Multi-modal Variational Faster R-CNN for Improved Visual Object Detection in Manufacturing.
2021 IEEE/CVF INTERNATIONAL CONFERENCE ON COMPUTER VISION WORKSHOPS (ICCVW 2021)(2021)
摘要
Visual object detection is a critical task for a variety of industrial applications, such as robot navigation, quality control and product assembling. Modern industrial environments require AI-based object detection methods that can achieve high accuracy, robustness and generalization. To this end, we propose a novel object detection approach that can process and fuse information from RGB-D images for the accurate detection of industrial objects. The proposed approach utilizes a novel Variational Faster R-CNN algorithm that aims to improve the robustness and generalization ability of the original Faster R-CNN algorithm by employing a VAE encoder-decoder network and a very powerful attention layer. Experimental results on two object detection datasets, namely the well-known RGB-D Washington dataset and the QCONPASS dataset of industrial objects that is first presented in this paper, verify the significant performance improvement achieved when the proposed approach is employed.
更多查看译文
关键词
visual object detection,robot navigation,quality control,modern industrial environments,object detection approach,industrial objects,object detection datasets,RGB-D Washington dataset,multimodal variational faster R-CNN,AI-based object detection methods,RGB-D images,fuse information,VAE encoder-decoder network,powerful attention layer,QCONPASS dataset
AI 理解论文
溯源树
样例
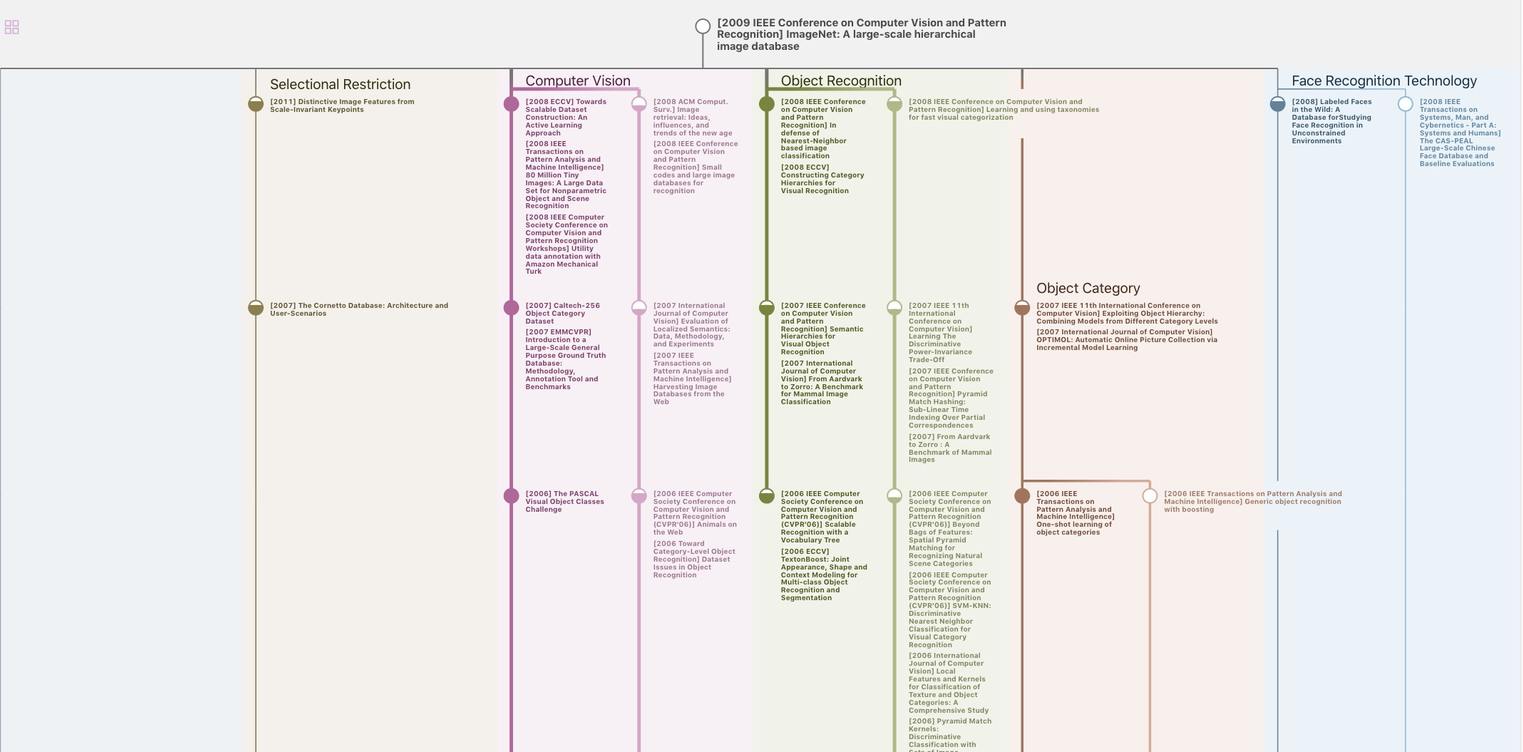
生成溯源树,研究论文发展脉络
Chat Paper
正在生成论文摘要