An encoder-decoder based deep architecture for visible to near infrared image transformation.
ICVGIP(2021)
摘要
Near-infrared (NIR) band is also one of the exciting wavelength ranges, besides optical range, to the human visual perception system. The NIR band shares various attributes with visible spectrum range. NIR images have the potential for better representation of vegetation and certain details of a scene that are sometimes opaque to RGB wavelengths. In this paper, we propose a dual encoder-decoder based architecture with different depths for transforming RGB images to NIR images. This network handles scale variations in data and helps to reduce artifacts in the transformed image domain. Two different resolutions of the input image are subjected to feature extraction by a series of five consecutive convolution and ReLu layers. Then, their corresponding aggregated features are assembled and up-sampled to regress over the ground truth NIR image. We consider two datasets with different resolutions and properties, EPFL and OMSIV, which provide corresponding RGB-NIR pairs of images of natural scenes. We train and test our network on both the datasets and evaluate its qualitative and quantitative performance. The results of our network in transforming RGB images to NIR images are found to be interesting which are demonstrated through various examples.
更多查看译文
AI 理解论文
溯源树
样例
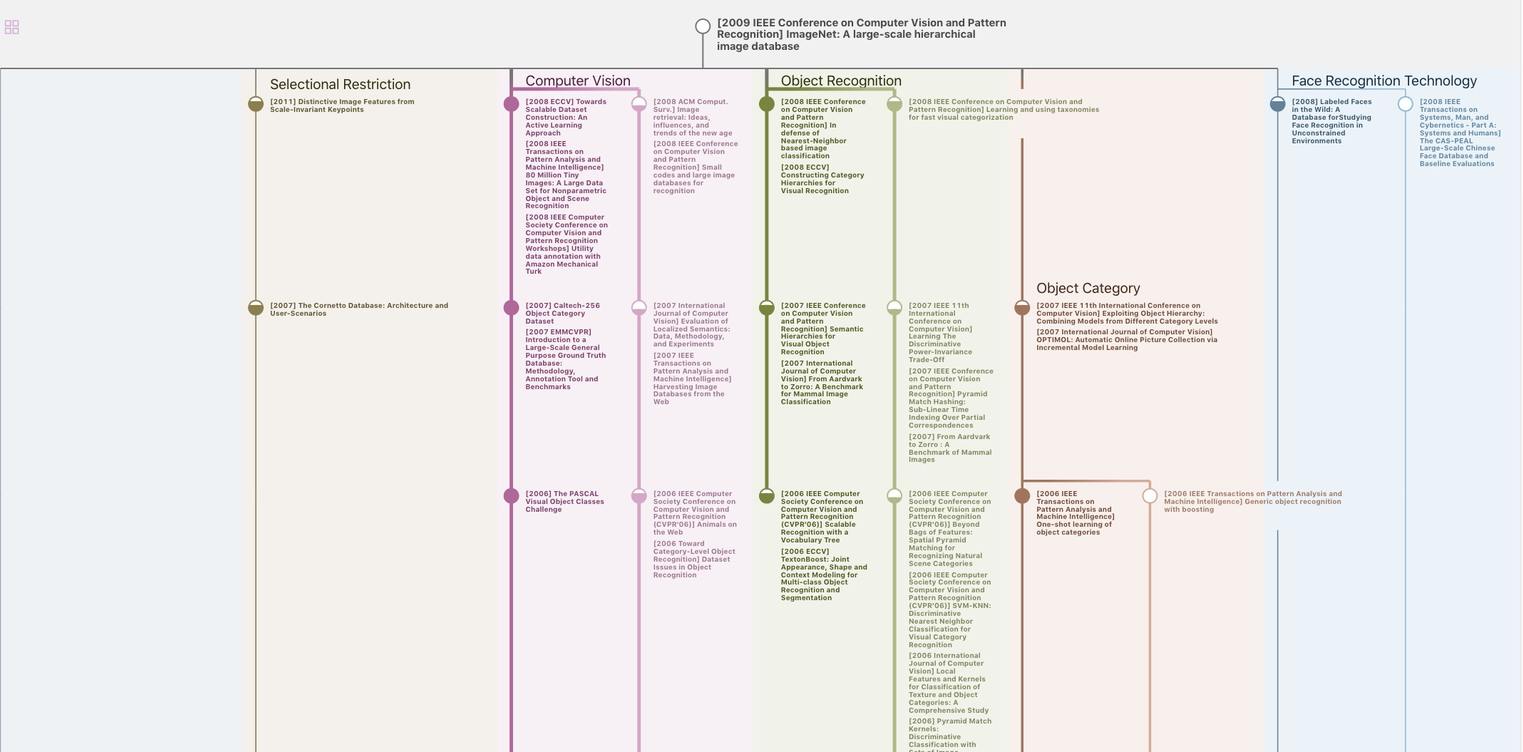
生成溯源树,研究论文发展脉络
Chat Paper
正在生成论文摘要