Towards More Efficient Insertion Transformer with Fractional Positional Encoding
arxiv(2023)
摘要
Auto-regressive neural sequence models have been shown to be effective across text generation tasks. However, their left-to-right decoding order prevents generation from being parallelized. Insertion Transformer (Stern et al., 2019) is an attractive alternative that allows outputting multiple tokens in a single generation step. Nevertheless, due to the incompatibility between absolute positional encoding and insertion-based generation schemes, it needs to refresh the encoding of every token in the generated partial hypothesis at each step, which could be costly. We design a novel reusable positional encoding scheme for Insertion Transformers called Fractional Positional Encoding (FPE), which allows reusing representations calculated in previous steps. Empirical studies on various text generation tasks demonstrate the effectiveness of FPE, which leads to floating-point operation reduction and latency improvements on batched decoding.
更多查看译文
关键词
more efficient insertion transformer,fractional positional
AI 理解论文
溯源树
样例
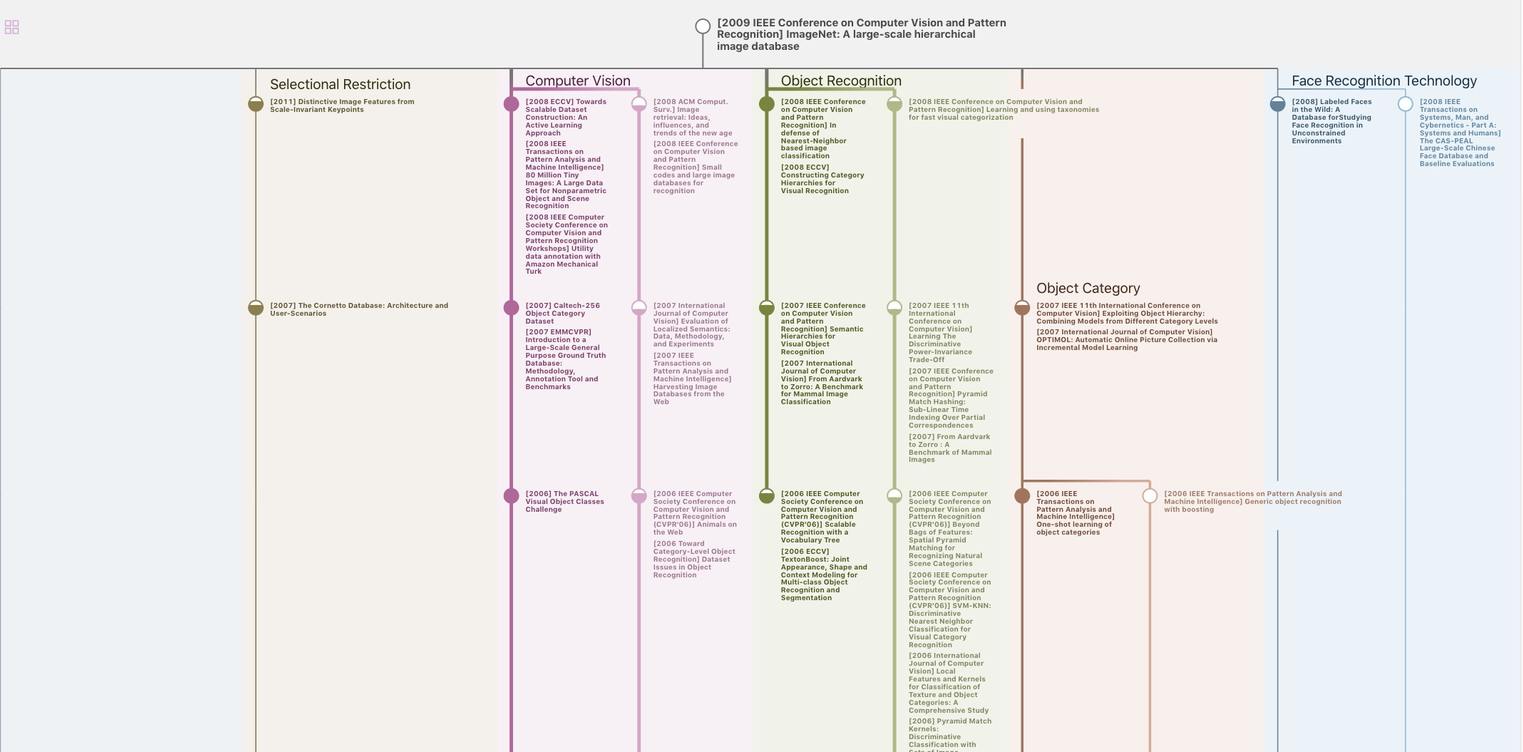
生成溯源树,研究论文发展脉络
Chat Paper
正在生成论文摘要