Ship Detection in Sentinel 2 Multi-Spectral Images with Self-Supervised Learning
REMOTE SENSING(2021)
摘要
Automatic ship detection provides an essential function towards maritime domain awareness for security or economic monitoring purposes. This work presents an approach for training a deep learning ship detector in Sentinel-2 multi-spectral images with few labeled examples. We design a network architecture for detecting ships with a backbone that can be pre-trained separately. By using self supervised learning, an emerging unsupervised training procedure, we learn good features on Sentinel-2 images, without requiring labeling, to initialize our network's backbone. The full network is then fine-tuned to learn to detect ships in challenging settings. We evaluated this approach versus pre-training on ImageNet and versus a classical image processing pipeline. We examined the impact of variations in the self-supervised learning step and we show that in the few-shot learning setting self-supervised pre-training achieves better results than ImageNet pre-training. When enough training data are available, our self-supervised approach is as good as ImageNet pre-training. We conclude that a better design of the self-supervised task and bigger non-annotated dataset sizes can lead to surpassing ImageNet pre-training performance without any annotation costs.
更多查看译文
关键词
ship detection, self-supervised learning, transfer learning, Sentinel 2 dataset
AI 理解论文
溯源树
样例
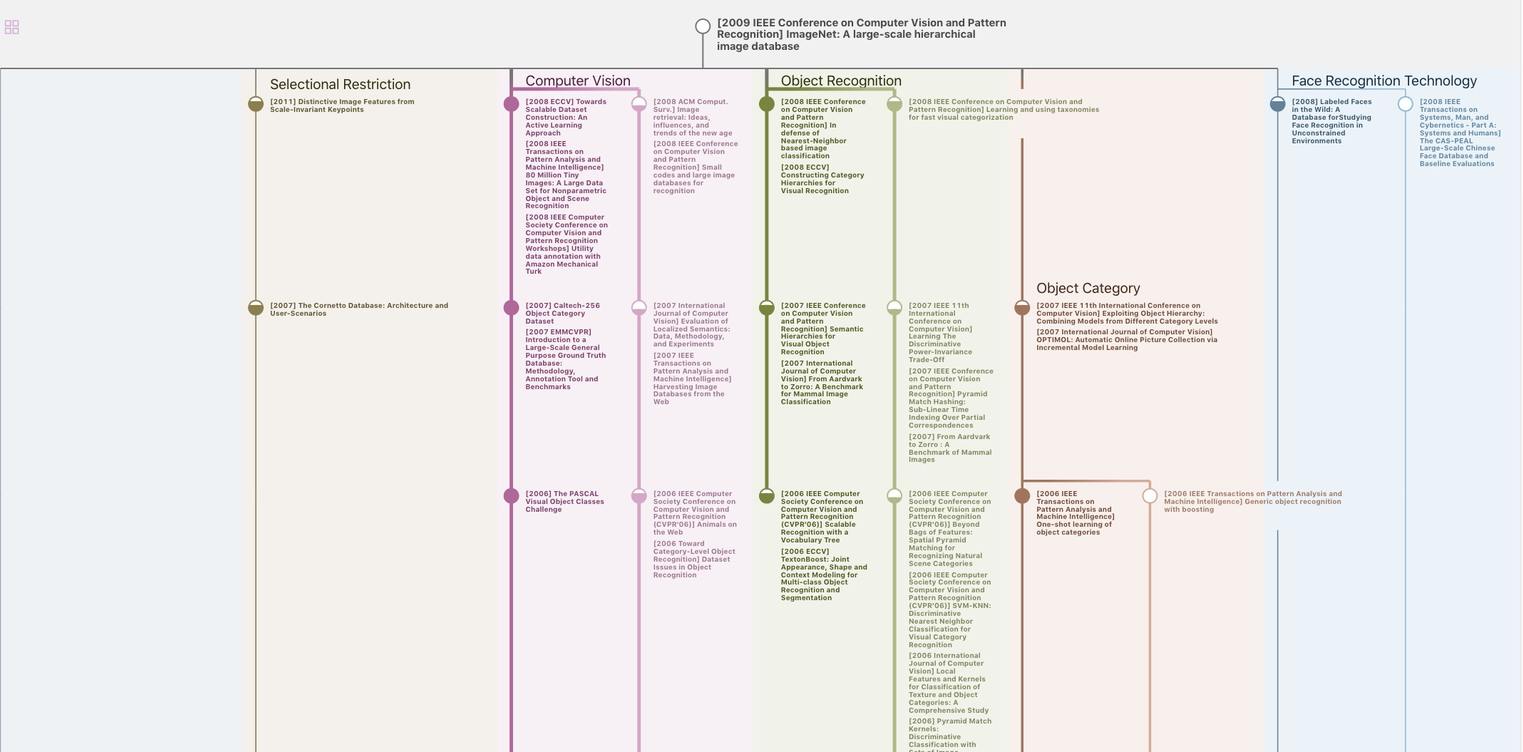
生成溯源树,研究论文发展脉络
Chat Paper
正在生成论文摘要