Hyperdimensional Feature Fusion for Out-of-Distribution Detection
WACV(2023)
摘要
We introduce powerful ideas from Hyperdimensional Computing into the challenging field of Out-of-Distribution (OOD) detection. In contrast to most existing works that perform OOD detection based on only a single layer of a neural network, we use similarity-preserving semi-orthogonal projection matrices to project the feature maps from multiple layers into a common vector space. By repeatedly applying the bundling operation., we create expressive classspecific descriptor vectors for all in-distribution classes. At test time, a simple and efficient cosine similarity calculation between descriptor vectors consistently identifies OOD samples with competitive performance to the current stateof-the-art whilst being significantly faster. We show that our method is orthogonal to recent state-of-the-art OOD detectors and can be combined with them to further improve upon the performance.
更多查看译文
关键词
detection,feature,fusion,out-of-distribution
AI 理解论文
溯源树
样例
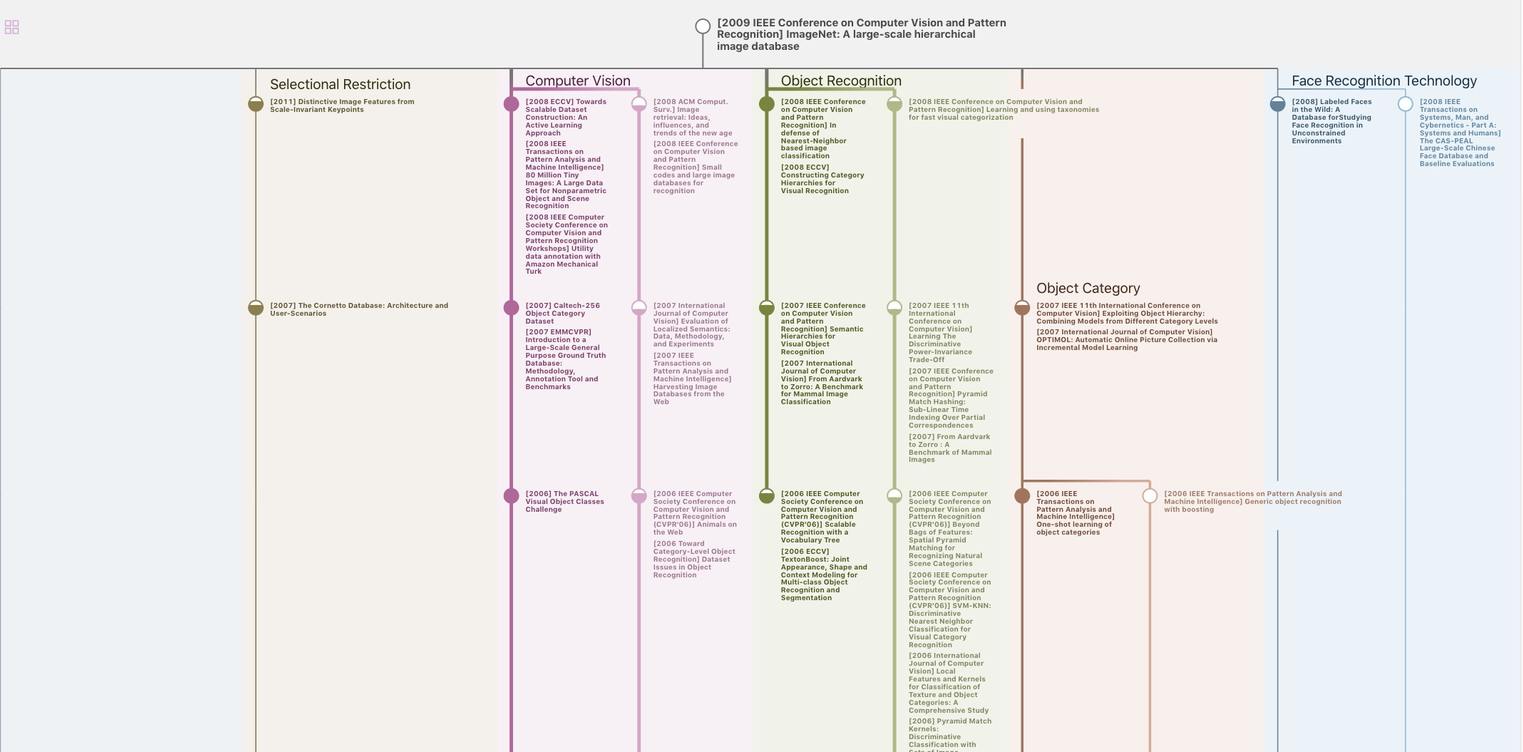
生成溯源树,研究论文发展脉络
Chat Paper
正在生成论文摘要