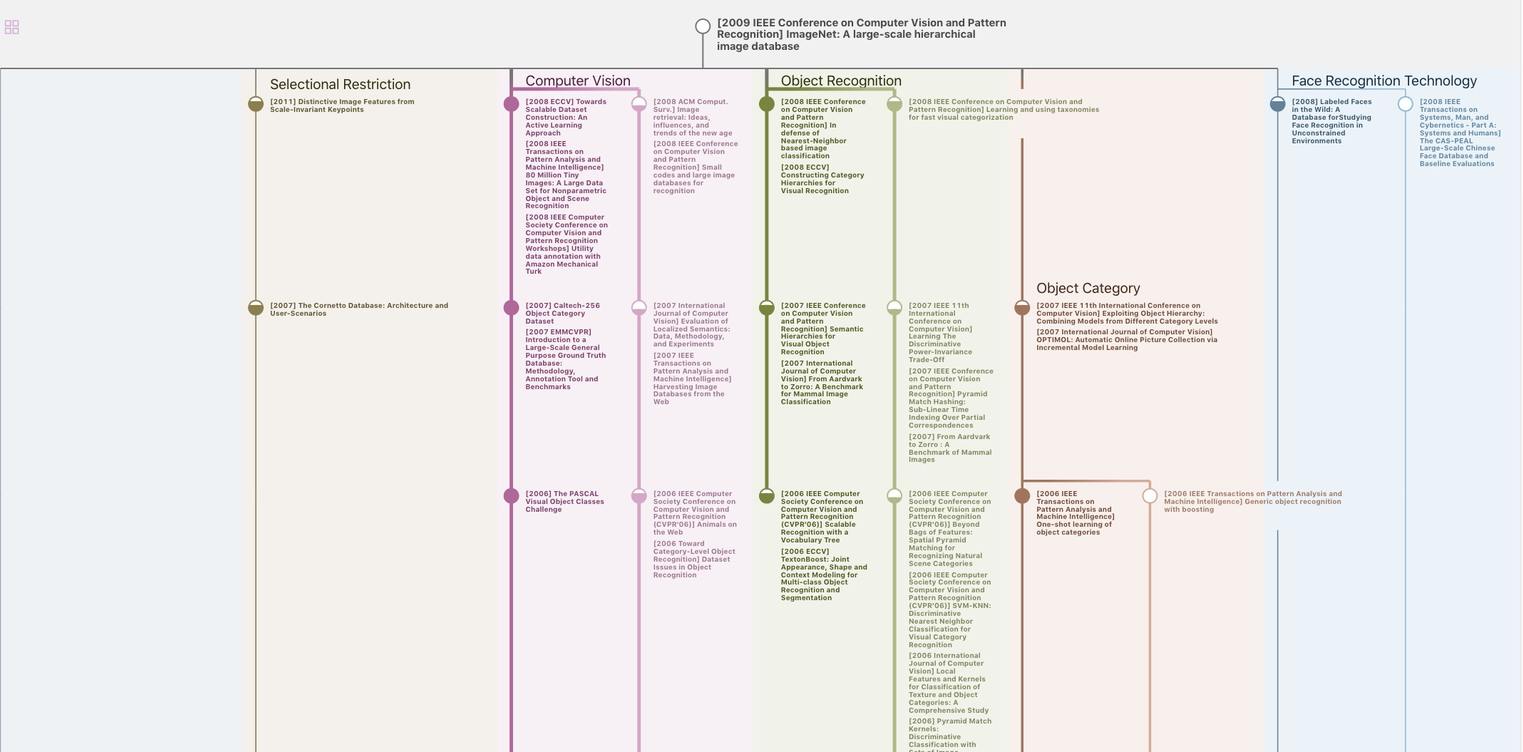
生成溯源树,研究论文发展脉络
Chat Paper
正在生成论文摘要