Explainable Sleep Stage Classification with Multimodal Electrophysiology Time-series
2021 43RD ANNUAL INTERNATIONAL CONFERENCE OF THE IEEE ENGINEERING IN MEDICINE & BIOLOGY SOCIETY (EMBC)(2021)
摘要
Many automated sleep staging studies have used deep learning approaches, and a growing number of them have used multimodal data to improve their classification performance. However, few studies using multimodal data have provided model explainability. Some have used traditional ablation approaches that "zero out" a modality. However, the samples that result from this ablation are unlikely to be found in real electroencephalography (EEG) data, which could adversely affect the importance estimates that result. Here, we train a convolutional neural network for sleep stage classification with EEG, electrooculograms (EOG), and electromyograms (EMG) and propose an ablation approach that replaces each modality with values that approximate the line-related noise commonly found in electrophysiology data. The relative importance that we identify for each modality is consistent with sleep staging guidelines, with EEG being important for most sleep stages and EOG being important for Rapid Eye Movement (REM) and non-REM stages. EMG showed low relative importance across classes. A comparison of our approach with a "zero out" ablation approach indicates that while the importance results are consistent for the most part, our method accentuates the importance of modalities to the model for the classification of some stages like REM (p < 0.05). These results suggest that a careful, domain-specific selection of an ablation approach may provide a clearer indicator of modality importance. Further, this study provides guidance for future research on using explainability methods with multimodal electrophysiology data. Clinical Relevance- While explainability is helpful for clinical machine learning classifiers, it is important to consider how explainability methods interact with clinical data, a domain for which they were not originally designed.
更多查看译文
关键词
Electrooculography,Electrophysiology,Polysomnography,Sleep,Sleep Stages
AI 理解论文
溯源树
样例
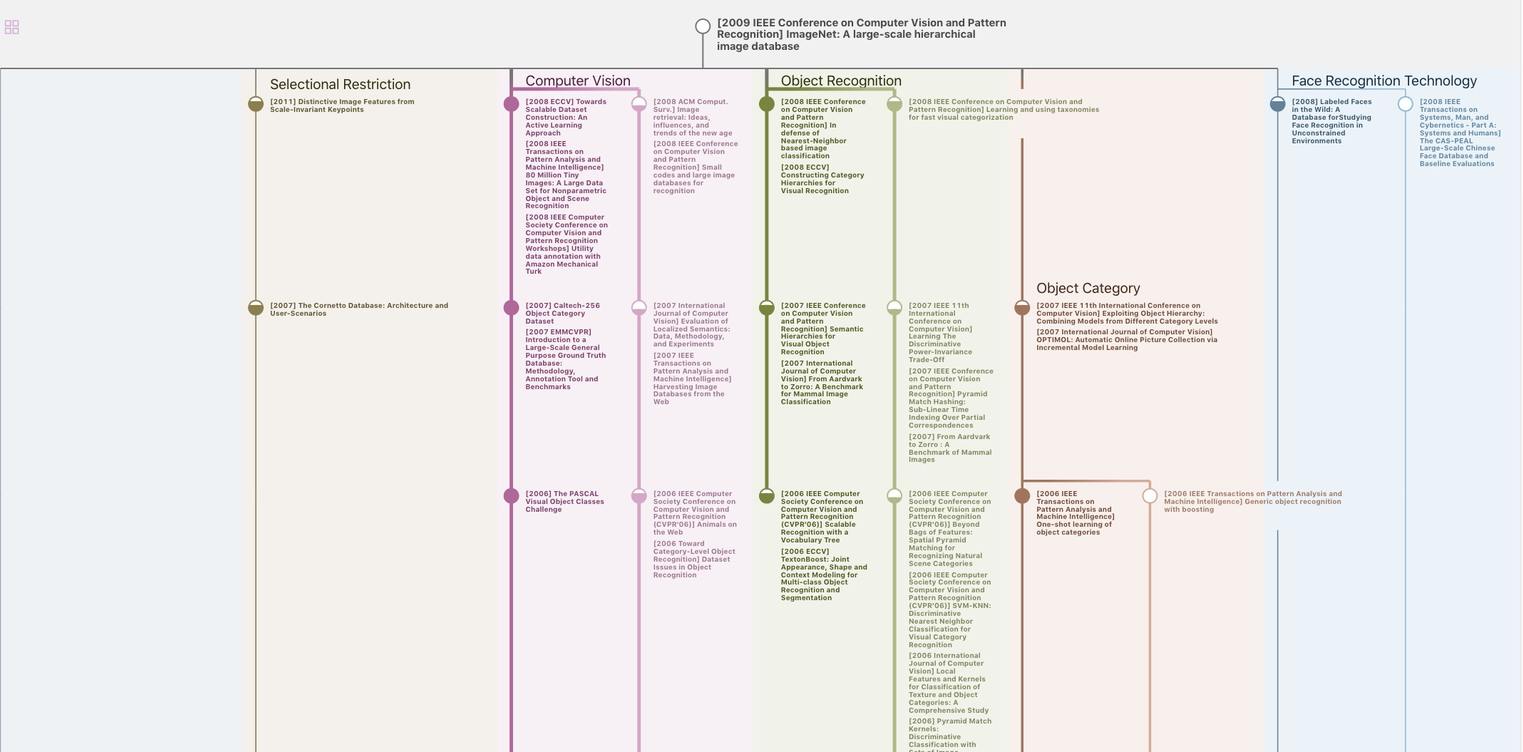
生成溯源树,研究论文发展脉络
Chat Paper
正在生成论文摘要