Semi-Automated Semantic Segmentation of Arctic Shorelines Using Very High-Resolution Airborne Imagery, Spectral Indices and Weakly Supervised Machine Learning Approaches
REMOTE SENSING(2021)
摘要
Precise coastal shoreline mapping is essential for monitoring changes in erosion rates, surface hydrology, and ecosystem structure and function. Monitoring water bodies in the Arctic National Wildlife Refuge (ANWR) is of high importance, especially considering the potential for oil and natural gas exploration in the region. In this work, we propose a modified variant of the Deep Neural Network based U-Net Architecture for the automated mapping of 4 Band Orthorectified NOAA Airborne Imagery using sparsely labeled training data and compare it to the performance of traditional Machine Learning (ML) based approaches-namely, random forest, xgboost-and spectral water indices-Normalized Difference Water Index (NDWI), and Normalized Difference Surface Water Index (NDSWI)-to support shoreline mapping of Arctic coastlines. We conclude that it is possible to modify the U-Net model to accept sparse labels as input and the results are comparable to other ML methods (an Intersection-over-Union (IoU) of 94.86% using U-Net vs. an IoU of 95.05% using the best performing method).
更多查看译文
关键词
land water segmentation, remote sensing, deep learning, sparse labels
AI 理解论文
溯源树
样例
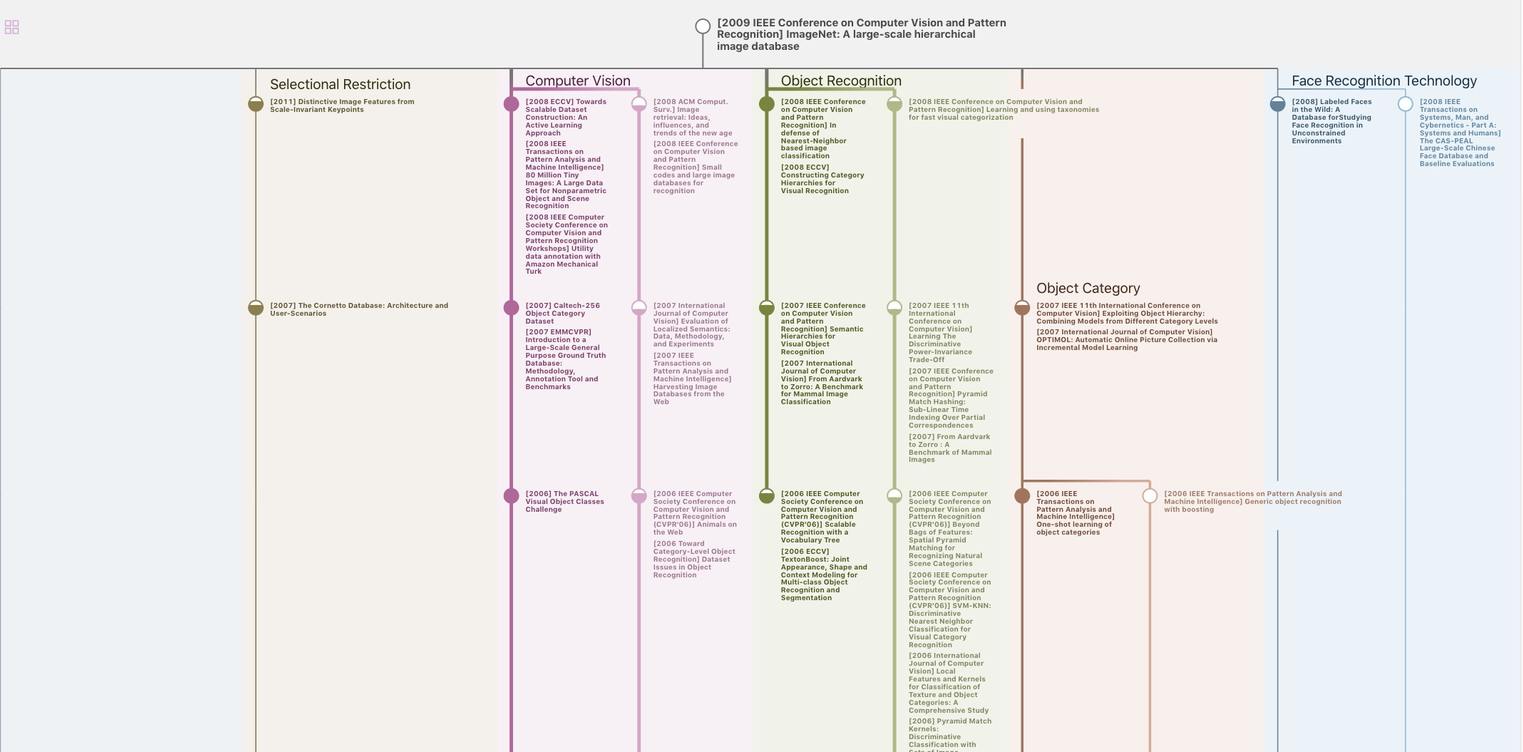
生成溯源树,研究论文发展脉络
Chat Paper
正在生成论文摘要