Long-Term Map Maintenance in Complex Environments.
BRACIS(2021)
摘要
As changes in external environments are inevitable, a lifelong mapping system is desirable for autonomous robots that aim at long-term operation. Capturing external environment changes into internal representations (for example, maps) is crucial for proper behavior and safety, especially in the case of autonomous vehicles. In this work, we propose a new large-scale mapping system for our autonomous vehicle or any other. The new mapping system is based on the Graph SLAM algorithm, with extensions to deal with the calibration of odometry directly in the optimization of the graph and to address map merging for long-term map maintenance. The mapping system can use sensor data from one or more robots to build and merge different types of occupancy grid maps. The system’s performance is evaluated in a series of experiments carried out with data captured in complex real-world scenarios. The experimental results indicate that the new large-scale mapping system can provide high-quality occupancy grid maps for later navigation and localization of autonomous vehicles that use occupancy grid maps.
更多查看译文
关键词
Mapping, SLAM, Map maintenance, Map merging, Autonomous vehicles
AI 理解论文
溯源树
样例
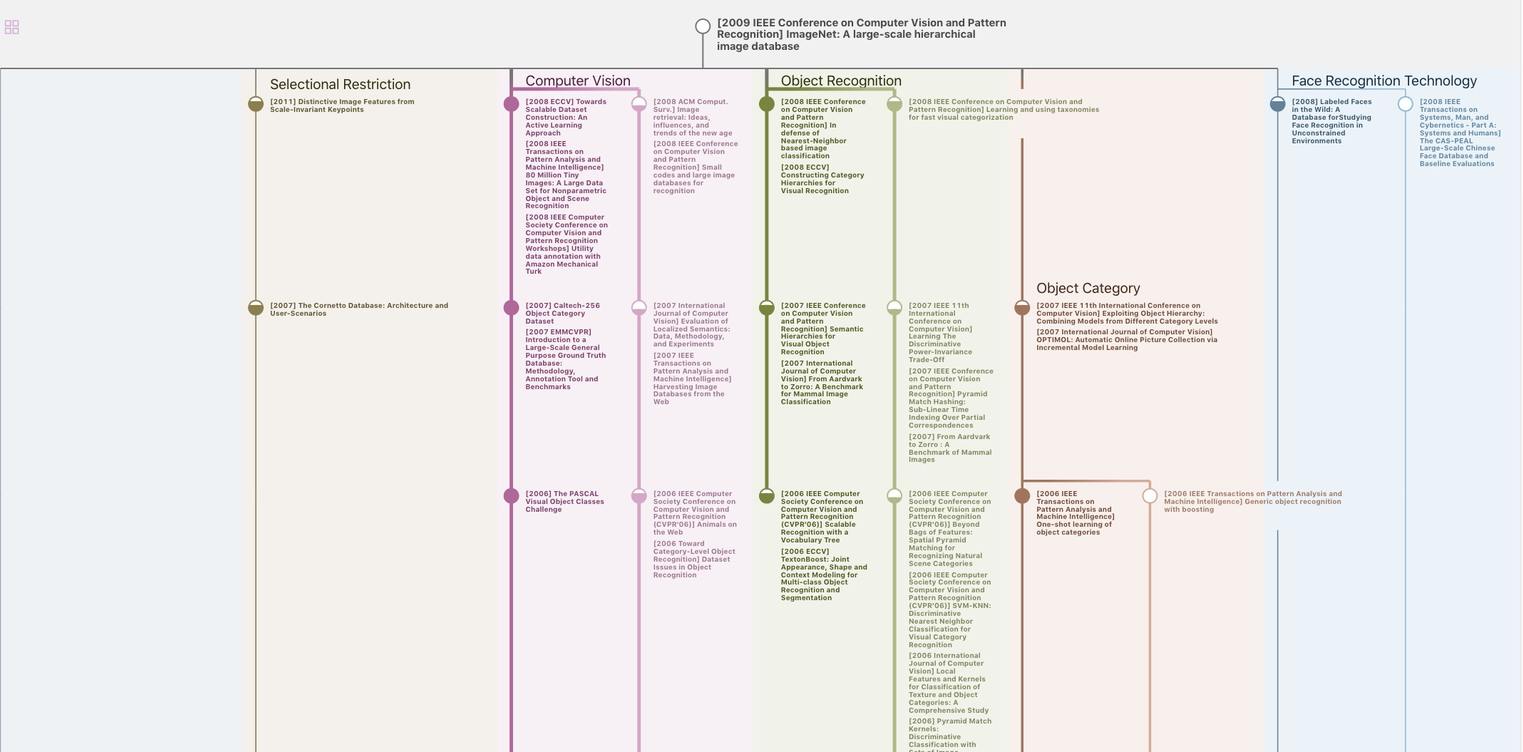
生成溯源树,研究论文发展脉络
Chat Paper
正在生成论文摘要