Interpolated Joint Space Adversarial Training for Robust and Generalizable Defenses
arxiv(2023)
摘要
Adversarial training (AT) is considered to be one of the most reliable defenses against adversarial attacks. However, models trained with AT sacrifice standard accuracy and do not generalize well to unseen attacks. Recent works show generalization improvement with adversarial samples under unseen threat models such as on-manifold threat model or neural perceptual threat model. However, the former requires exact manifold information while the latter requires algorithm relaxation. Motivated by these considerations, we propose a novel threat model called Joint Space Threat Model (JSTM), which exploits the underlying manifold information with Normalizing Flow, ensuring that the exact manifold assumption holds. Under JSTM, we develop novel adversarial attacks and defenses. Specifically, we propose the Robust Mixup strategy in which we maximize the adversity of the interpolated images and gain robustness and prevent overfitting. Our experiments show that Interpolated Joint Space Adversarial Training (IJSAT) achieves good performance in standard accuracy, robustness, and generalization. IJSAT is also flexible and can be used as a data augmentation method to improve standard accuracy and combined with many existing AT approaches to improve robustness. We demonstrate the effectiveness of our approach on three benchmark datasets, CIFAR-10/100, OM-ImageNet and CIFAR-10-C.
更多查看译文
关键词
Adversarial defense,adversarial robustness,generative models,image classification
AI 理解论文
溯源树
样例
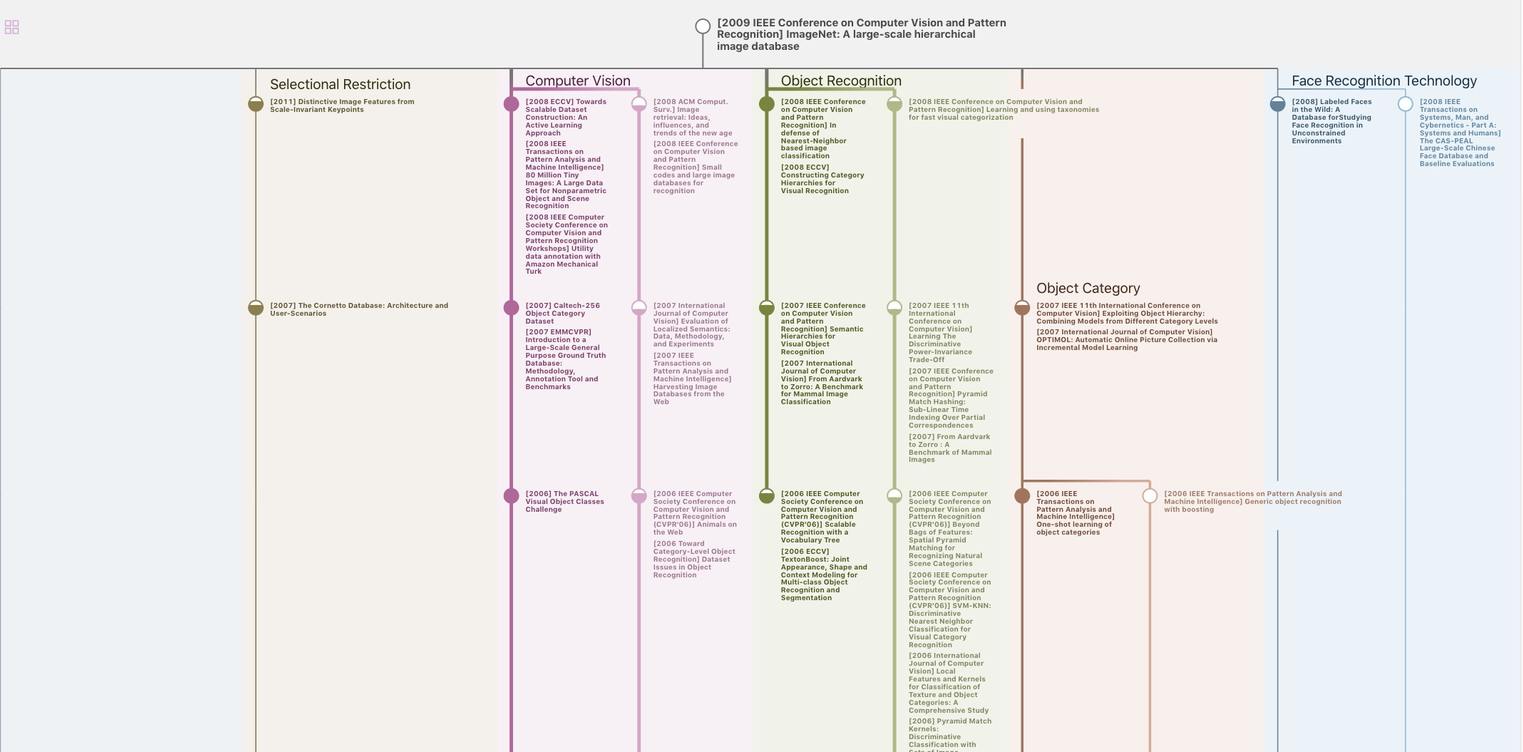
生成溯源树,研究论文发展脉络
Chat Paper
正在生成论文摘要