Visibility Graph Network of Multidimensional Time Series Data for Protein Structure Classification
29TH EUROPEAN SIGNAL PROCESSING CONFERENCE (EUSIPCO 2021)(2021)
摘要
In the last decades, many studies have explored the potential of utilizing complex network approaches to characterize time series generated from dynamical systems. Along these lines, Visibility Graph (VG) and Horizontal Visibility Graph (HVG) networks have contributed to an important yet difficult problem in bioinformatics, the classification of the secondary structure of low-homology proteins. In particular, each protein is presented as a two-dimensional time series that is later transformed, using either VG or HVG, into two independent graphs. However, this is an inefficient way of processing multidimensional time series as it fails to capture the correlation between the two signals while it also increases the time and memory complexity. To address this issue, this work proposes four novel VG and HVG-based frameworks that are able to deal directly with the multidimensional time series. Each of the methods generates a unique graph following a different visibility rule concerning only the relation between pairs of time series intensities of the multidimensional time series. Experimental evaluation on real protein sequences demonstrates the superiority of our best scheme, with respect to both accuracy and computational time, when compared against the state-of-the-art.
更多查看译文
关键词
Visibility Graph, Horizontal Visibility Graph, Multidimensional time series, Nonlinear time series analysis, Secondary structure classification
AI 理解论文
溯源树
样例
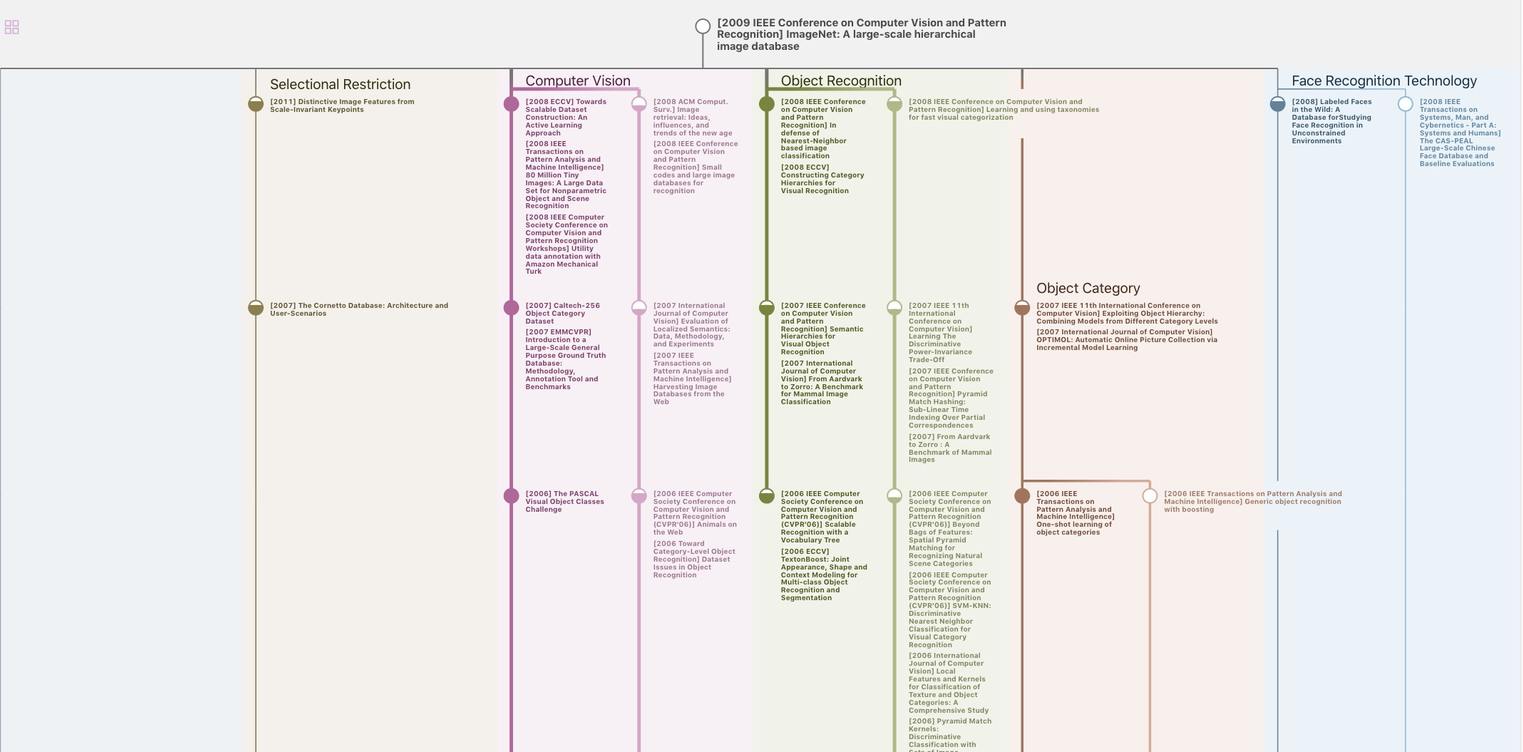
生成溯源树,研究论文发展脉络
Chat Paper
正在生成论文摘要