Transversal GRAND for Network Coded Data
2022 IEEE International Symposium on Information Theory (ISIT)(2022)
摘要
This paper considers a transmitter, which uses random linear coding (RLC) to encode data packets. The generated coded packets are broadcast to one or more receivers. A receiver can recover the data packets if it gathers a sufficient number of coded packets. We assume that the receiver does not abandon its efforts to recover the data packets if RLC decoding has been unsuccessful; instead, it employs syndrome decoding in an effort to repair erroneously received coded packets before it attempts RLC decoding again. A key assumption of most decoding techniques, including syndrome decoding, is that errors are independently and identically distributed within the received coded packets. Motivated by the ‘guessing random additive noise decoding’ (GRAND) framework, we develop transversal GRAND: an algorithm that exploits statistical dependence in the occurrence of errors, complements RLC decoding and achieves a gain over syndrome decoding, in terms of the probability that the receiver will recover the original data packets.
更多查看译文
关键词
Network coding,fountain coding,random linear coding,GRAND,syndrome decoding,error burst
AI 理解论文
溯源树
样例
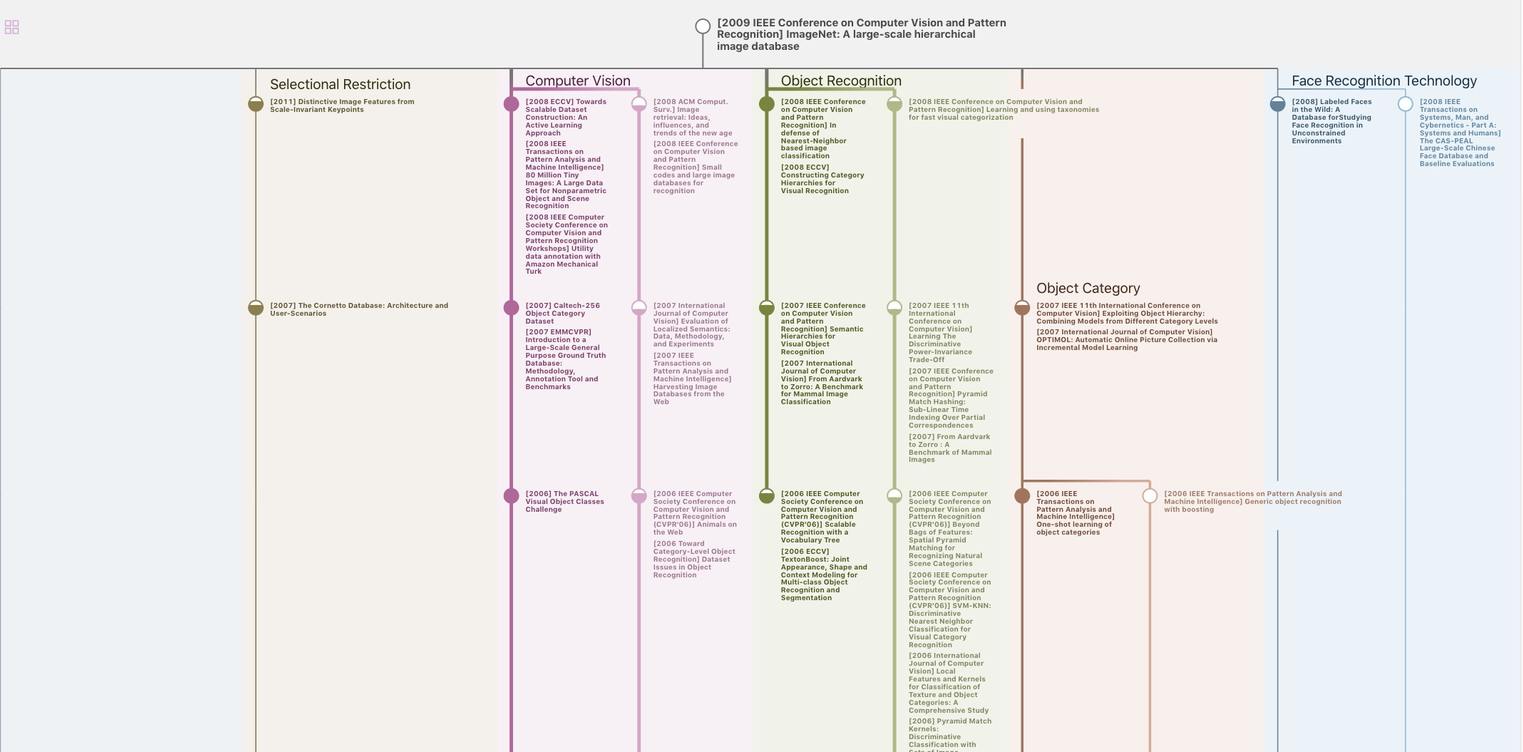
生成溯源树,研究论文发展脉络
Chat Paper
正在生成论文摘要