Testing the robustness of simulation-based gravitational-wave population inference
arxiv(2023)
摘要
Gravitational-wave population studies have become more important in gravitational-wave astronomy because of the rapid growth of the observed catalog. In recent studies, emulators based on different machine learning techniques are used to emulate the outcomes of the population synthesis simulation with fast speed. In this study, we benchmark the performance of two emulators that learn the truncated power-law phenomenological model by using Gaussian process regression and normalizing flows techniques to see which one is a more capable likelihood emulator in the population inference. We benchmark the characteristic of the emulators by comparing their performance in the population inference to the phenomenological model using mock and real observation data. Our results suggest that the normalizing flows emulator can recover the posterior distribution by using the phenomenological model in the population inference with up to 300 mock injections. The normalizing flows emulator also underestimates the uncertainty for some posterior distributions in the population inference on real observation data. On the other hand, the Gaussian process regression emulator has poor performance on the same task and can only be used effectively in low-dimension cases.
更多查看译文
关键词
robustness,simulation-based,gravitational-wave
AI 理解论文
溯源树
样例
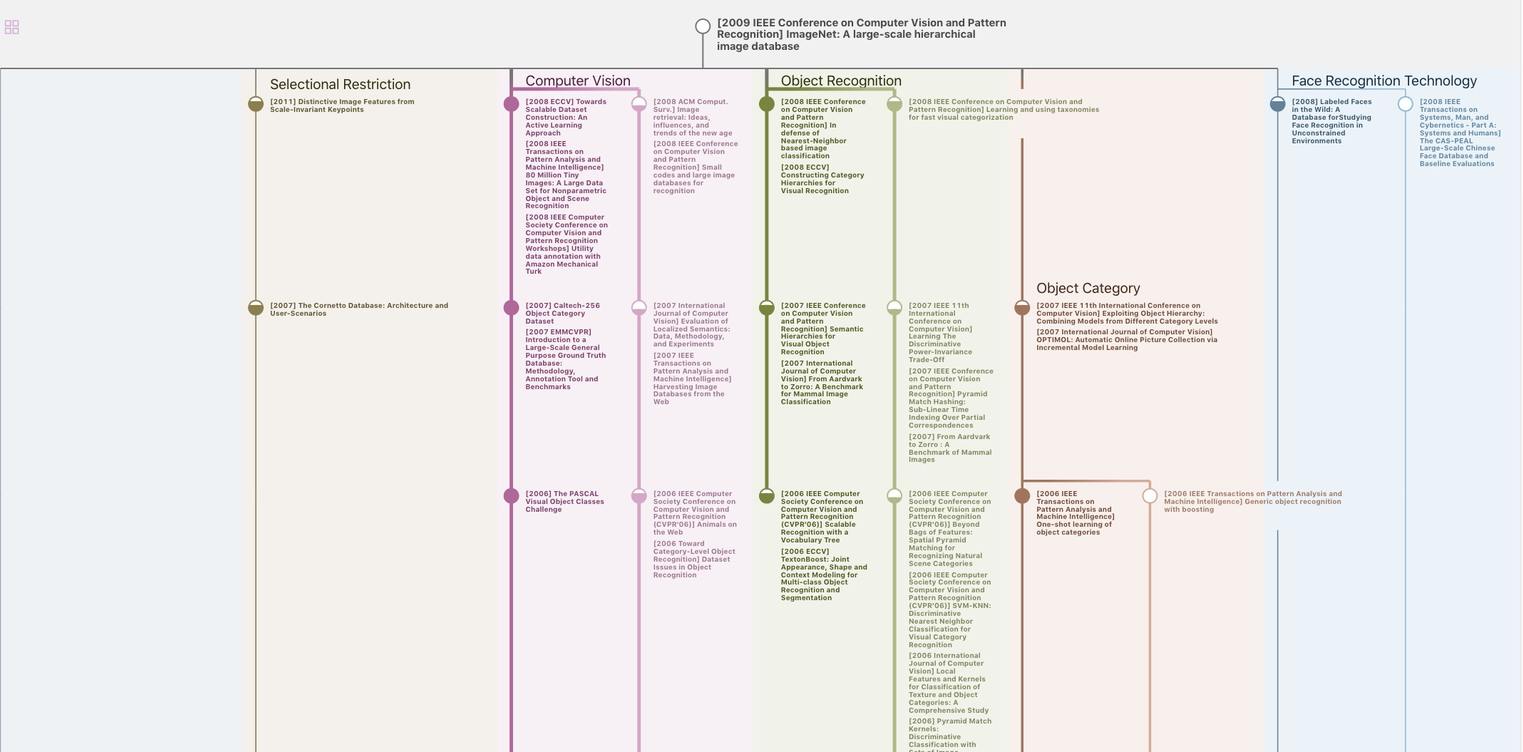
生成溯源树,研究论文发展脉络
Chat Paper
正在生成论文摘要