Towards Human-Level Performance in Solving Double Dummy Bridge Problem.
ICONIP(2021)
摘要
Double Dummy Bridge Problem (DDBP) is a hard classification problem that consists in estimating the number of tricks to be taken by N-S pair during a bridge game. In this paper we propose a new approach to DDBP which utilizes convolutional neural networks (CNNs) and a dedicated matrix representation of the problem, suitable for the CNN application. Following previous studies on the application of neural networks to DDBP, we take a knowledge-free approach, i.e. the CNN models are trained with no use of any expert knowledge or explicitly indicated bridge rules. As a result, two models are derived: a baseline CNN model and its ensemble refinement. The results are compared with two former neural network approaches, showing significant superiority of the CNN-based solution. Depending on the type DDBP deal, i.e. trump or notrump, our approach either outperforms or is slightly inferior to the outcomes of human bridge grandmasters solving DDBP. This state-of-the-art performance is complemented in the paper with an analysis of the internal structure (weight patterns) of the trained CNNs, which partly explains the underlying classification process.
更多查看译文
AI 理解论文
溯源树
样例
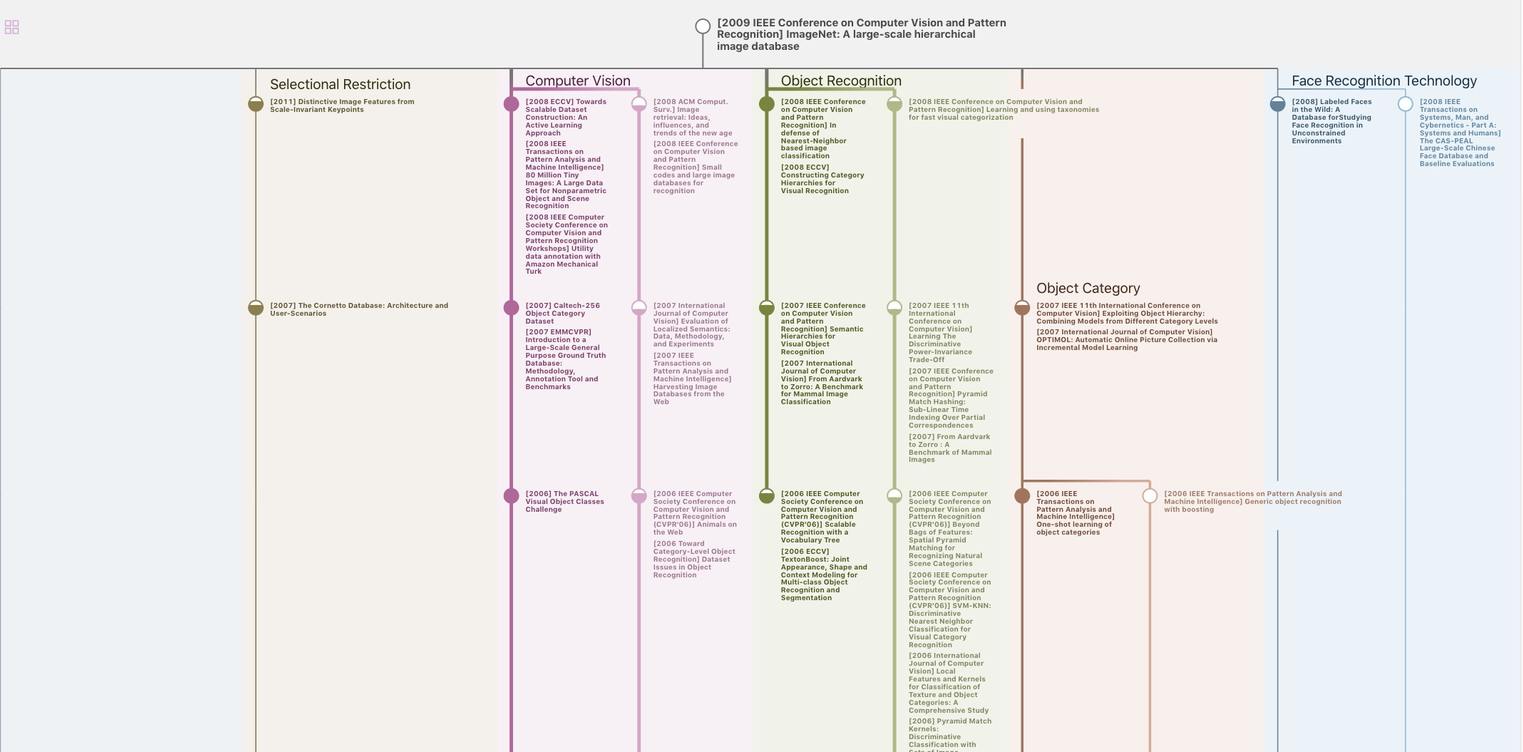
生成溯源树,研究论文发展脉络
Chat Paper
正在生成论文摘要