Identity-Based Data Augmentation via Progressive Sampling for One-Shot Person Re-identification.
ICONIP(2021)
摘要
One-shot person re-identification (Re-ID) is a hot spot nowadays, where there is only one labeled image along with many unlabeled images for each identity. Due to the short of labeled training images, it’s hard to catch up with performance under full supervision. In this paper, we propose a progressive method with identity-based data augmentation to improve lack of supervision information, which takes advantage of information of each identity to generate high-quality images. Specifically, with a certain image-to-image translation model, images are decoupled into content and style codes, where the images holding the features of identity well and injected in the style codes exclusive to the identity can be obtained by labeled images through the process of recombination. A progressive data augmentation method for one-shot labeled samples is also designed to optimize the sampling accuracy of pseudo labeled images, which contributes to our identity-based data augmentation process. The experimental results show that our method represents new state-of-the-art one-shot Re-ID work.
更多查看译文
关键词
data augmentation,progressive sampling,person,identity-based,one-shot,re-identification
AI 理解论文
溯源树
样例
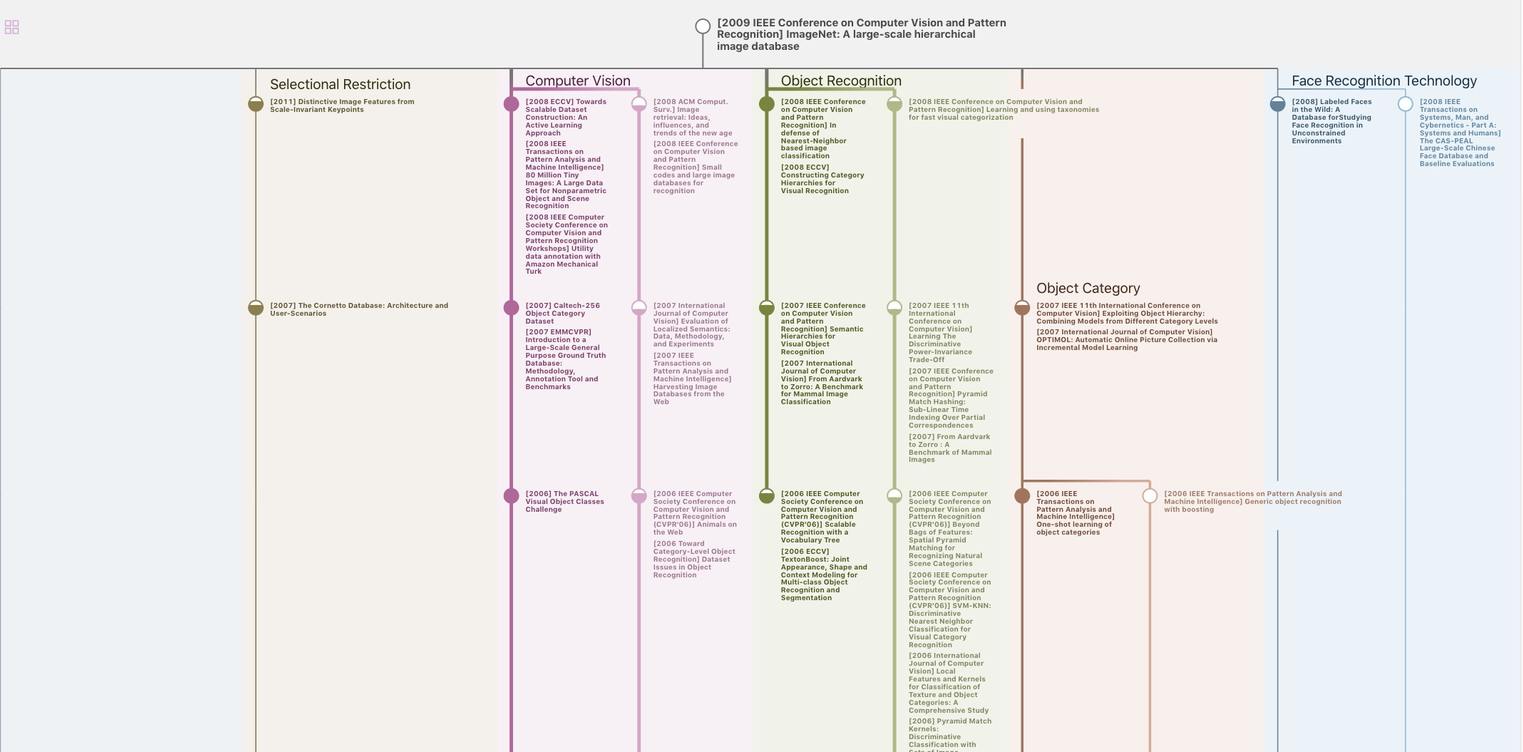
生成溯源树,研究论文发展脉络
Chat Paper
正在生成论文摘要