Vision-Based Driver's Cognitive Load Classification Considering Eye Movement Using Machine Learning and Deep Learning
SENSORS(2021)
摘要
Due to the advancement of science and technology, modern cars are highly technical, more activity occurs inside the car and driving is faster; however, statistics show that the number of road fatalities have increased in recent years because of drivers' unsafe behaviors. Therefore, to make the traffic environment safe it is important to keep the driver alert and awake both in human and autonomous driving cars. A driver's cognitive load is considered a good indication of alertness, but determining cognitive load is challenging and the acceptance of wire sensor solutions are not preferred in real-world driving scenarios. The recent development of a non-contact approach through image processing and decreasing hardware prices enables new solutions and there are several interesting features related to the driver's eyes that are currently explored in research. This paper presents a vision-based method to extract useful parameters from a driver's eye movement signals and manual feature extraction based on domain knowledge, as well as automatic feature extraction using deep learning architectures. Five machine learning models and three deep learning architectures are developed to classify a driver's cognitive load. The results show that the highest classification accuracy achieved is 92% by the support vector machine model with linear kernel function and 91% by the convolutional neural networks model. This non-contact technology can be a potential contributor in advanced driver assistive systems.
更多查看译文
关键词
cognitive load, eye-movement, machine learning, non-contact
AI 理解论文
溯源树
样例
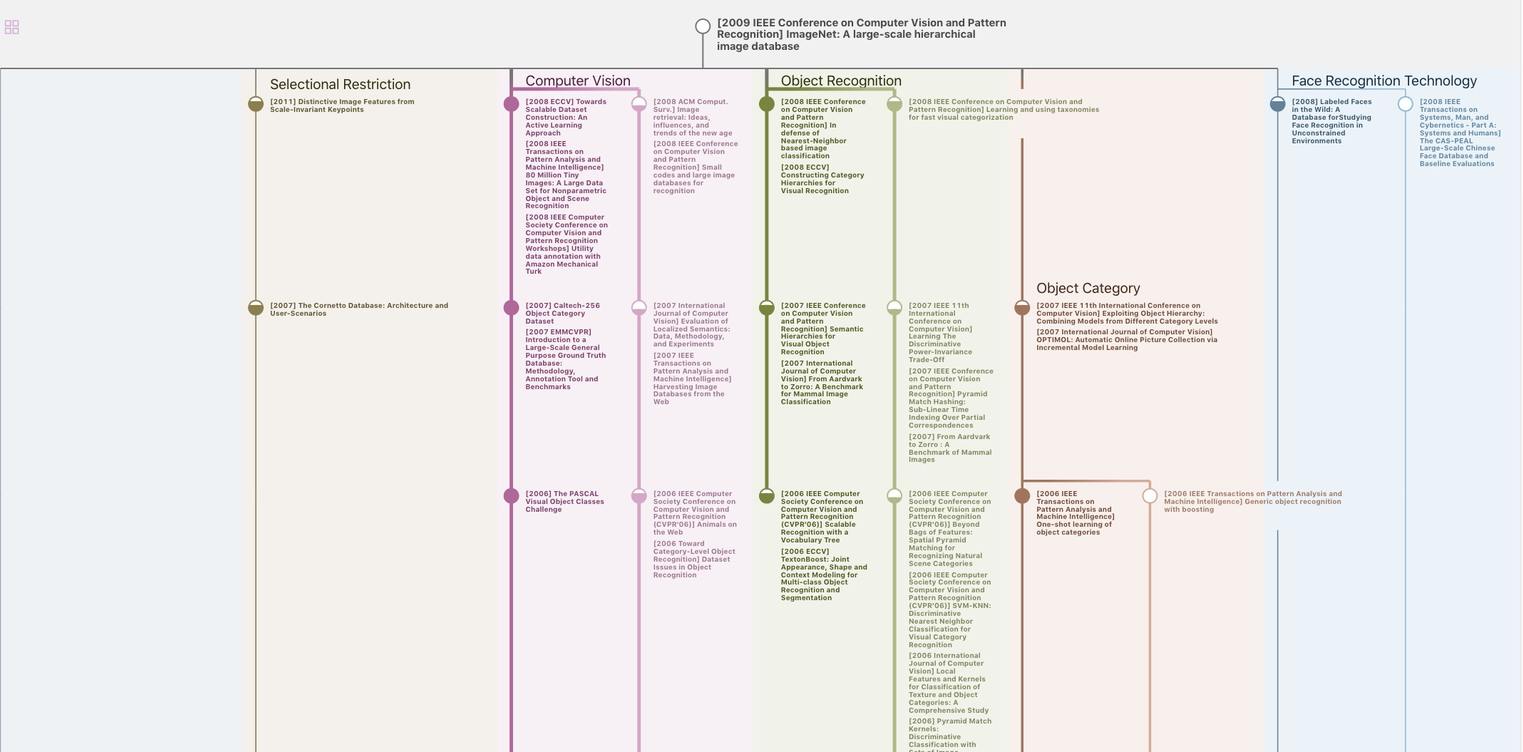
生成溯源树,研究论文发展脉络
Chat Paper
正在生成论文摘要