Unsupervised 3D Shape Coverage Estimation with Applications to Colonoscopy
IEEE International Conference on Computer Vision(2021)
摘要
Reconstructing shapes from partial and noisy 3D data is a well-studied problem, which in recent years has been dominated by data-driven techniques. Yet in a low data regime, these techniques struggle to provide fine and accurate reconstructions. Here we focus on the relaxed problem of estimating shape coverage, i.e. asking "how much of the shape was seen?" rather than "what was the original shape?" We propose a method for unsupervised shape coverage estimation, and validate that this task can be performed accurately in a low data regime. Shape coverage estimation can provide valuable insights which pave the way for innovative applications, as we demonstrate for the case of deficient coverage detection in colonoscopy screenings.
更多查看译文
关键词
unsupervised 3D,partial D data,noisy 3D data,data-driven techniques,low data regime,techniques struggle,fine reconstructions,accurate reconstructions,unsupervised shape coverage estimation,deficient coverage detection
AI 理解论文
溯源树
样例
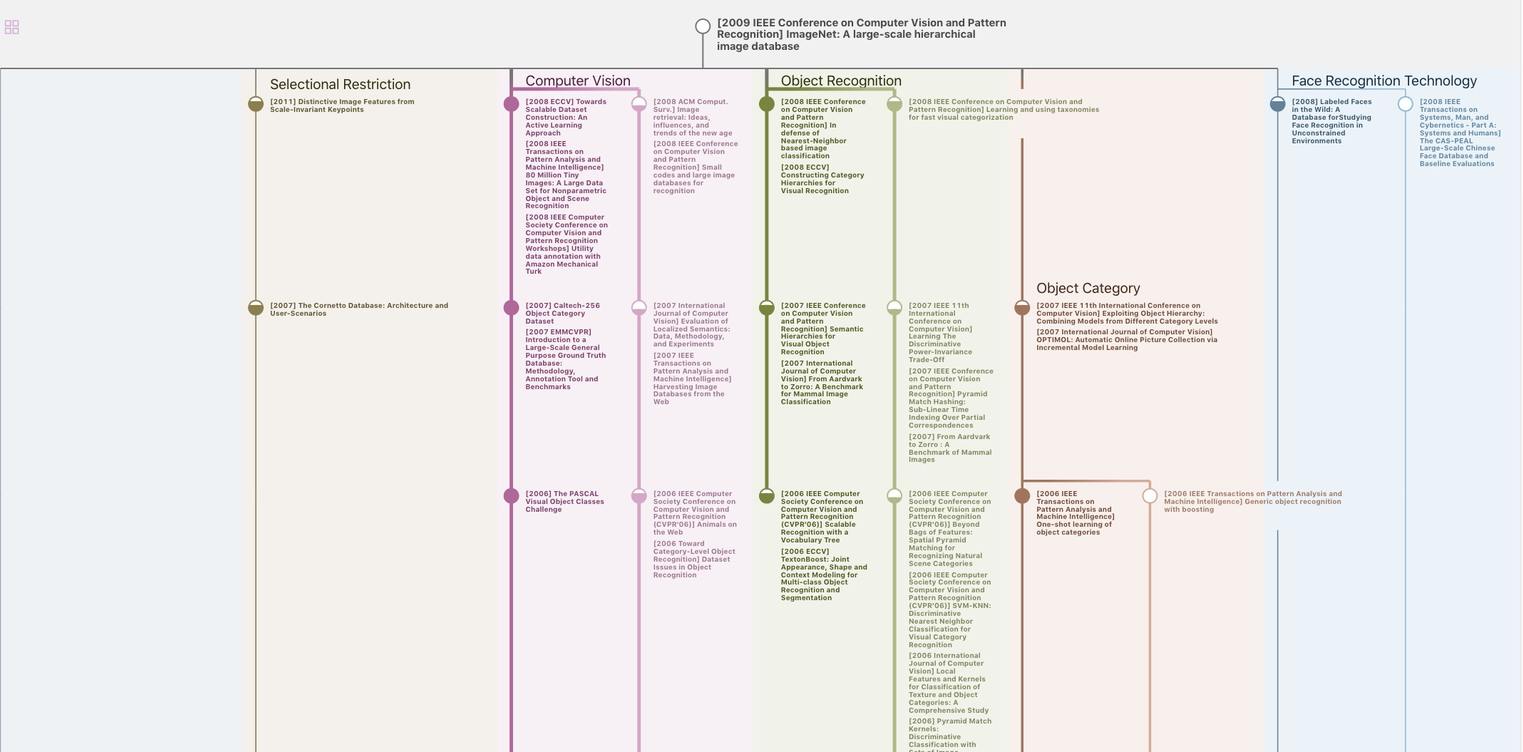
生成溯源树,研究论文发展脉络
Chat Paper
正在生成论文摘要