Up-to-Down Network: Fusing Multi-Scale Context for 3D Semantic Scene Completion
2021 IEEE/RSJ INTERNATIONAL CONFERENCE ON INTELLIGENT ROBOTS AND SYSTEMS (IROS)(2021)
摘要
An efficient 3D scene perception algorithm is a vital component for autonomous driving and robotics systems. In this paper, we focus on semantic scene completion, which is a task of jointly estimating the volumetric occupancy and semantic labels of objects. Since the real-world data is sparse and occluded, this is an extremely challenging task. We propose a novel framework, named Up-to-Down network (UDNet), to achieve the large-scale semantic scene completion with an encoder-decoder architecture for voxel grids. The novel up-to-down block can effectively aggregate multi-scale context information to improve labeling coherence, and the atrous spatial pyramid pooling module is leveraged to expand the receptive field while preserving detailed geometric information. Besides, the proposed multi-scale fusion mechanism efficiently aggregates global background information and improves the semantic completion accuracy. Moreover, to further satisfy the needs of different tasks, our UDNet can accomplish the multi-resolution semantic completion, achieving faster but coarser completion. Detailed experiments in the semantic scene completion benchmark of SemanticKITTI illustrate that our proposed framework surpasses the state-of-the-art methods with remarkable margins and a real-time inference speed by using only voxel grids as input.
更多查看译文
关键词
Three-dimensional displays,Aggregates,Semantics,Coherence,Benchmark testing,Real-time systems,Labeling
AI 理解论文
溯源树
样例
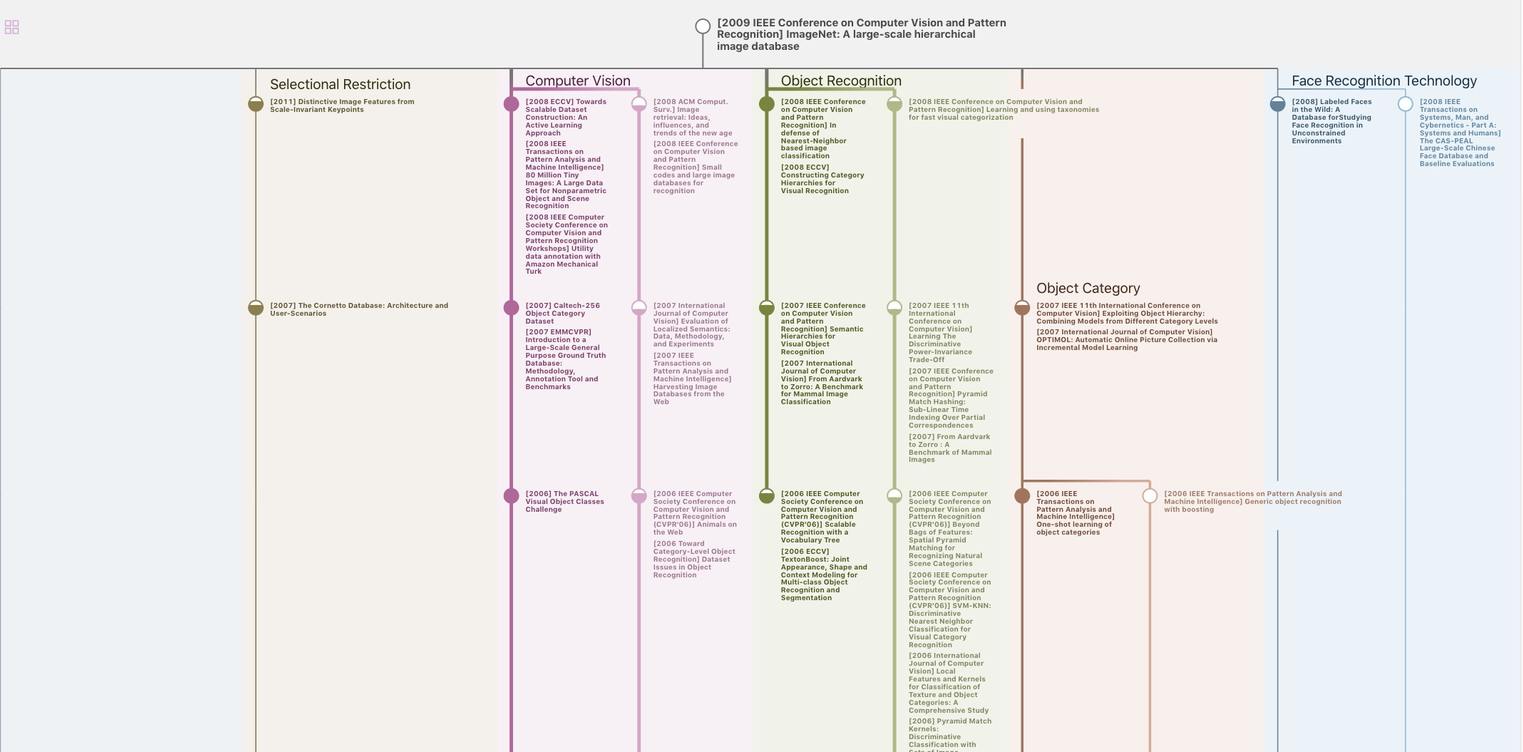
生成溯源树,研究论文发展脉络
Chat Paper
正在生成论文摘要