An Auxiliary Tasks Based Framework for Automated Medical Skill Assessment with Limited Data
2021 43RD ANNUAL INTERNATIONAL CONFERENCE OF THE IEEE ENGINEERING IN MEDICINE & BIOLOGY SOCIETY (EMBC)(2021)
摘要
Automated medical skill assessment facilitates medical education by merging varying clinical experiences across instructors for standardizing medical training. However, medical datasets for training such automated assessment rarely have satisfactory sizes due to the cost of data collection, safety concerns and privacy restrictions. Current medical training relies on evaluation rubrics that usually include multiple auxiliary labels to support the overall evaluation from varying aspects of the procedure. In this paper, we explore machine learning algorithms to design a generalizable auxiliary task-based framework for medical skill assessment to address training automated systems with limited data. Our framework exhaustively mines valid auxiliary information in the evaluation rubric to pre-train the feature extractor before training the skill assessment classifier. Notably, a new regression-based multitask weighting method is the key to pre-train a meaningful feature representation comprehensively, ensuring the evaluation rubric is well imitated in the final model. The overall evaluation task can be fine-tuned based on the pre-trained rubric-based feature representation. Our experimental results on two medical skill datasets show that our work can significantly improve performance, achieving 85.9% and 97.4% accuracy in the intubation dataset and surgical skill dataset, respectively.
更多查看译文
关键词
Algorithms,Machine Learning
AI 理解论文
溯源树
样例
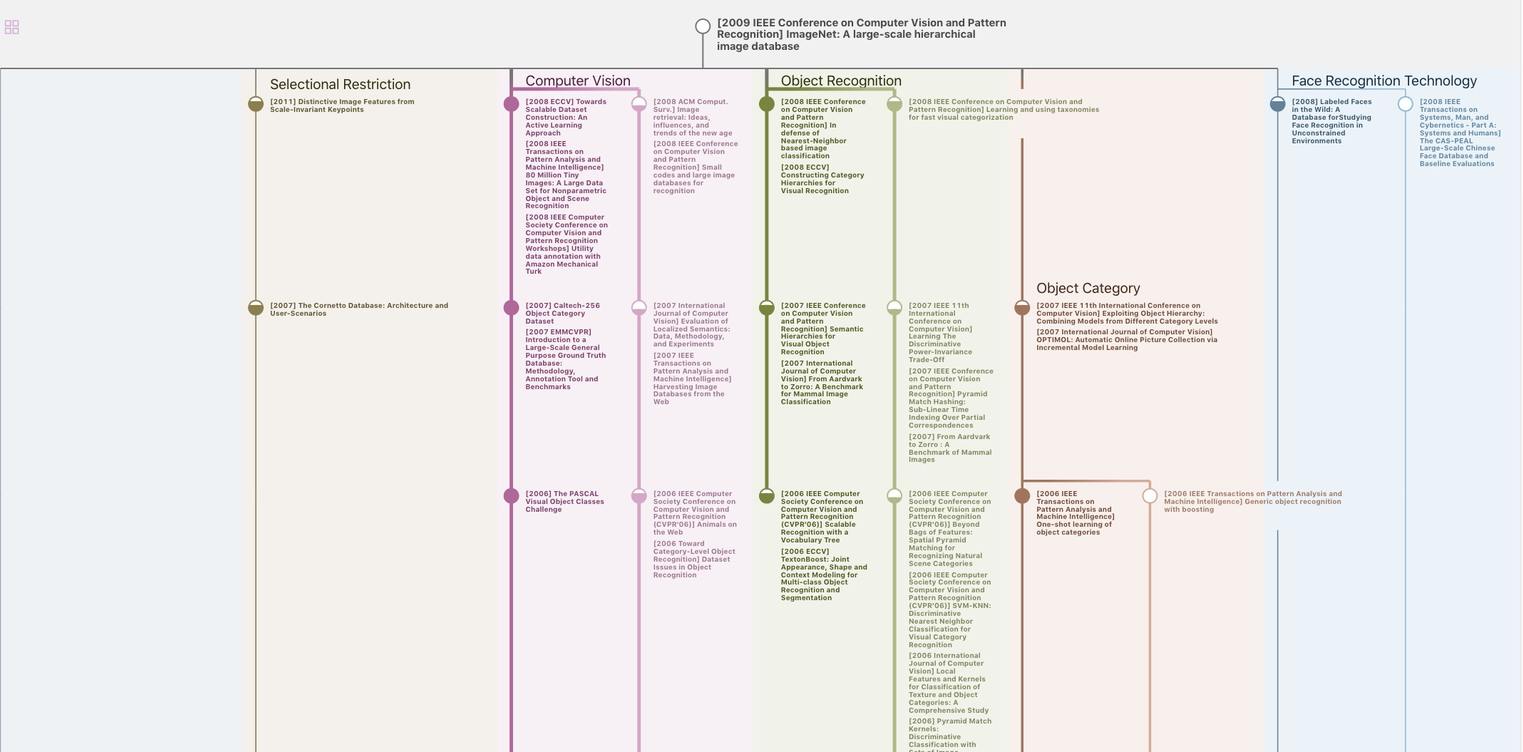
生成溯源树,研究论文发展脉络
Chat Paper
正在生成论文摘要