Adaptation Through Prediction: Multisensory Active Inference Torque Control
IEEE Transactions on Cognitive and Developmental Systems(2023)
摘要
Adaptation to external and internal changes is of major importance for robotic systems in uncertain environments. Here, we present a novel multisensory active inference (AIF) torque controller for industrial arms that shows how prediction can be used to resolve adaptation. Our controller, inspired by the predictive brain hypothesis, improves the capabilities of current AIF approaches by incorporating learning and multimodal integration of low- and high-dimensional sensor inputs (e.g., raw images) while simplifying the architecture. We performed a systematic evaluation of our model on a 7DoF Franka Emika Panda robot arm by comparing its behavior with previous AIF baselines and classic controllers, analyzing both qualitatively and quantitatively adaptation capabilities and control accuracy. The results showed improved control accuracy in goal-directed reaching with high noise rejection due to multimodal filtering, and adaptability to dynamical inertial changes, elasticity constraints, and human disturbances without the need to relearn the model or parameter retuning.
更多查看译文
关键词
Bio-inspired robotics,robot control,representation learning
AI 理解论文
溯源树
样例
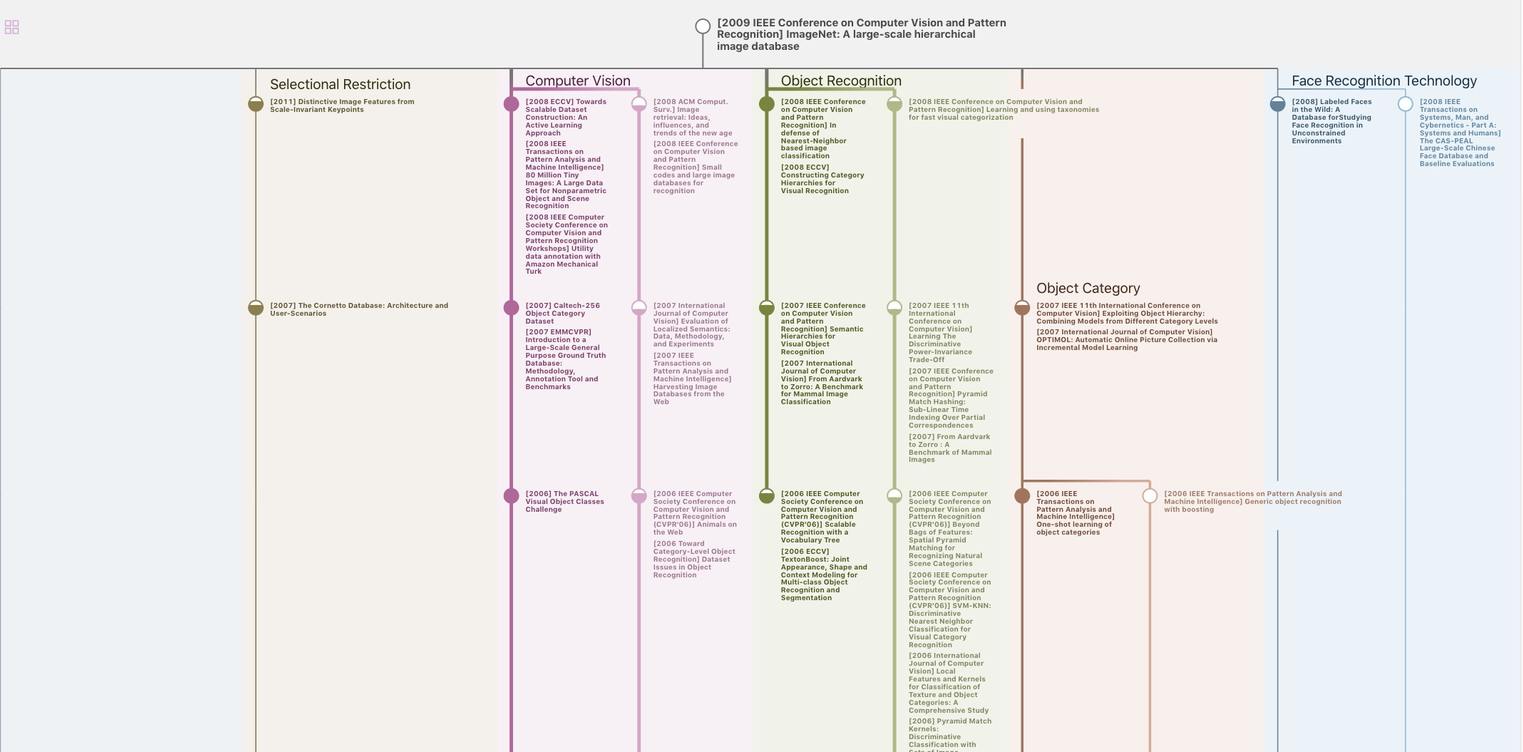
生成溯源树,研究论文发展脉络
Chat Paper
正在生成论文摘要