Recalibrating probabilistic forecasts of epidemics.
PLoS computational biology(2022)
摘要
Distributional forecasts are important for a wide variety of applications, including forecasting epidemics. Often, forecasts are miscalibrated, or unreliable in assigning uncertainty to future events. We present a recalibration method that can be applied to a black-box forecaster given retrospective forecasts and observations, as well as an extension to make this method more effective in recalibrating epidemic forecasts. This method is guaranteed to improve calibration and log score performance when trained and measured in-sample. We also prove that the increase in expected log score of a recalibrated forecaster is equal to the entropy of the PIT distribution. We apply this recalibration method to the 27 influenza forecasters in the FluSight Network and show that recalibration reliably improves forecast accuracy and calibration. This method, available on Github, is effective, robust, and easy to use as a post-processing tool to improve epidemic forecasts.
更多查看译文
关键词
probabilistic forecasts,epidemics
AI 理解论文
溯源树
样例
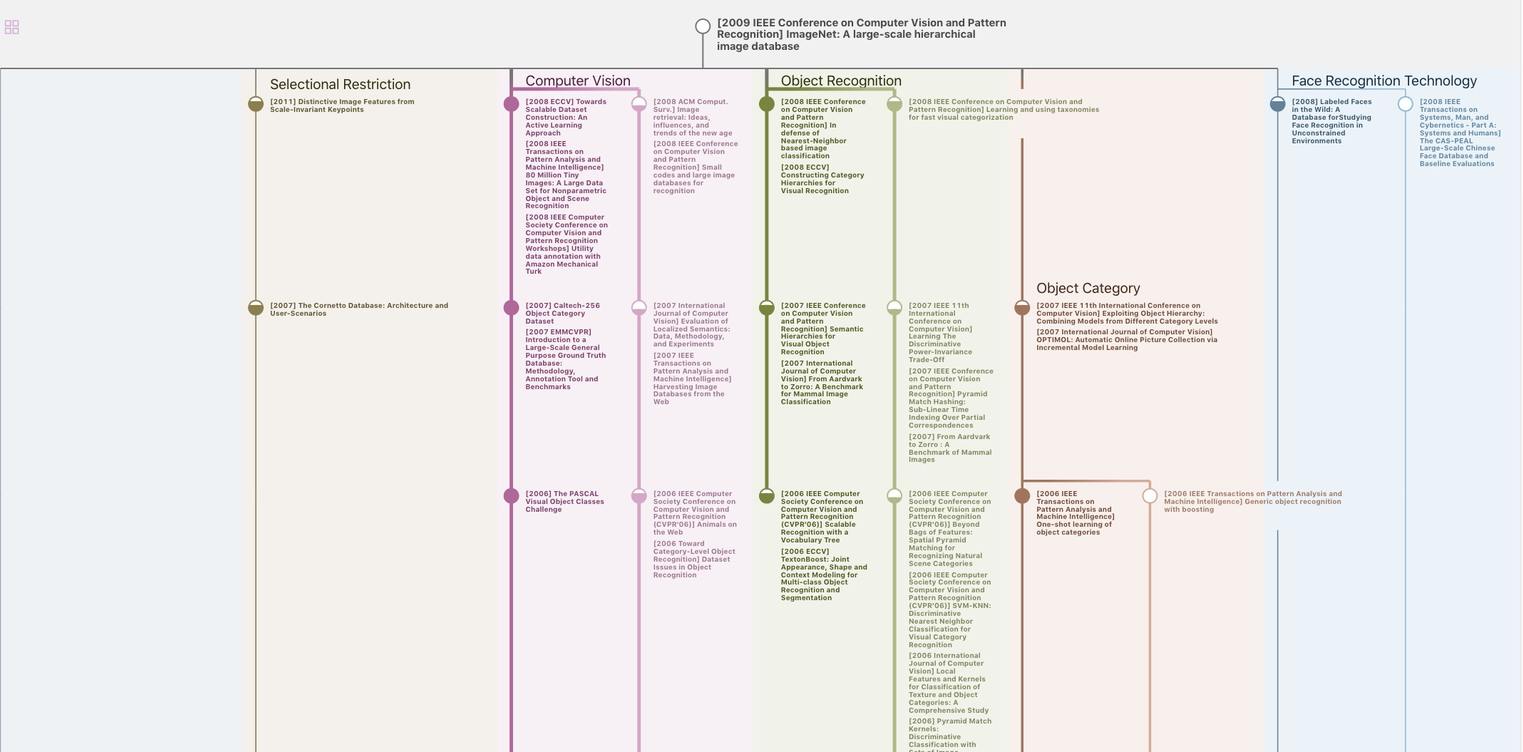
生成溯源树,研究论文发展脉络
Chat Paper
正在生成论文摘要