A Novel Machine-Learning Approach for Evaluating Rebounds-Associated Environmental Footprint of Households and Application to Cooperative Housing.
Journal of environmental management(2022)
摘要
Multiple environmental policies aim to increase resource efficiency and reduce consumption of goods and services with high environmental impact. This may lead to cost-savings and, consequently, additional consumption with environmental impacts (rebound effects). In this study, a supervised machine-learning model (i.e. an application of random forest regression) is developed to quantify consumption rebound effects. In contrast to previous approaches, it is a versatile method, which allows to estimate any income-related rebound at household level considering specific household properties and the entire profile of consumption. Socio-economic properties (e.g. income, age group) of the households are used as the independent properties for the regressor to detect the dependent consumption expenses of the households. Thus, this method can be used as a bottom-up study for understanding rebounds and developing targeted measures to prevent or reduce rebound effects. To illustrate the application of the method, it is applied to the case of cooperative housing in Switzerland. In addition to environmental goals, the cooperative aims to provide affordable housing, and the reduced rent increases the disposable income of tenants. The results show that households tend to spend the 'extra' income on housing (e.g. for larger apartments) and travel. For the former, the cooperative already has a policy in place regulating the apartment area permitted per person, which delimits induced environmental impacts. For the latter, households with lower income particularly spend their extra-money on purchase and operation of vehicles, while high-erincome groups rather spend it on recreation and package holidays. Travel, housing, clothing and personal care products have highest emissions per Swiss Franc (similar to 0.3-0.6 kg CO2-eq/CHF). Thus, it is recommended to provide incentives for shifting these expenses to other consumption, to avoid jeopardizing environmental goals. The method was also used for a range of other applications e.g. rebounds due to energy-efficient devices to illustrate its versatility.
更多查看译文
关键词
Machine learning,Regression,Rebounds,Housing sector,Environmental footprint
AI 理解论文
溯源树
样例
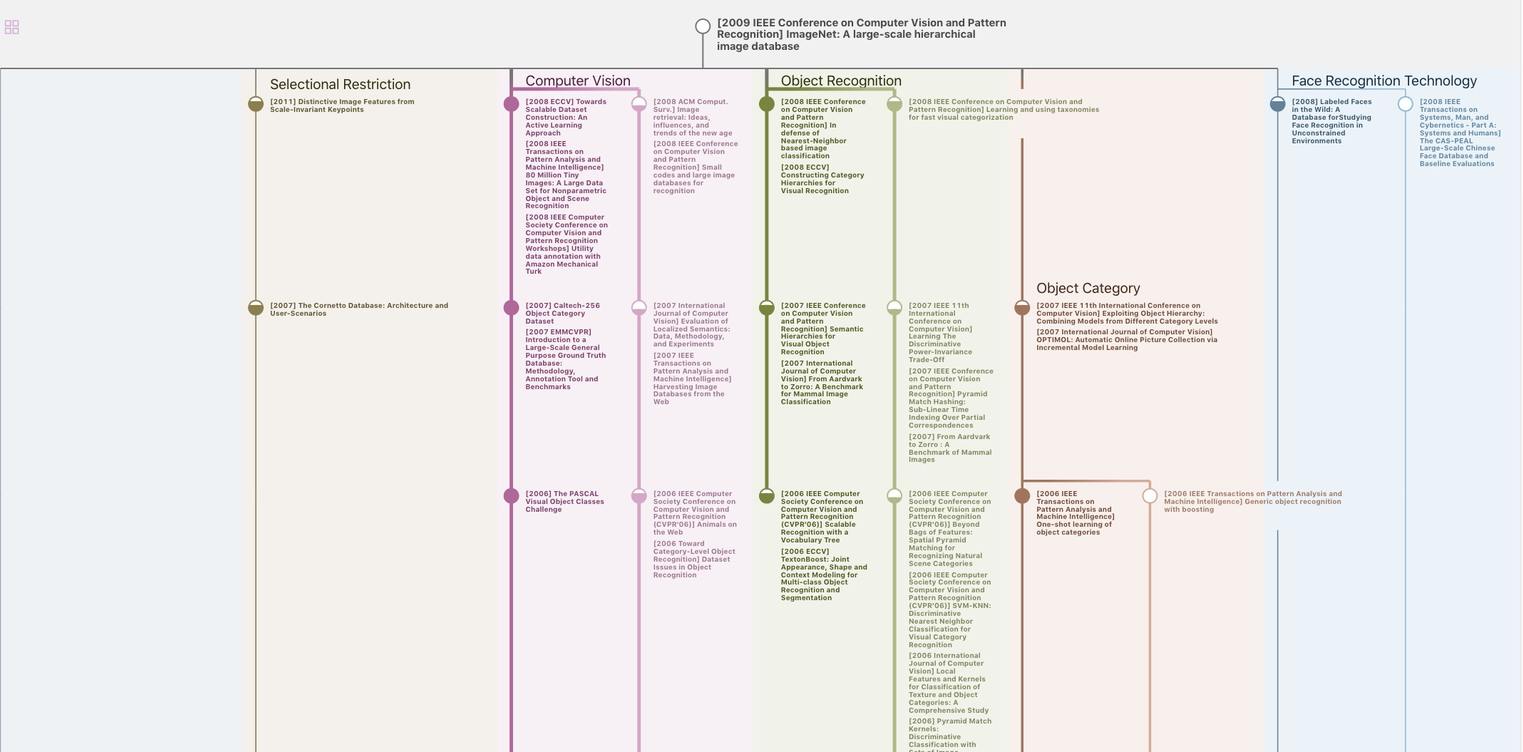
生成溯源树,研究论文发展脉络
Chat Paper
正在生成论文摘要