Extraction of Behavioral Patterns by Recombining Non-Negative Multiple Matrix Factorization and Clustering Results.
GCCE(2021)
摘要
In recent years, the widespread use of IC cards and the development of sensor devices have made it possible to collect and store a wide range of data. Research has been conducted on analyzing human behavior using this data. Non-negative Multiple Matrix Factorization (NMMF) is one of the matrix factorization-based methods to extract frequent patterns from multiple data. Kojima et al. proposed a method for analyzing the relationship between behavioral characteristics and personal attributes using NMMF and decision tree learning on the movement log data of workers. However, there are several problems with this method of interpreting behavior patterns. Therefore, we propose a method that facilitates the interpretation of the behavioral patterns of clusters by creating and visualizing a matrix of extracted features of the patterns.
更多查看译文
关键词
traveling-time patterns,entry and exit data,office workers,clustering,Non-Negative Multiple Matrix Factorization
AI 理解论文
溯源树
样例
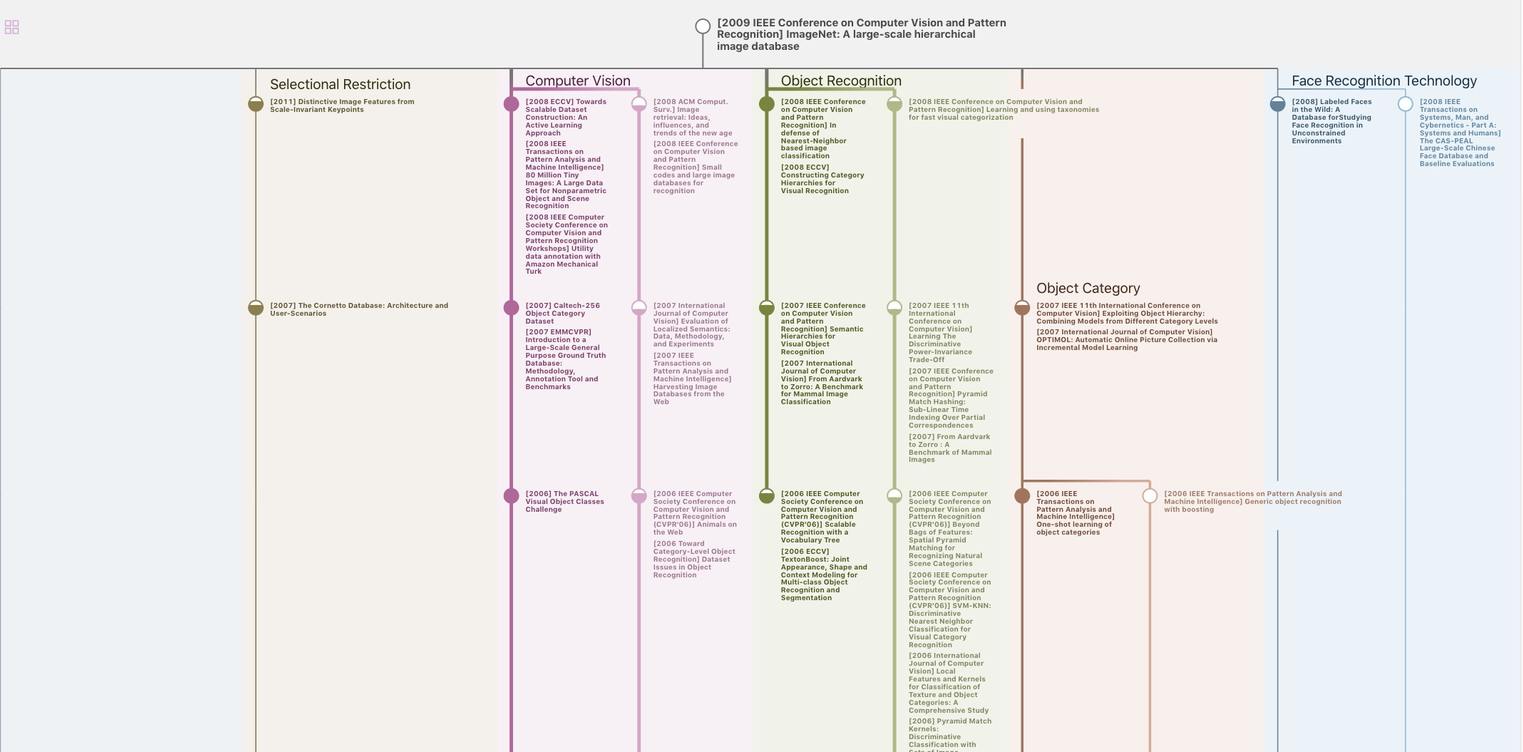
生成溯源树,研究论文发展脉络
Chat Paper
正在生成论文摘要