Ultra-low-power Physical Activity Classifier for Wearables: From Generic MCUs to ASICs
2021 43RD ANNUAL INTERNATIONAL CONFERENCE OF THE IEEE ENGINEERING IN MEDICINE & BIOLOGY SOCIETY (EMBC)(2021)
摘要
In the era of Internet of Things (IoT), an increasing amount of sensors is being integrated into intelligent wearable devices. These sensors have the potential to produce a large quantity of physiological data streams to be analyzed in order to produce meaningful and actionable information. An important part of this processing is usually located in the device itself and takes the form of embedded algorithms which are executed into the onboard microcontroller (MCU). As data processing algorithms have become more complex due to, in part, the disruption of machine learning, they are taking an increasing part of MCU time becoming one of the main driving factors in the energy budget of the overall embedded system. We propose to integrate such algorithms into dedicated low-power circuits making the power consumption of the processing part negligible to the overall system. We provide the results of several implementations of a pre-trained physical activity classifier used in smartwatches and wristbands. The algorithm combines signal processing for feature extraction and machine learning in the form of decision trees for physical activity classification. We show how an in-silicon implementation decreases up to 0.1 mu W the power consumption compared to 73 mu W on a general-purpose ARM's Cortex-M0 MCU.
更多查看译文
关键词
Algorithms,Exercise,Machine Learning,Signal Processing, Computer-Assisted,Wearable Electronic Devices
AI 理解论文
溯源树
样例
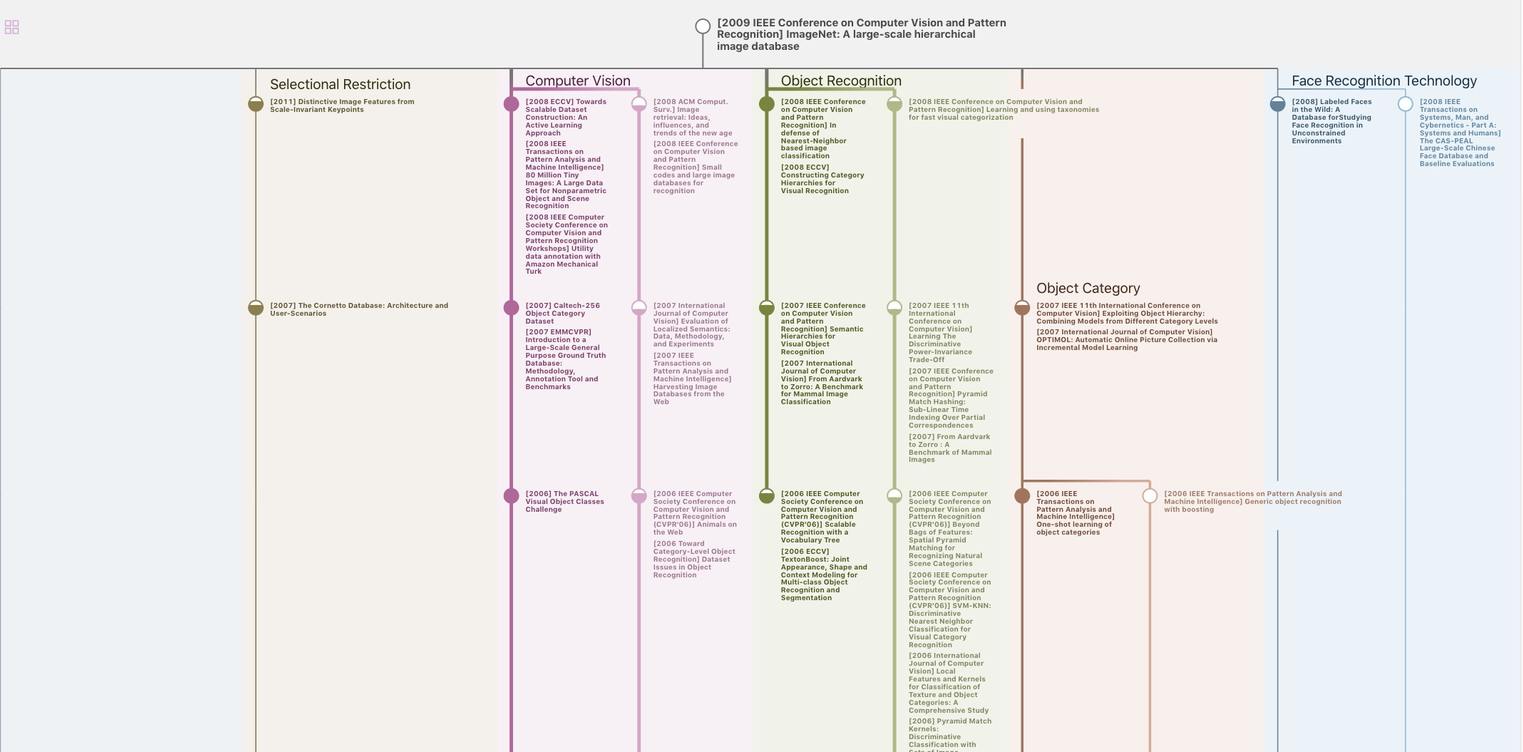
生成溯源树,研究论文发展脉络
Chat Paper
正在生成论文摘要