A Novel Deep Learning Approach for Tracking Regions of Interest in Ultrasound Images
2021 43RD ANNUAL INTERNATIONAL CONFERENCE OF THE IEEE ENGINEERING IN MEDICINE & BIOLOGY SOCIETY (EMBC)(2021)
摘要
Due to their great success in learning a universal object similarity metric, Siamese Trackers have been adopted for motion tracking a Region of Interest (ROI) in Ultrasound (US) image sequences. However, these Fully Convolutional Siamese networks (SiamFC) offer no online adaptation of the network and fail to take cues from the input sequence. The more recent Correlation Filter Networks (CFNet) solve this problem by learning the reference template online using a Correlation Filter layer. In this work, we use the CFNet as our backbone model and propose an advanced tracking algorithm (SeqCFNet) for tracking an ROI in US sequences by constructing a sequential cascade of two identical CFNet. The cascade with CFNet is novel and offers practical benefits in tracking accuracy. Our method is evaluated on 10 different sequences of a Carotid Artery (CA) dataset to track the transverse section of the carotid artery. Results show that Seq-CFNet obtains better Root Mean Square Error (RMSE) values than the baseline CFNet as well as SiamFC, without significantly compromising the speed.
更多查看译文
关键词
Speckle Tracking, Correlation Filter Network, Siamese Network, Convolutional Neural Network, Cascaded Network
AI 理解论文
溯源树
样例
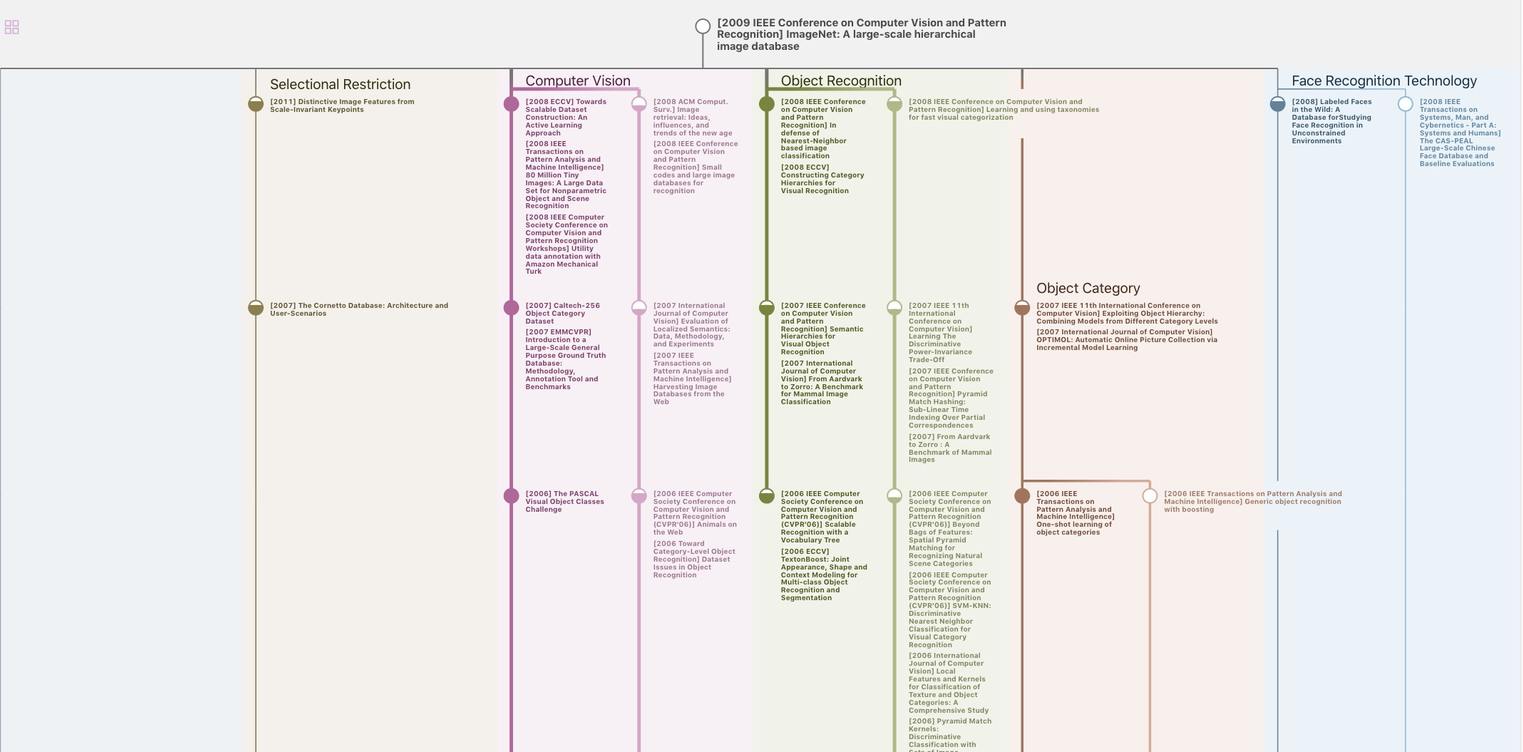
生成溯源树,研究论文发展脉络
Chat Paper
正在生成论文摘要