Equivariant Quantum Graph Circuits.
International Conference on Machine Learning(2023)
摘要
We investigate quantum circuits for graph representation learning, and propose equivariant quantum graph circuits (EQGCs), as a class of parameterized quantum circuits with strong relational inductive bias for learning over graph-structured data. Conceptually, EQGCs serve as a unifying framework for quantum graph representation learning, allowing us to define several interesting subclasses which subsume existing proposals. In terms of the representation power, we prove that the studied subclasses of EQGCs are universal approximators for functions over the bounded graph domain. This theoretical perspective on quantum graph machine learning methods opens many directions for further work, and could lead to models with capabilities beyond those of classical approaches. We empirically verify the expressive power of EQGCs through a dedicated experiment on synthetic data, and additionally observe that the performance of EQGCs scales well with the depth of the model and does not suffer from barren plateu issues.
更多查看译文
关键词
Quantum machine learning,Graph representation learning,Machine learning theory,Model expressivity
AI 理解论文
溯源树
样例
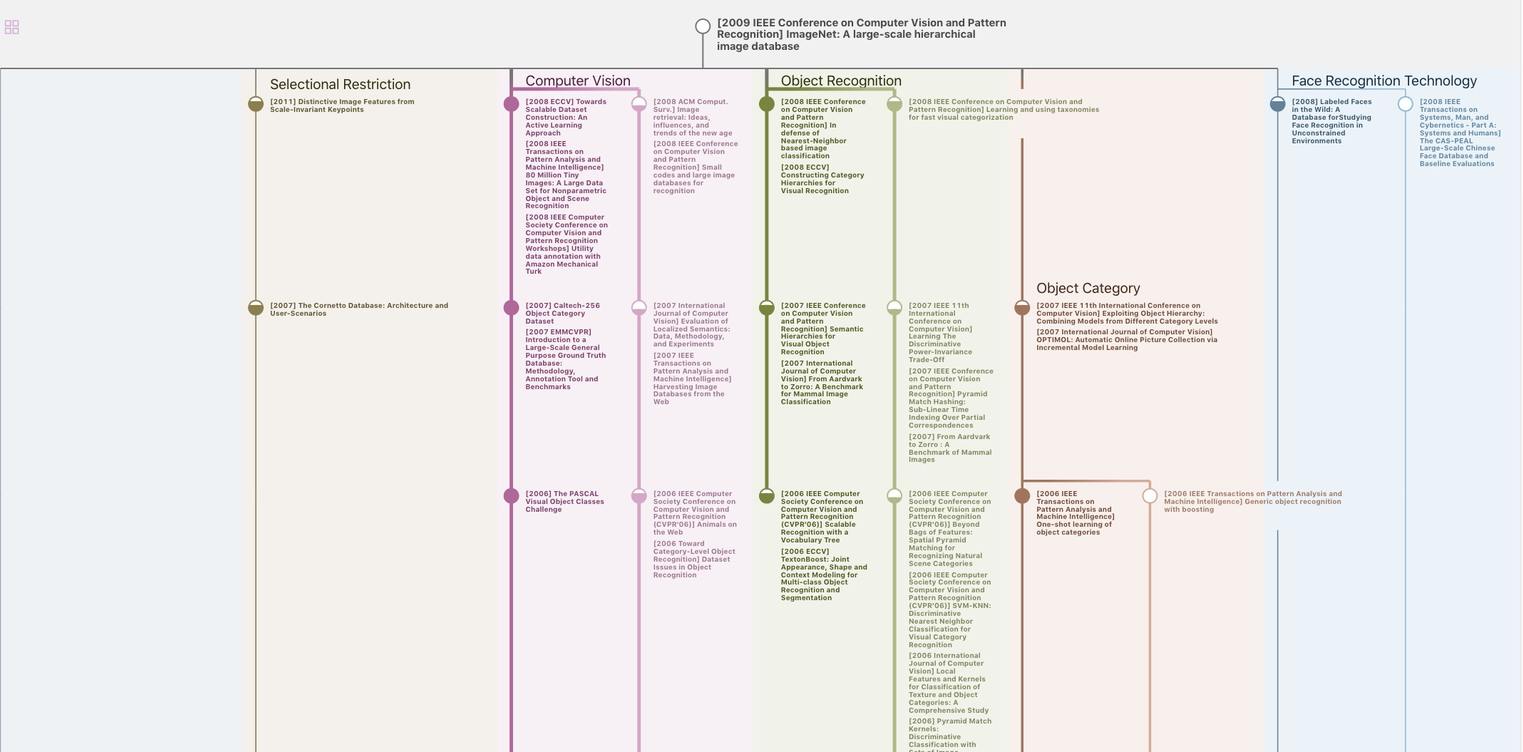
生成溯源树,研究论文发展脉络
Chat Paper
正在生成论文摘要