Deep Learning-Based Segmentation and Uncertainty Assessment for Automated Analysis of Myocardial Perfusion MRI Datasets Using Patch-Level Training and Advanced Data Augmentation
2021 43RD ANNUAL INTERNATIONAL CONFERENCE OF THE IEEE ENGINEERING IN MEDICINE & BIOLOGY SOCIETY (EMBC)(2021)
摘要
In this work, we develop a patch-level training approach and a task-driven intensity-based augmentation method for deep-learning-based segmentation of motion-corrected perfusion cardiac magnetic resonance imaging (MRI) datasets. Further, the proposed method generates an image-based uncertainty map thanks to a novel spatial sliding-window approach used during patch-level training, hence allowing for uncertainty quantification. Using the quantified uncertainty, we detect the out-of-distribution test data instances so that the end-user can be alerted that the test data is not suitable for the trained network. This feature has the potential to enable a more reliable integration of the proposed deep learning-based framework into clinical practice. We test our approach on external MRI data acquired using a different acquisition protocol to demonstrate the robustness of our performance to variations in pulse-sequence parameters. The presented results further demonstrate that our deep-learning image segmentation approach trained with the proposed data-augmentation technique incorporating spatiotemporal (2D+time) patches is superior to the state-of-the-art 2D approach in terms of generalization performance.
更多查看译文
关键词
Deep Learning,Heart,Magnetic Resonance Imaging,Perfusion,Uncertainty
AI 理解论文
溯源树
样例
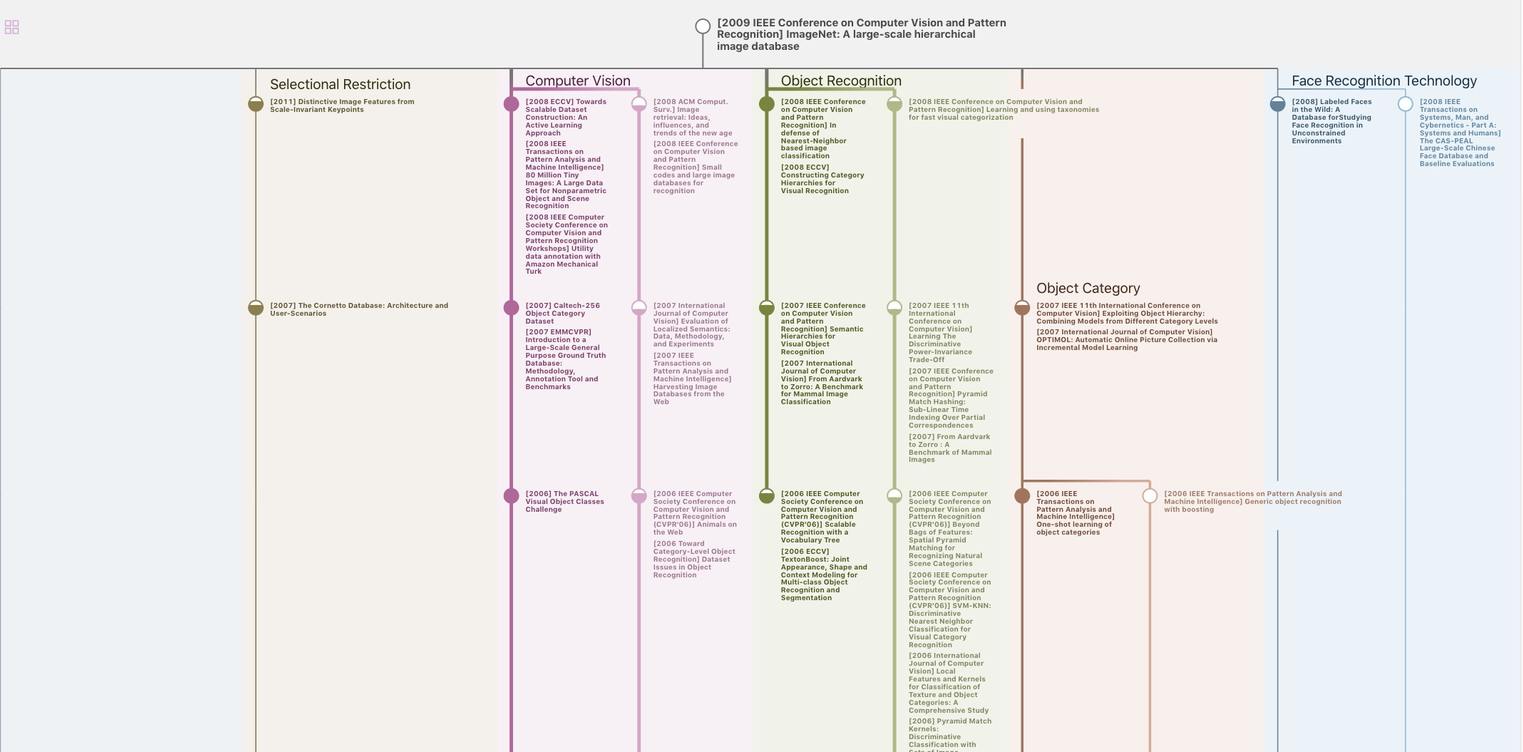
生成溯源树,研究论文发展脉络
Chat Paper
正在生成论文摘要