In vitro validation of neoantigen prediction algorithm for developing personalized cancer vaccine therapy
biorxiv(2021)
摘要
Background The development of personalized neoantigen-based therapeutic cancer vaccines relies on computational algorithm-based pipelines. One of the critical issues in the pipeline is obtaining higher positive predictive value (PPV) performance, i.e., how many are immunogenic when selecting the top 5 to 20 candidate neoepitopes for the vaccination. We attempted to test the PPV of a neoepitope prediction algorithm Neopepsee.
Methods Six breast cancer patients and patient-derived xenografts from three lung cancer patients and their paired peripheral blood samples were subjected to whole-exome and RNA sequencing. Neoantigen was predicted using two different algorithms (Neopepsee and pVACseq). Response of induced memory T cells to neopeptide candidates was evaluated by IFN-γ Enzyme-linked immune absorbent spot (ELISpot) assays of peripheral blood mononuclear cell (PBMC) from three HLA-matched donors. Positive ELISpot response to a candidate peptide in at least 2 of 3 donor PBMC was regarded as an immunogenic response.
Results Neopepsee predicted 159 HLA-A matched neoepitope candidates out of 898 somatic mutations in nine patients (six breast and three lung cancer patients), whereas pVACseq predicted 84 HLA-A matched candidates. A total of 26 neopeptide candidates overlapped between the two predicted candidate pools. Among the candidates, 28 (20%, 28/ 137) and 15 (20%, 15/ 75) were positive by ELISpot assay, respectively. Among 26 overlapped candidates, 20 could be tested, and 7 of them (35%) were validated by ELISpot. Neopepsee identified at least one neoepitope in 7 of 9 patients (range 0-6), compared to 6 by pVACseq (range 0-5).
Conclusion As suggested by Tumor Neoantigen Selection Alliance (TESLA), our results demonstrate low PPV of individual prediction models as well as the complementary nature of the Neopepsee and pVACseq and may help design neoepitope targeted cancer vaccines. Our data contribute a significant addition to the database of tested neoepitope candidates that can be utilized to further train and improve the prediction algorithms.
### Competing Interest Statement
The authors have declared no competing interest.
更多查看译文
AI 理解论文
溯源树
样例
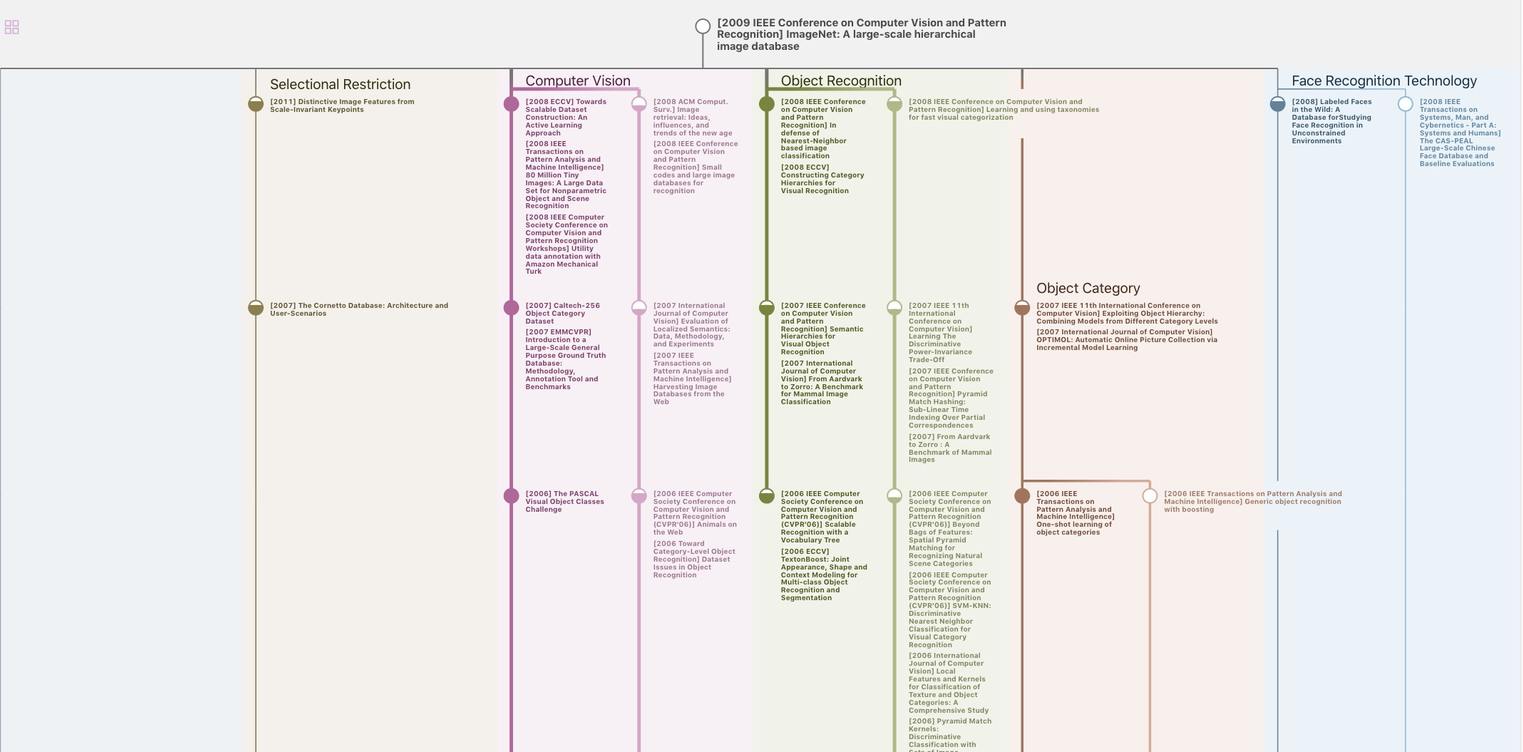
生成溯源树,研究论文发展脉络
Chat Paper
正在生成论文摘要