Emulation-accelerated Hamiltonian Monte Carlo algorithms for parameter estimation and uncertainty quantification in differential equation models
STATISTICS AND COMPUTING(2021)
摘要
We propose to accelerate Hamiltonian and Lagrangian Monte Carlo algorithms by coupling them with Gaussian processes for emulation of the log unnormalised posterior distribution. We provide proofs of detailed balance with respect to the exact posterior distribution for these algorithms, and validate the correctness of the samplers’ implementation by Geweke consistency tests. We implement these algorithms in a delayed acceptance (DA) framework, and investigate whether the DA scheme can offer computational gains over the standard algorithms. A comparative evaluation study is carried out to assess the performance of the methods on a series of models described by differential equations, including a real-world application of a 1D fluid-dynamics model of the pulmonary blood circulation. The aim is to identify the algorithm which gives the best trade-off between accuracy and computational efficiency, to be used in nonlinear DE models, which are computationally onerous due to repeated numerical integrations in a Bayesian analysis. Results showed no advantage of the DA scheme over the standard algorithms with respect to several efficiency measures based on the effective sample size for most methods and DE models considered. These gradient-driven algorithms register a high acceptance rate, thus the number of expensive forward model evaluations is not significantly reduced by the first emulator-based stage of DA. Additionally, the Lagrangian Dynamical Monte Carlo and Riemann Manifold Hamiltonian Monte Carlo tended to register the highest efficiency (in terms of effective sample size normalised by the number of forward model evaluations), followed by the Hamiltonian Monte Carlo, and the No U-turn sampler tended to be the least efficient.
更多查看译文
关键词
Parameter estimation, Uncertainty quantification, MCMC, Emulation, Gaussian processes, Differential equations
AI 理解论文
溯源树
样例
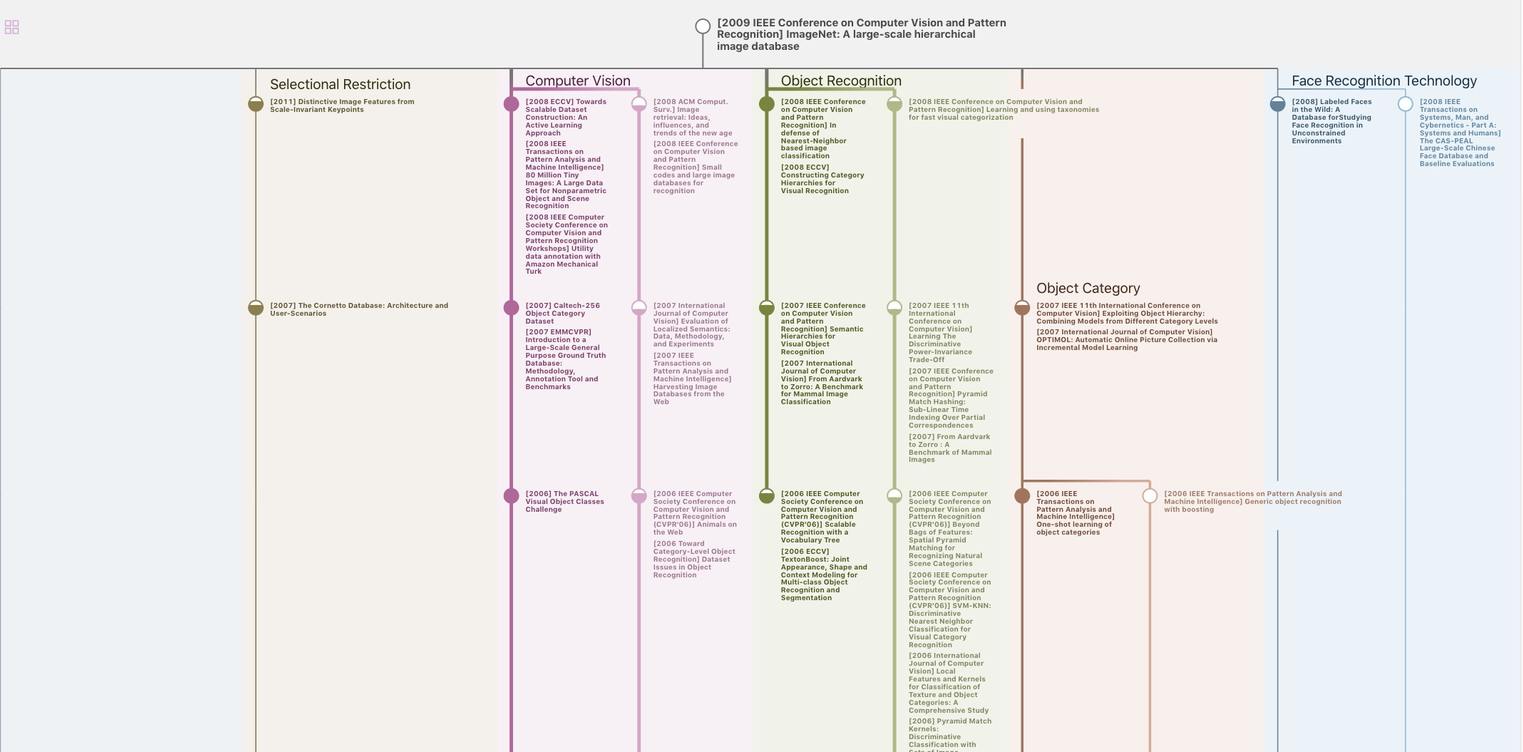
生成溯源树,研究论文发展脉络
Chat Paper
正在生成论文摘要