Generating Adversarial Examples by Distributed Upsampling.
ICONIP(2021)
摘要
The development of neural networks provides state-of-the-art results for many tasks. However, much research has shown that deep neural networks are vulnerable to adversarial attacks that fool deep models by adding small perturbations into original images. The defense of the neural networks also benefits from a better understanding of the attacks. In consequence, generating adversarial examples with a high attack success rate is worth researching. Inspired by single image super-resolution, this paper treats adversarial attacks as an image generation task and designs a new model based on generative adversarial networks (GANs). The latent feature maps have been divided into low-level and high-level in this research. We exploit low-level features with noises to add perturbations during the upsampling process. To further generate perturbed images, we reconsider and make use of checkerboard artifacts caused by deconvolution. We illustrate the performance of our method using experiments conducted on MNIST and CIFAR-10. The experiment results prove that adversarial examples generated by our method achieve a higher attack success rate and better transferability.
更多查看译文
关键词
adversarial examples,upsampling
AI 理解论文
溯源树
样例
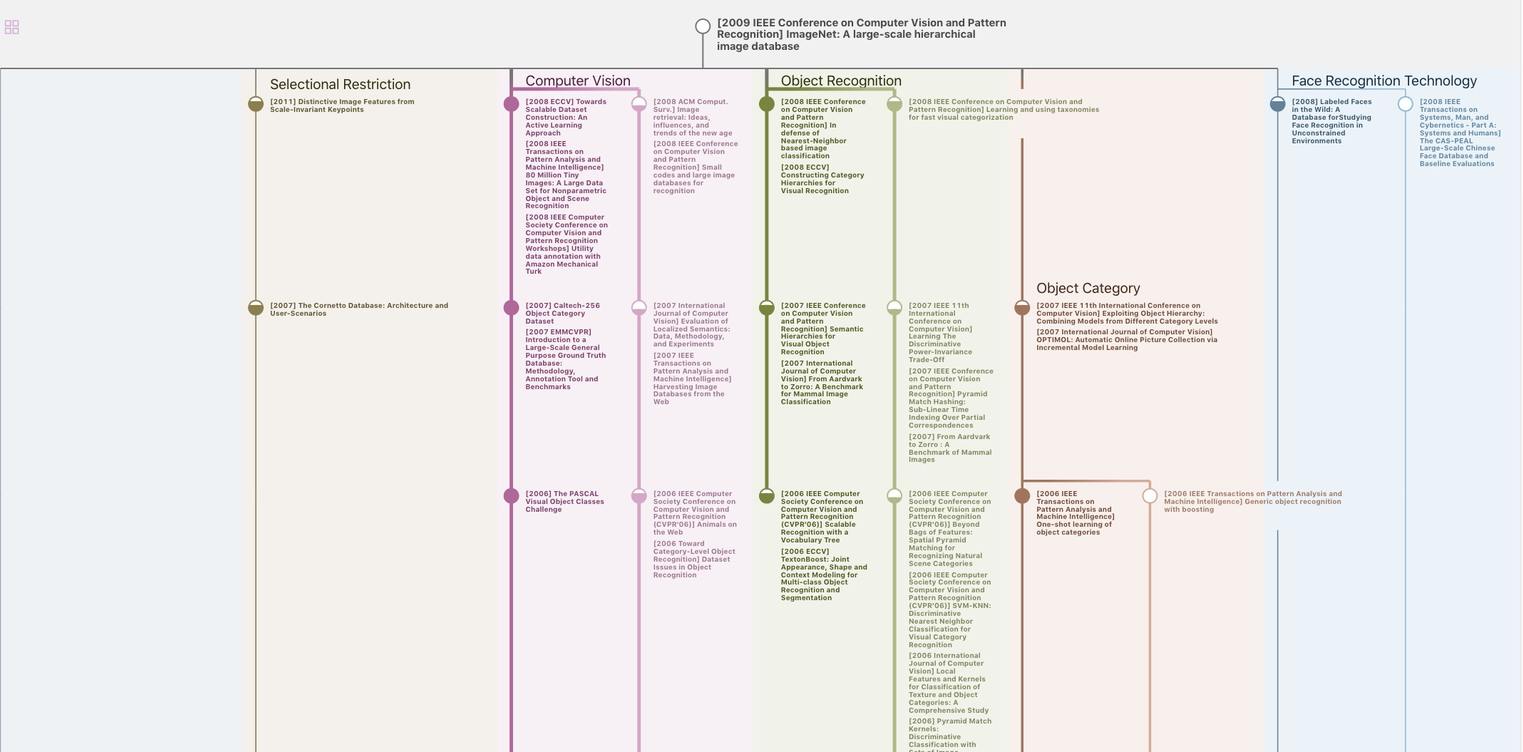
生成溯源树,研究论文发展脉络
Chat Paper
正在生成论文摘要