ATTENTIVE CONTEXTUAL CARRYOVER FOR MULTI-TURN END-TO-END SPOKEN LANGUAGE UNDERSTANDING
2021 IEEE AUTOMATIC SPEECH RECOGNITION AND UNDERSTANDING WORKSHOP (ASRU)(2021)
摘要
Recent years have seen significant advances in end-to-end (E2E) spoken language understanding (SLU) systems, which directly predict intents and slots from spoken audio. While dialogue history has been exploited to improve conventional text-based natural language understanding systems, current E2E SLU approaches have not yet incorporated such critical contextual signals in multi-turn and task-oriented dialogues. In this work, we propose a contextual E2E SLU model architecture that uses a multi-head attention mechanism over encoded previous utterances and dialogue acts (actions taken by the voice assistant) of a multi-turn dialogue. We detail alternative methods to integrate these contexts into the state-of-the-art recurrent and transformer-based models. When applied to a large dc-identified dataset of utterances collected by a voice assistant, our method reduces average word and semantic error rates by 10.8% and 12.6%, respectively. We also present results on a publicly available dataset and show that our method significantly improves performance over a noncontextual baseline.
更多查看译文
关键词
Spoken language understanding, multi-turn, attention, contextual, RNN/Transformer-Transducer
AI 理解论文
溯源树
样例
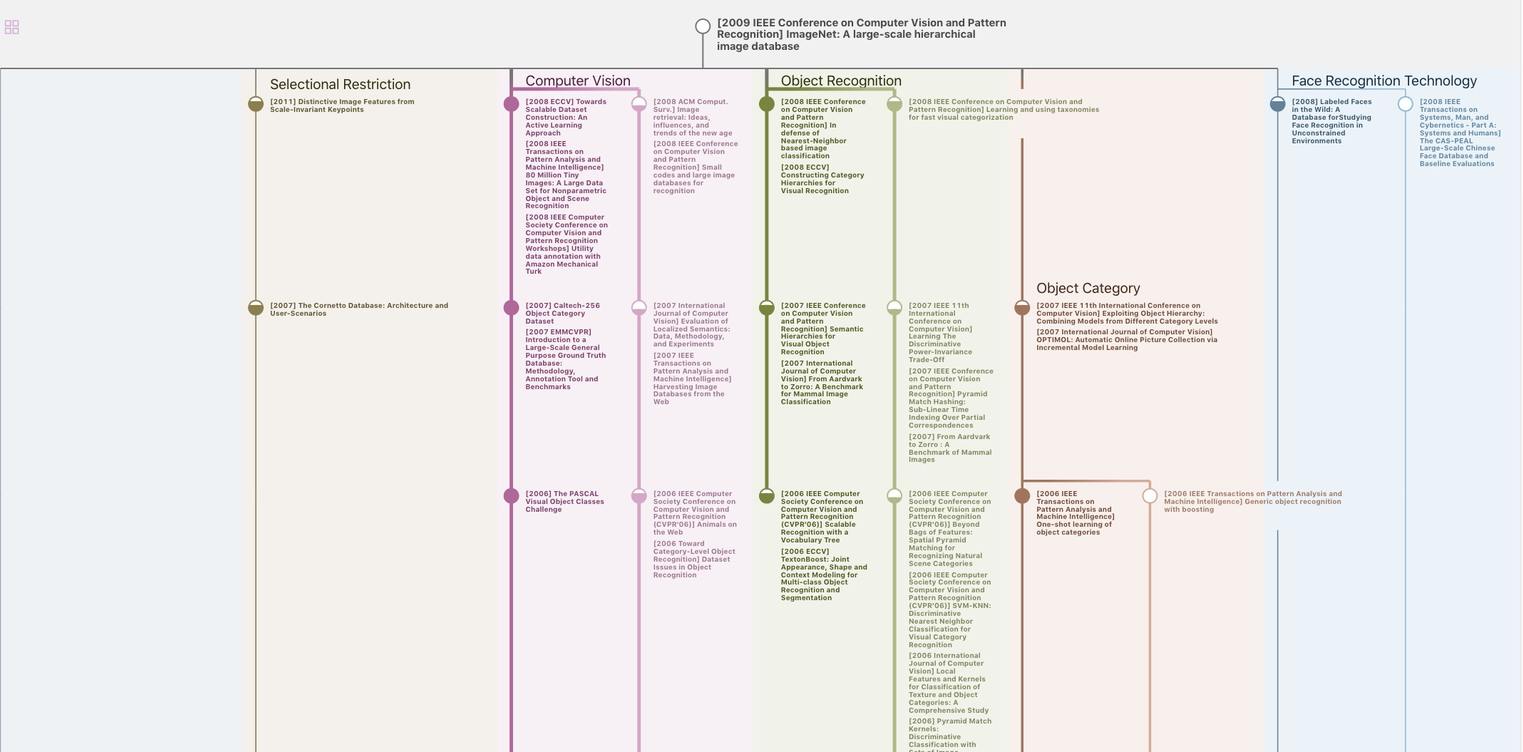
生成溯源树,研究论文发展脉络
Chat Paper
正在生成论文摘要