Long-tail Recognition via Compositional Knowledge Transfer.
IEEE Conference on Computer Vision and Pattern Recognition(2022)
摘要
In this work, we introduce a novel strategy for long-tail recognition that addresses the tail classes' few-shot problem via training-free knowledge transfer. Our objective is to transfer knowledge acquired from information-rich common classes to semantically similar, and yet data-hungry, rare classes in order to obtain stronger tail class representations. We leverage the fact that class prototypes and learned cosine classifiers provide two different, complementary representations of class cluster centres in feature space, and use an attention mechanism to select and recompose learned classifier features from common classes to obtain higher quality rare class representations. Our knowledge transfer process is training free, reducing overfitting risks, and can afford continual extension of classifiers to new classes. Experiments show that our approach can achieve significant performance boosts on rare classes while maintaining robust common class performance, outperforming directly comparable state-of-the-art models.
更多查看译文
关键词
recognition,knowledge,long-tail
AI 理解论文
溯源树
样例
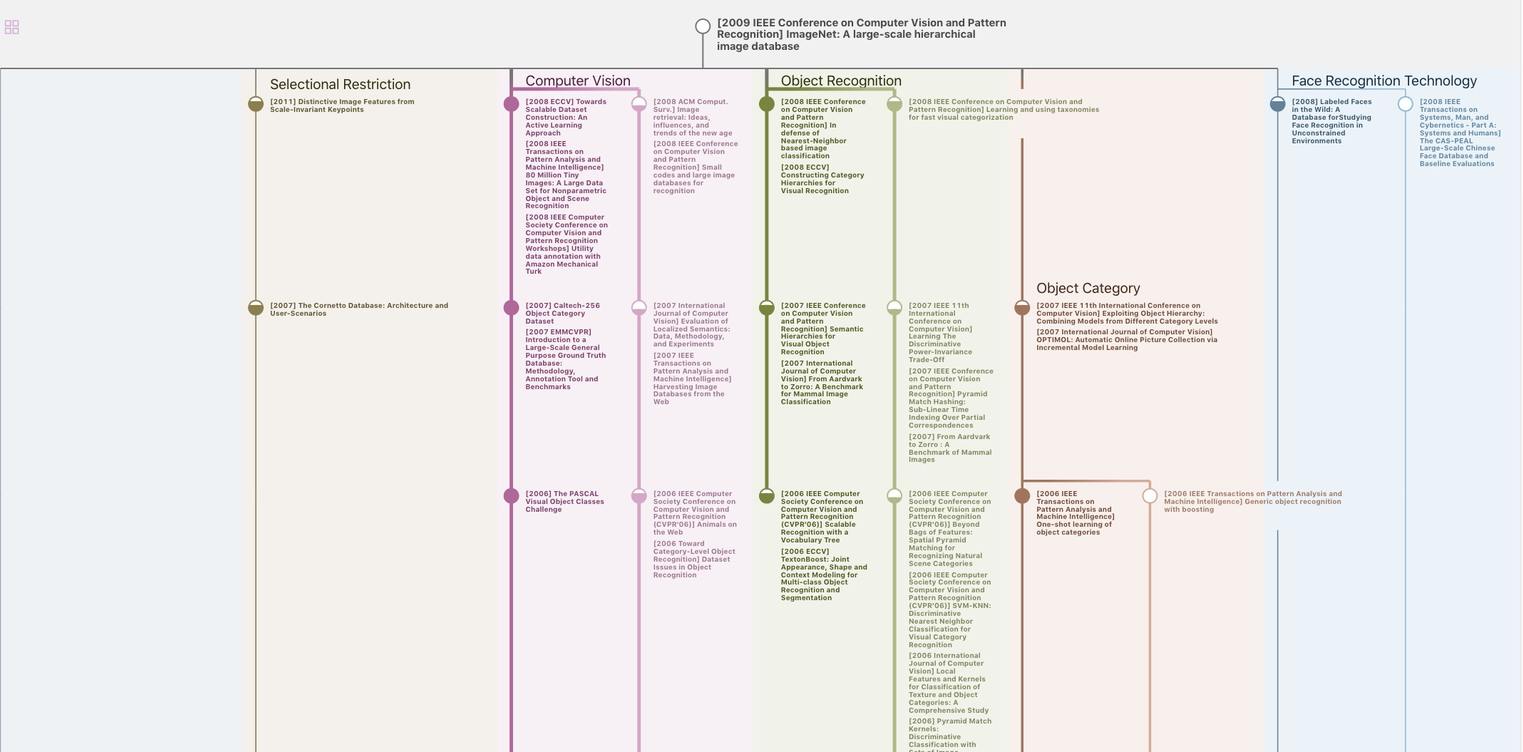
生成溯源树,研究论文发展脉络
Chat Paper
正在生成论文摘要