Super-resolution for in situ Plankton Images.
IEEE International Conference on Computer Vision(2021)
摘要
Being inherently limited by the wave properties of light, underwater plankton cameras compromise between their imaging resolution and field of view (FOV) for in situ observations. In order to enlarge the sampling volume in single frame acquisition, lower magnifications are usually adopted to enable larger FOV but sacrifice the resolution. In this paper, we build a real-underwater image dataset called IsPlanktonSR for in situ plankton image super-resolution (SR), in which paired low resolution (LR) and high resolution (HR) images of the same individual live planktonic organisms are captured by a customized dual-channel darkfield imaging system. An image registration algorithmic pipeline is also proposed to preprocess and align the image pairs at different scaling factors of 2 x and 4x. The IsPlanktonSR dataset is used to train an enhanced deep residual network for SR through the L2, the perceptual and the contextual losses, respectively. Our extensive experimental results demonstrate that the deep learning model trained on real data through the contextual loss has delivered better visual and quantitative SR performance than those trained on simulated data or through other loss functions. The trained SR model is also proved to generalize well to images of various plankton species or captured by different instruments. The proposed SR technology is anticipated to enhance the existing darkfield plankton imageries and enable the future in situ plankton imaging instruments for better observation capability and hence deepen understanding of the plankton ecology.
更多查看译文
关键词
deep learning model,visual SR performance,plankton species,plankton ecology,underwater plankton cameras compromise,single frame acquisition,larger FOV,real-underwater image dataset,situ plankton im-age super-resolution,high resolution images,image registration algorithmic pipeline,IsPlanktonSR dataset,deep residual network,in situ plankton images,field of view,darkfield plankton imageries,low resolution images,dual-channel darkfield imaging system,live planktonic organisms
AI 理解论文
溯源树
样例
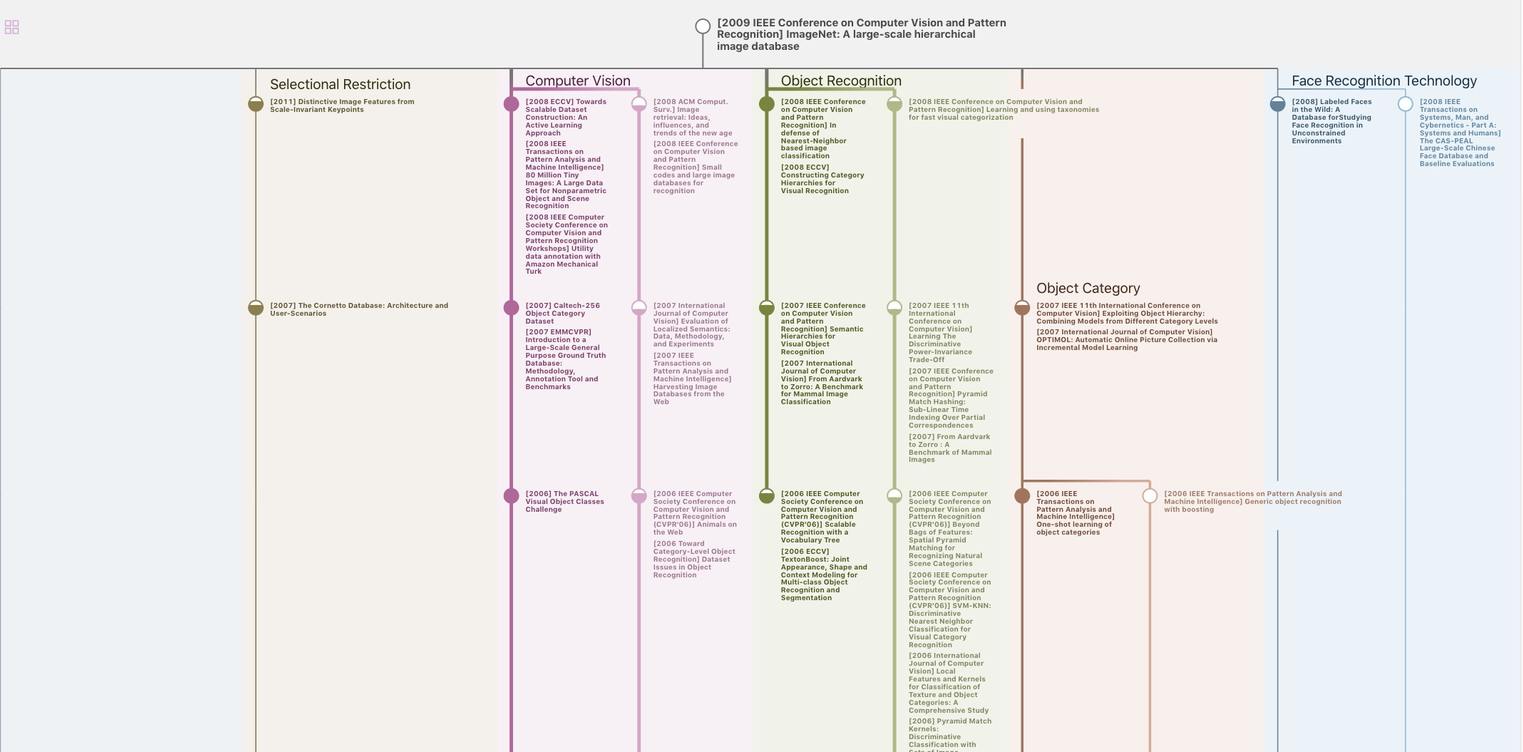
生成溯源树,研究论文发展脉络
Chat Paper
正在生成论文摘要