Learning Connectivity-Maximizing Network Configurations
IEEE ROBOTICS AND AUTOMATION LETTERS(2022)
摘要
In this letter we propose a data-driven approach to optimizing the algebraic connectivity of a team of robots. While a considerable amount of research has been devoted to this problem, we lack a method that scales in a manner suitable for online applications for more than a handful of agents. To that end, we propose a supervised learning approach with a convolutional neural network (CNN) that learns to place communication agents from an expert that uses an optimization-based strategy. We demonstrate the performance of our CNN on canonical line and ring topologies, 105 k randomly generated test cases, and larger teams not seen during training. We also show how our system can be applied to dynamic robot teams through a Unity-based simulation. After training, our system produces connected configurations over an order of magnitude faster than the optimization-based scheme for teams of 10-20 agents.
更多查看译文
关键词
Deep learning methods,multi-robot systems,networked robots
AI 理解论文
溯源树
样例
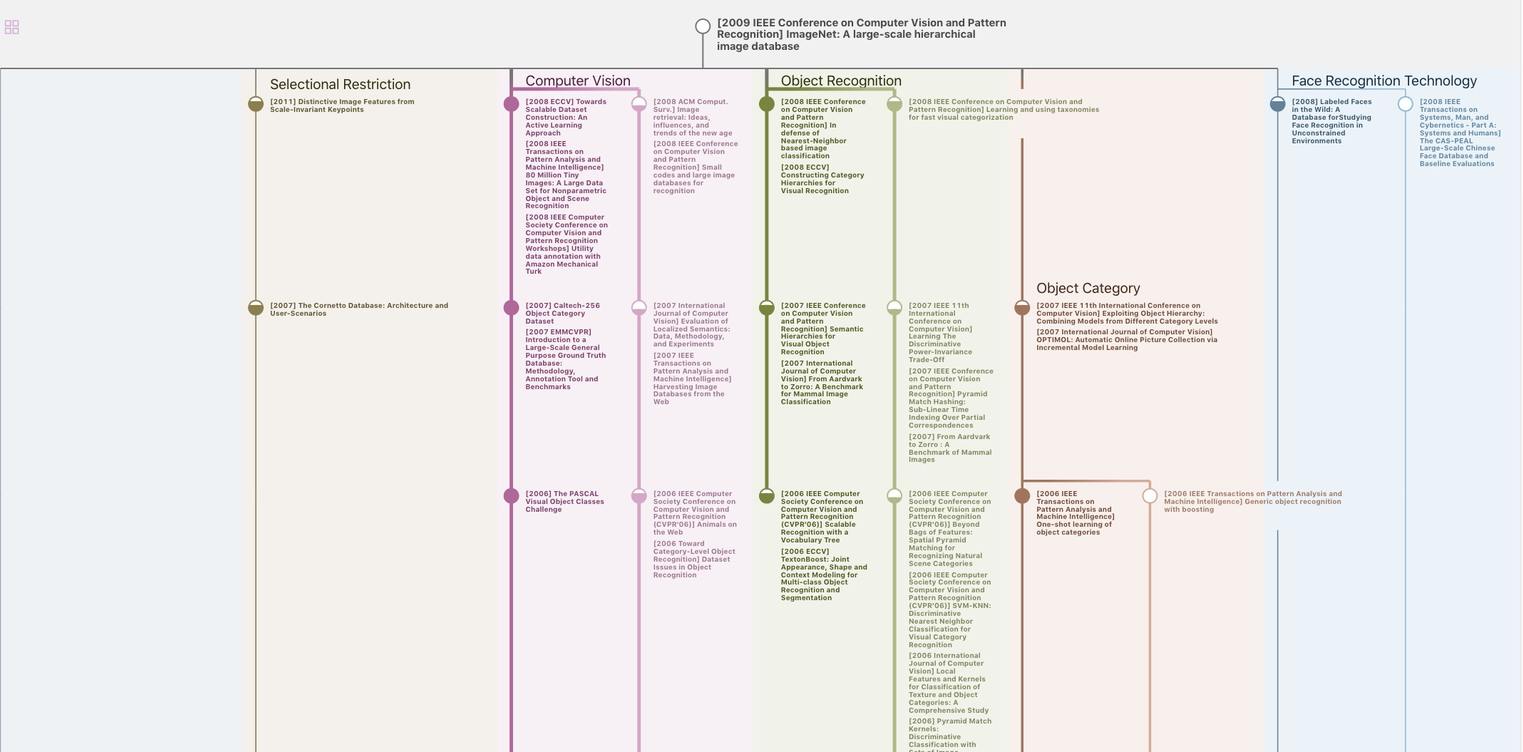
生成溯源树,研究论文发展脉络
Chat Paper
正在生成论文摘要