NeuroLogic A*esque Decoding: Constrained Text Generation with Lookahead Heuristics
North American Chapter of the Association for Computational Linguistics (NAACL)(2022)
摘要
The dominant paradigm for neural text generation is left-to-right decoding from autoregressive language models. Constrained or controllable generation under complex lexical constraints, however, requires foresight to plan ahead feasible future paths. Drawing inspiration from the A* search algorithm, we propose NeuroLogic A*esque, a decoding algorithm that incorporates heuristic estimates of future cost. We develop efficient lookahead heuristics that are efficient for large-scale language models, making our method a drop-in replacement for common techniques such as beam search and top-k sampling. To enable constrained generation, we build on NeuroLogic decoding (Lu et al., 2021), combining its flexibility in incorporating logical constraints with A*esque estimates of future constraint satisfaction. Our approach outperforms competitive baselines on five generation tasks, and achieves new state-of-the-art performance on table-to-text generation, constrained machine translation, and keyword-constrained generation. The improvements are particularly notable on tasks that require complex constraint satisfaction or in few-shot or zero-shot settings. NeuroLogic A*esque illustrates the power of decoding for improving and enabling new capabilities of large-scale language models.
更多查看译文
关键词
constrained text generation,lookahead
AI 理解论文
溯源树
样例
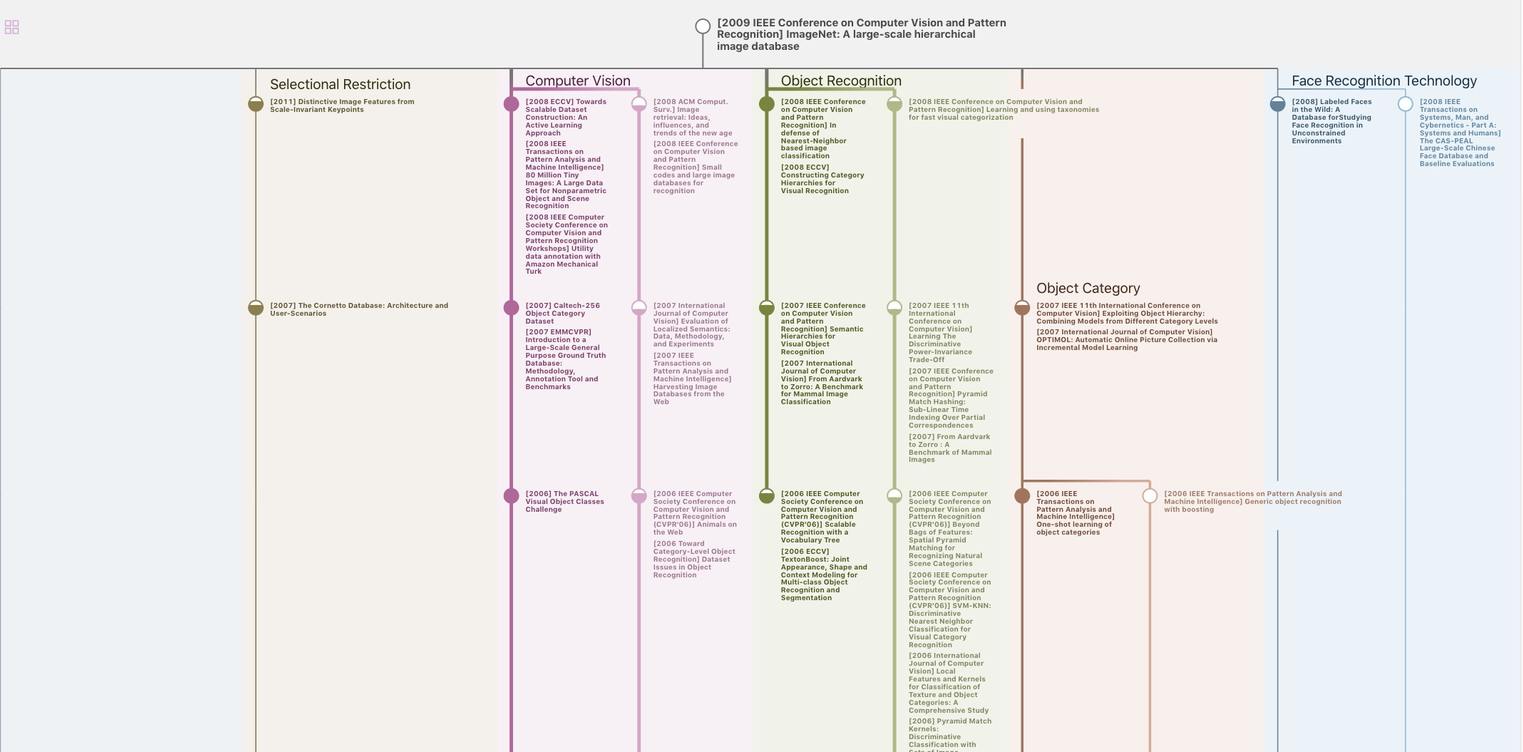
生成溯源树,研究论文发展脉络
Chat Paper
正在生成论文摘要