Cardinality and Short-Term Memory Concepts based Novel Feature Extraction for Myoelectric Pattern Recognition
2021 43RD ANNUAL INTERNATIONAL CONFERENCE OF THE IEEE ENGINEERING IN MEDICINE & BIOLOGY SOCIETY (EMBC)(2021)
摘要
The quality of the extracted traditional hand-crafted Electromyogram (EMG) features has been recently identified in the literature as a limiting factor prohibiting the translation from laboratory to clinical settings. To address this limitation, a shift of focus from traditional feature extraction methods to deep learning models was witnessed, as the latter can learn the best feature representation for the task at hand. However, while deep learning models achieve promising results based on raw EMG data, their clinical implementation is often challenged due to their significantly high computational costs (significantly large number of generated models' parameters and a huge amount of data needed for training). This paper is focused on combining the simplicity and low computational characteristics of traditional feature extraction with the memory concepts from Long Short-Term Memory (LSTM) models to efficiently extract the spatial-temporal dynamics of the EMG signals. The novelty of the proposed method can be summarized in a) the memory concept leveraged from deep learning structures, capturing short-term temporal dependencies of the EMG signals, b) the use of cardinality to generate logical combinations of spatially distinct EMG signals and as a feature extraction method and 3) low computational costs and the enhanced classification performance. The performance of the proposed method is validated using three EMG databases collected with 1) laboratory hardware (9 transradial amputees and 17 intact-limbed), and 2) wearables (22 intact-limed using two wearable consumer armbands). In comparison to several other well-known methods from the literature, the proposed method shows significantly enhanced myoelectric pattern recognition performance, with accuracies reaching up to 99%.
更多查看译文
关键词
feature extraction,novel feature extraction,recognition,short-term
AI 理解论文
溯源树
样例
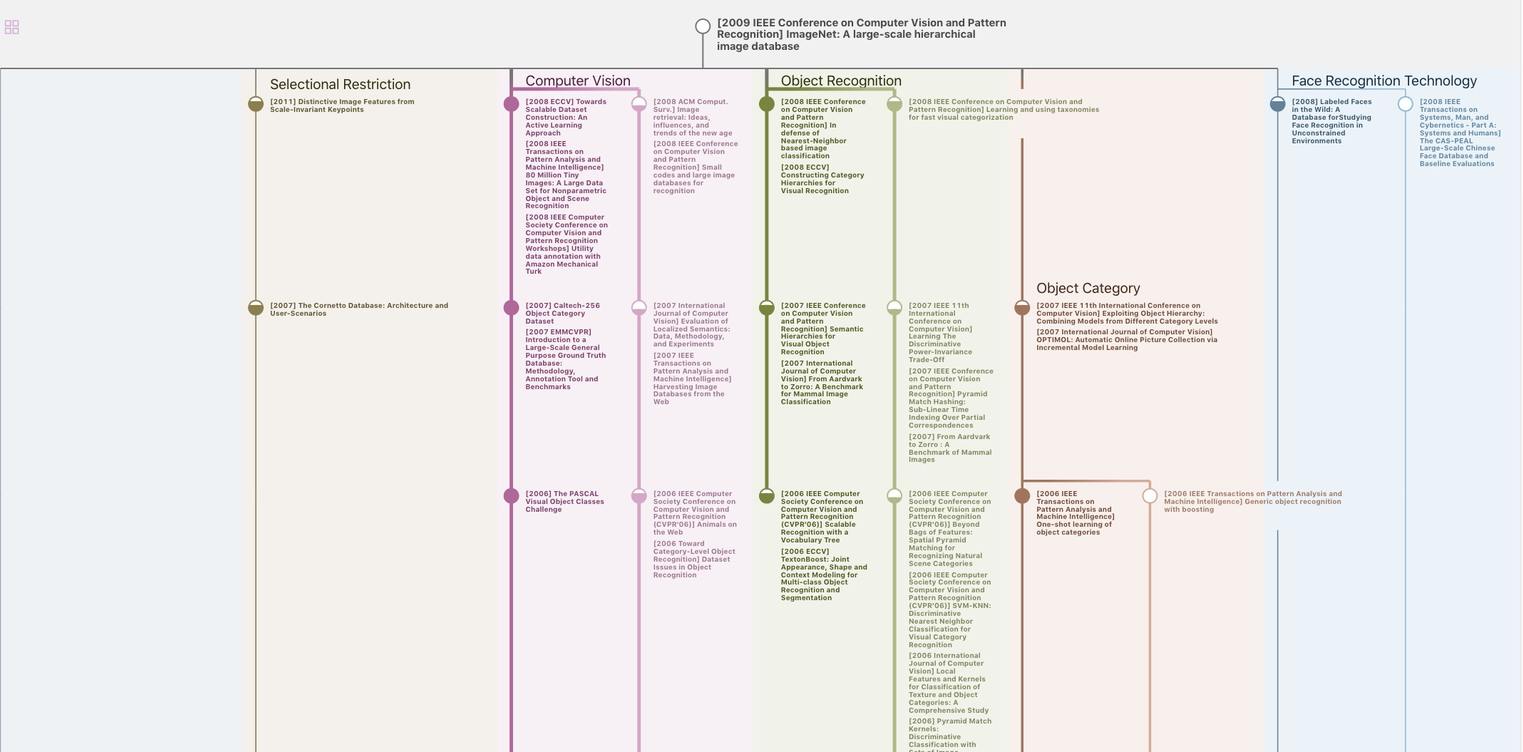
生成溯源树,研究论文发展脉络
Chat Paper
正在生成论文摘要