Deep Kernelized Network for Fine-Grained Recognition.
ICONIP(2021)
摘要
Convolutional Neural Networks (CNNs) are based on linear kernel at different levels of the network. Linear kernels are not efficient, particularly, when the original data is not linearly separable. In this paper, we focus on this issue by investigating the impact of using higher order kernels. For this purpose, we replace convolution layers with Kervolution layers proposed in [ 28 ]. Similarly, we replace fully connected layers alternatively with Kernelized Dense Layers (KDL) proposed in [ 16 ] and Kernel Support vector Machines (SVM) [ 1 ]. These kernel-based methods are more discriminative in the way that they can learn more complex patterns compared to the linear one. Those methods first maps input data to a higher space. After that, they learn a linear classifier in that space which is similar to a powerful non-linear classifier in the first space. We have used Fine-Grained datasets namely FGVC-Aircraft, StanfordCars and CVPRIndoor as well as Facial Expression Recognition (FER) datasets namely, RAF-DB, ExpW and FER2013 to evaluate the performance of these methods. The experimental results demonstrate that these methods outperform the ordinary linear layers when used in a deep network fashion.
更多查看译文
关键词
Facial expression recognition, Fine-grained recognition, Kernel function, Deep learning
AI 理解论文
溯源树
样例
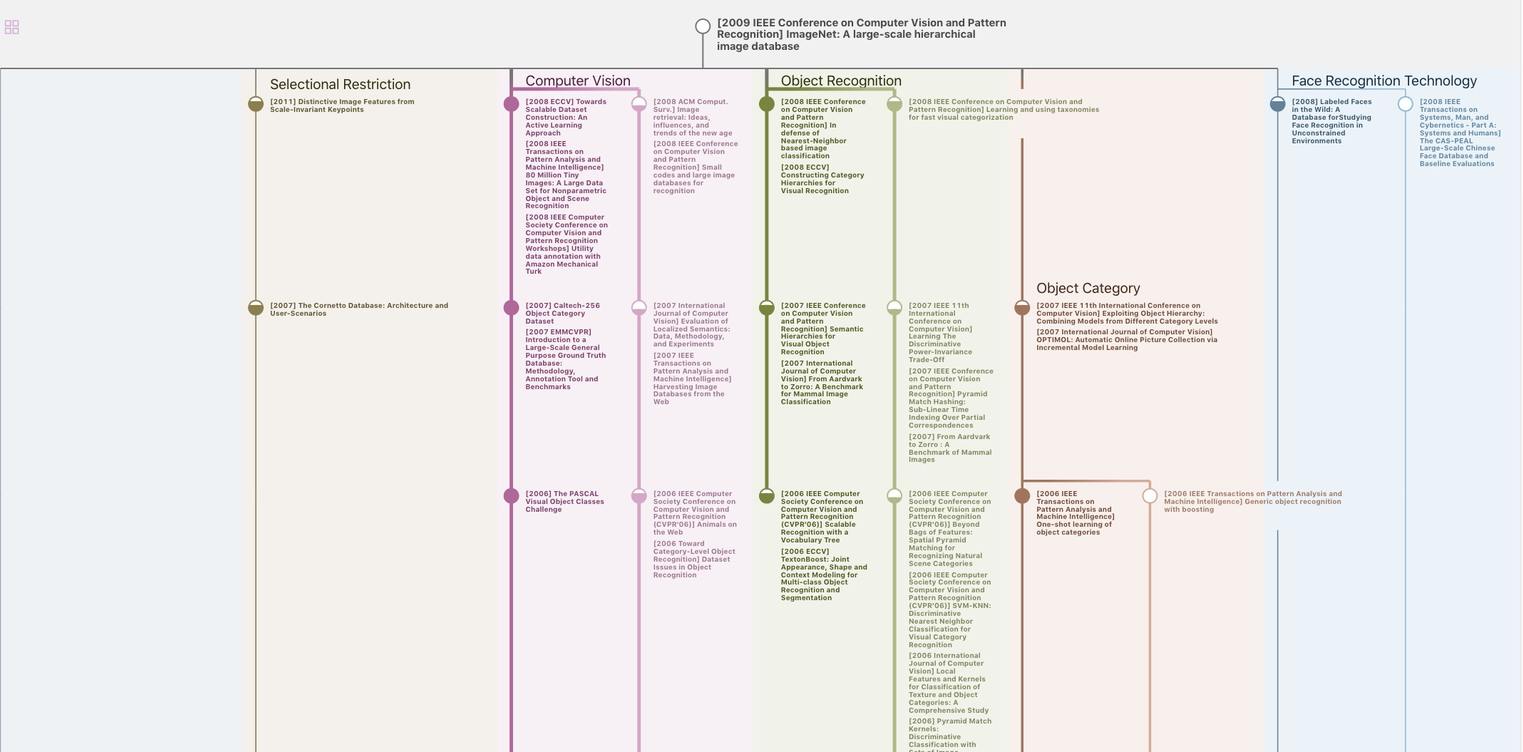
生成溯源树,研究论文发展脉络
Chat Paper
正在生成论文摘要