A Comparative Study of Arousal and Valence Dimensional Variations for Emotion Recognition Using Peripheral Physiological Signals Acquired from Wearable Sensors
2021 43RD ANNUAL INTERNATIONAL CONFERENCE OF THE IEEE ENGINEERING IN MEDICINE & BIOLOGY SOCIETY (EMBC)(2021)
摘要
Wearable sensors have made an impact on healthcare and medicine by enabling out-of-clinic health monitoring and prediction of pathological events. Further advancements made in the analysis of multimodal signals have been in emotion recognition which utilizes peripheral physiological signals captured by sensors in wearable devices. There is no universally accepted emotion model, though multidimensional methods are often used, the most popular of which is the two-dimensional Russell’s model based on arousal and valence. Arousal and valence values are discrete, usually being either binary with low and high labels along each dimension creating four quadrants or 3-valued with low, neutral, and high labels. In day-to-day life, the neutral emotion class is the most dominant leaving emotion datasets with the inherent problem of class imbalance. In this study, we show how the choice of values in the two–dimensional model affects the emotion recognition using multiple machine learning algorithms. Binary classification resulted in an accuracy of 87.2% for arousal and up to 89.5% for valence. Maximal 3-class classification accuracy was 80.9% for arousal and 81.1% for valence. For the joined classification of arousal and valence, the four-quadrant model reached 87.8%, while the nine-class model had an accuracy of 75.8%. This study can be used as a basis for further research into feature extraction for better overall classification performance.
更多查看译文
关键词
emotion recognition,peripheral physiological signals,arousal,valence dimensional variations
AI 理解论文
溯源树
样例
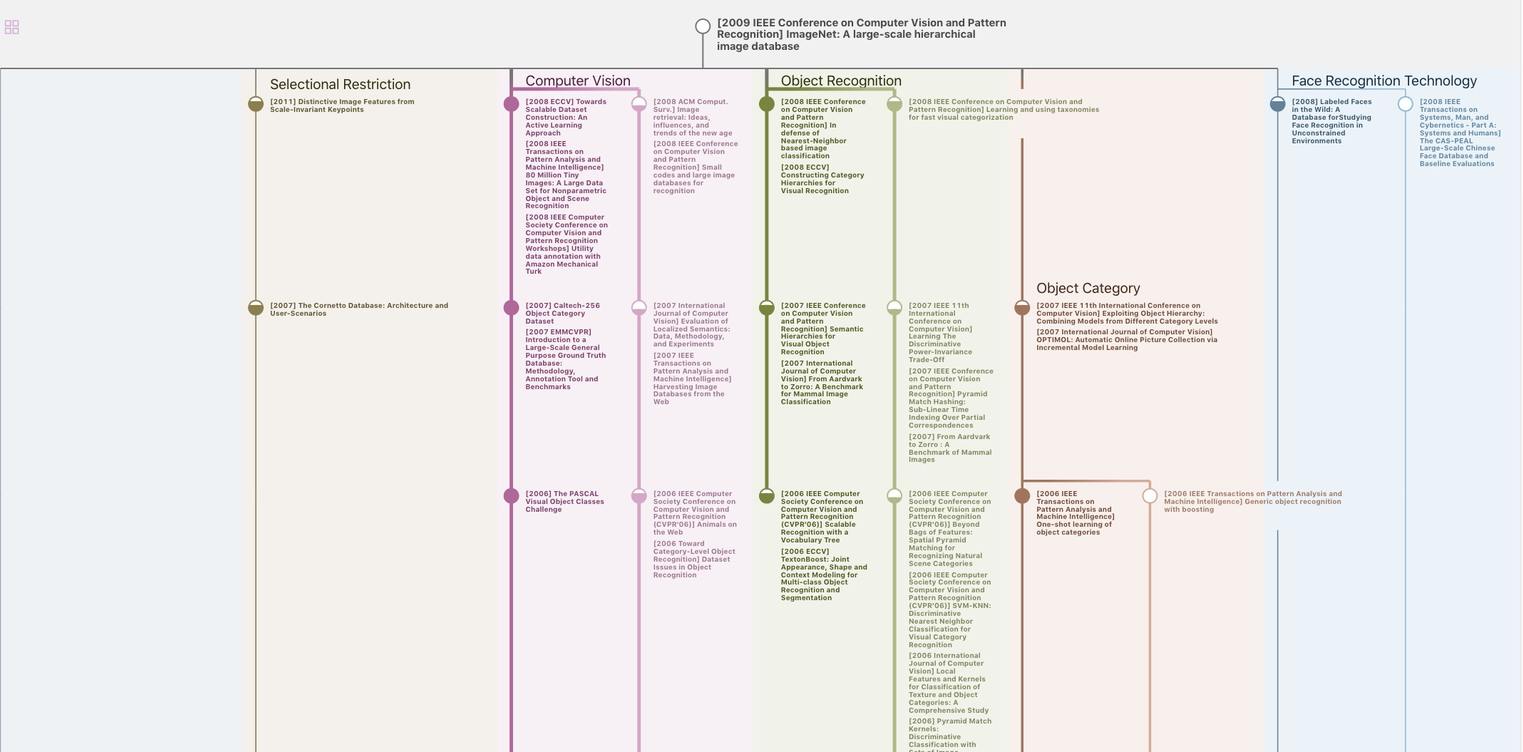
生成溯源树,研究论文发展脉络
Chat Paper
正在生成论文摘要