Using Automated Feature Selection for Building Case-Based Reasoning Systems: An Example from Patient-Reported Outcome Measurements
ARTIFICIAL INTELLIGENCE XXXVIII(2021)
摘要
Feature selection for case representation is an essential phase of Case-Based Reasoning (CBR) system development. To (semi-)automate the feature selection process can ease the knowledge engineering process. This paper explores the feature importance provided for XGBoost models as basis for creating CBR systems. We use Patient-Reported Outcome Measurements (PROMs) on low back pain from the selfBACK project in our experiments. PROMs are a valuable source of information that capture physical, emotional as well as social aspects of well-being from the perspective of the patients. Leveraging the analytical capabilities of machine learning methods and data science techniques for exploiting PROMs have the potential of improving decision making. This paper presents a two-fold approach employed on our dataset for feature selection that combines statistical strength with data-driven knowledge modelling in CBR and compares it with permutation feature selection using XGBoost regressor. Furthermore, we compare the performance of the CBR models, built with the selected features, with two machine learning algorithms for predicting different PROMs.
更多查看译文
关键词
Case-based reasoning,Feature selection,Case representation,Patient-reported outcome measurements
AI 理解论文
溯源树
样例
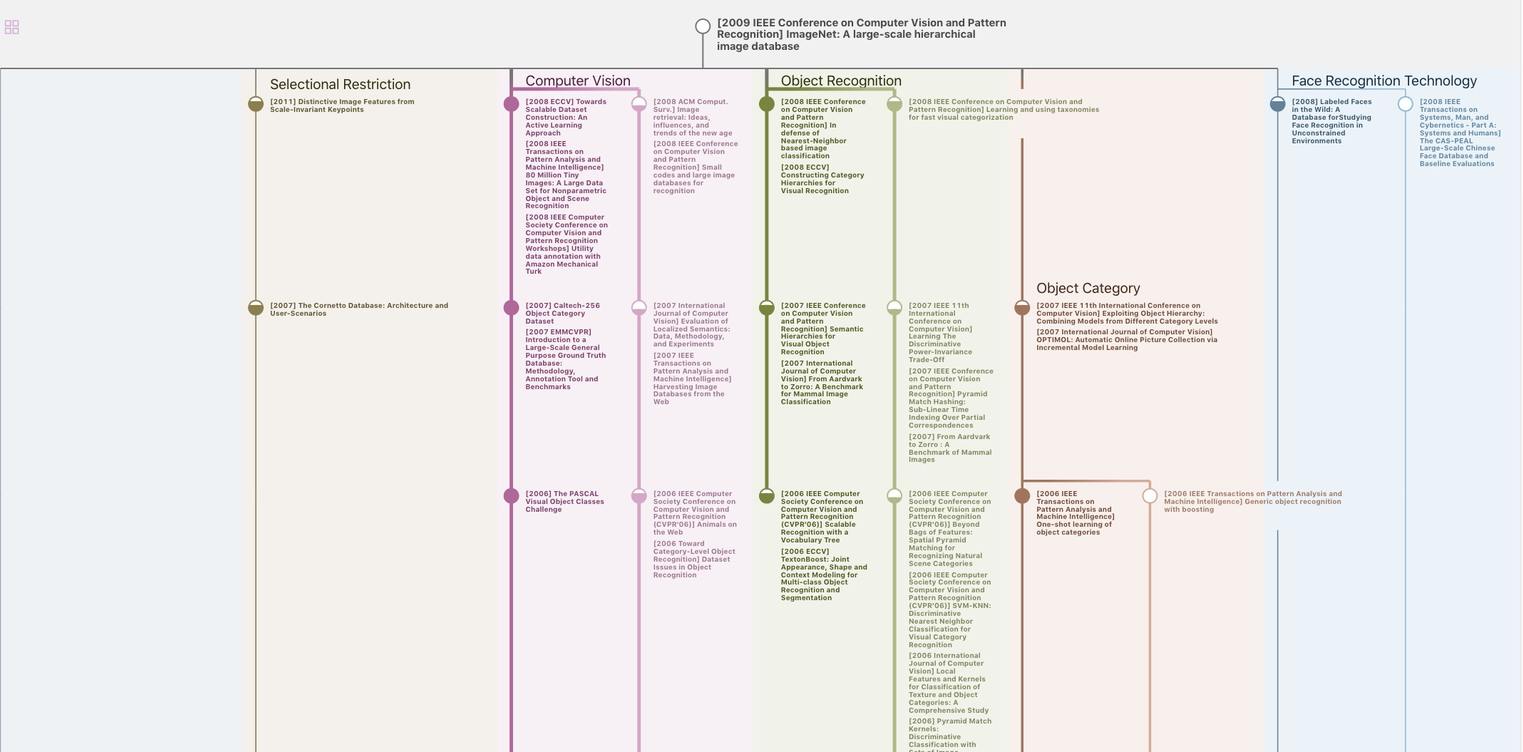
生成溯源树,研究论文发展脉络
Chat Paper
正在生成论文摘要