A Data-Centric Weak Supervised Learning for Highway Traffic Incident Detection
Accident analysis and prevention(2022)
摘要
Using the data from loop detector sensors for near-real-time detection of traffic incidents on highways is crucial to averting major traffic congestion. While recent supervised machine learning methods offer solutions to incident detection by leveraging human-labeled incident data, the false alarm rate is often too high to be used in practice. Specifically, the inconsistency in the human labeling of the incidents significantly affects the performance of supervised learning models. To that end, we focus on a data-centric approach to improve the accuracy and reduce the false alarm rate of traffic incident detection on highways. We develop a weak supervised learning workflow to generate high-quality training labels for the incident data without the ground truth labels, and we use those generated labels in the supervised learning setup for final detection. This approach comprises three stages. First, we introduce a data preprocessing and curation pipeline that processes traffic sensor data to generate high-quality training data through leveraging labeling functions, which can be domain knowledge-related or simple heuristic rules. Second, we evaluate the training data generated by weak supervision using three supervised learning models—random forest, k-nearest neighbors, and a support vector machine ensemble—and long short-term memory classifiers. The results show that the accuracy of all of the models improves significantly after using the training data generated by weak supervision. Third, we develop an online real-time incident detection approach that leverages the model ensemble and the uncertainty quantification while detecting incidents. Overall, we show that our proposed weak supervised learning workflow achieves a high incident detection rate (0.90) and low false alarm rate (0.08).
更多查看译文
关键词
Data-centric machine learning,Traffic incident detection,Recurrent neural network,Weak supervision
AI 理解论文
溯源树
样例
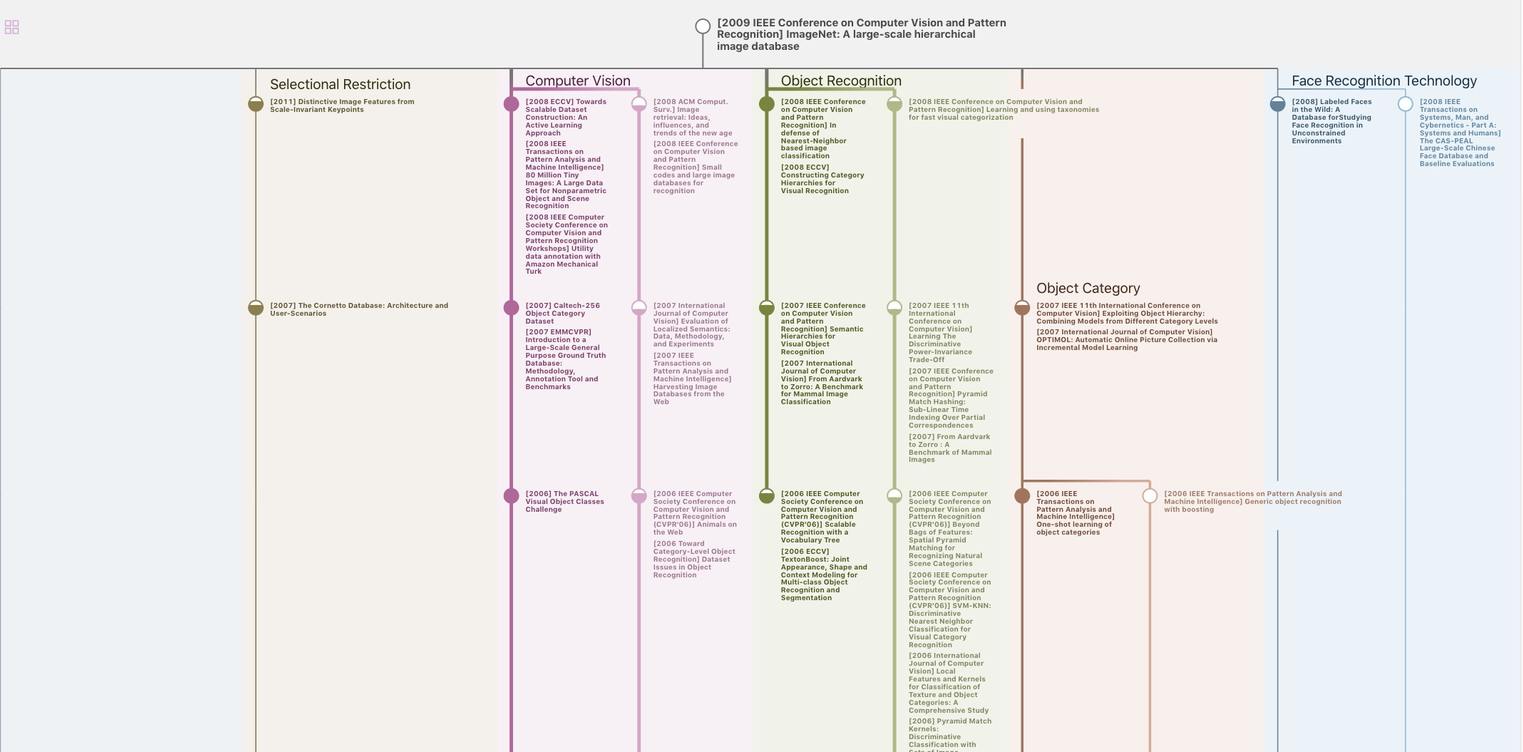
生成溯源树,研究论文发展脉络
Chat Paper
正在生成论文摘要