How to Learn when Data Gradually Reacts to Your Model
INTERNATIONAL CONFERENCE ON ARTIFICIAL INTELLIGENCE AND STATISTICS, VOL 151(2022)
摘要
A recent line of work has focused on training machine learning (ML) models in the performative setting, i.e. when the data distribution reacts to the deployed model. The goal in this setting is to learn a model which both induces a favorable data distribution and performs well on the induced distribution, thereby minimizing the test loss. Previous work on finding an optimal model assumes that the data distribution immediately adapts to the deployed model. In practice, however, this may not be the case, as the population may take time to adapt to the model. In many applications, the data distribution depends on both the currently deployed ML model and on the "state" that the population was in before the model was deployed. In this work, we propose a new algorithm, Stateful Performative Gradient Descent (Stateful PerfGD), for minimizing the performative loss even in the presence of these effects. We provide theoretical guarantees for the convergence of Stateful PerfGD. Our experiments confirm that Stateful PerfGD substantially outperforms previous state-of-the-art methods.
更多查看译文
关键词
data gradually reacts,model
AI 理解论文
溯源树
样例
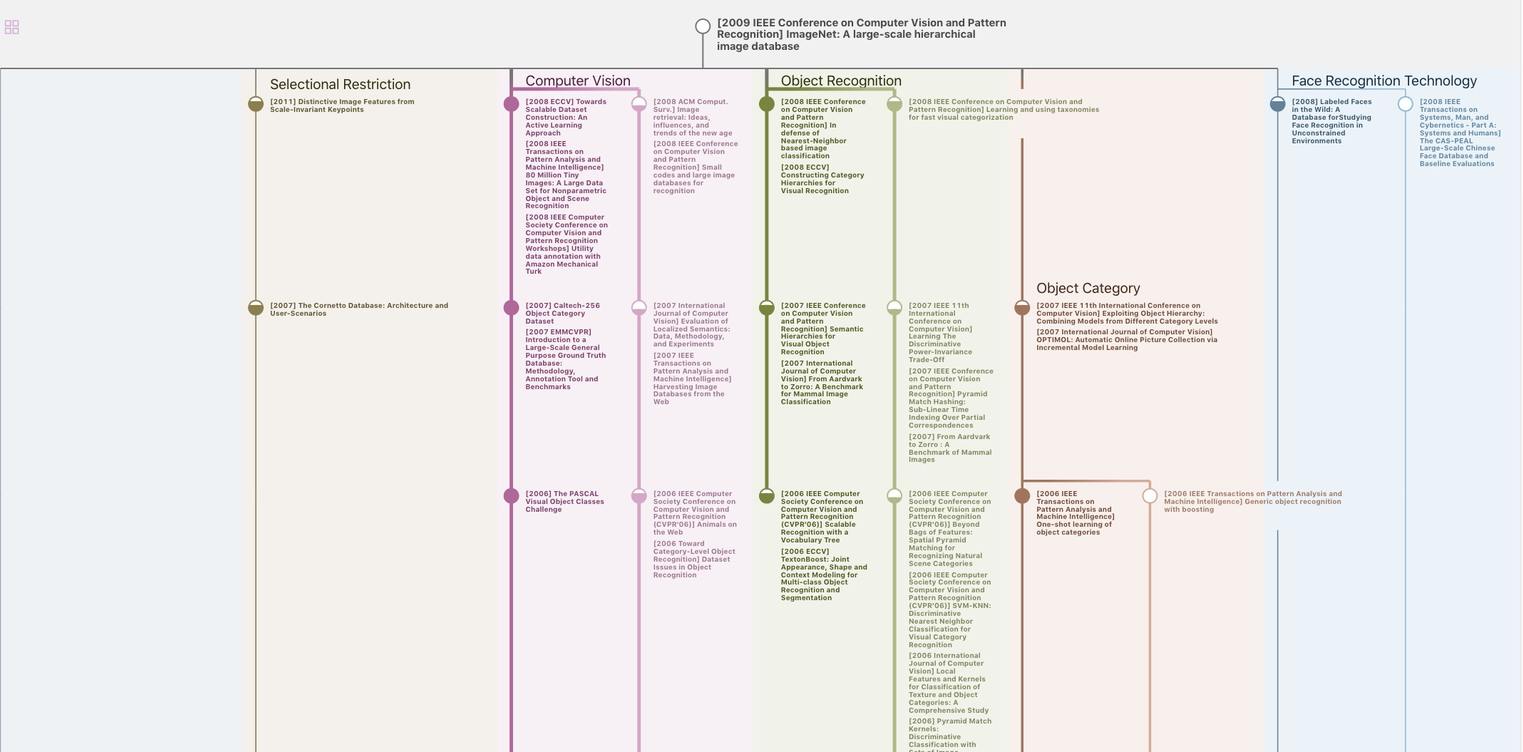
生成溯源树,研究论文发展脉络
Chat Paper
正在生成论文摘要