Efficiency of ETA Prediction
arxiv(2023)
摘要
Modern mobile applications such as navigation services and ride-sharing platforms rely heavily on geospatial technologies, most critically predictions of the time required for a vehicle to traverse a particular route, or the so-called estimated time of arrival (ETA). There are various methods used in practice, which differ in terms of the geographic granularity at which the predictive model is trained -- e.g., segment-based methods predict travel time at the level of road segments (or a combination of several adjacent road segments) and then aggregate across the route, whereas route-based methods use generic information about the trip, such as origin and destination, to predict travel time. Though various forms of these methods have been developed, there has been no rigorous theoretical comparison regarding their accuracies, and empirical studies have, in many cases, drawn opposite conclusions. We provide the first theoretical analysis of the predictive accuracy of various ETA prediction methods. In a finite-sample setting, we give mild conditions under which a segment-based method is more accurate than a wide variety of route-based methods. Then we analyze an asymptotic setting in which the number of trip observations grows with the size of the road network. Under a broad range of trip-generating processes on a grid network, we show that a class of very simple segment-based methods is at least as good, up to a logarithmic factor, as any possible predictor. In other words, segment-based methods are asymptotically optimal up to a logarithmic factor. Our work highlights that the accuracy of ETA prediction is driven not just by the sophistication of the model but also by the spatial granularity at which those methods are applied.
更多查看译文
关键词
eta prediction,efficiency
AI 理解论文
溯源树
样例
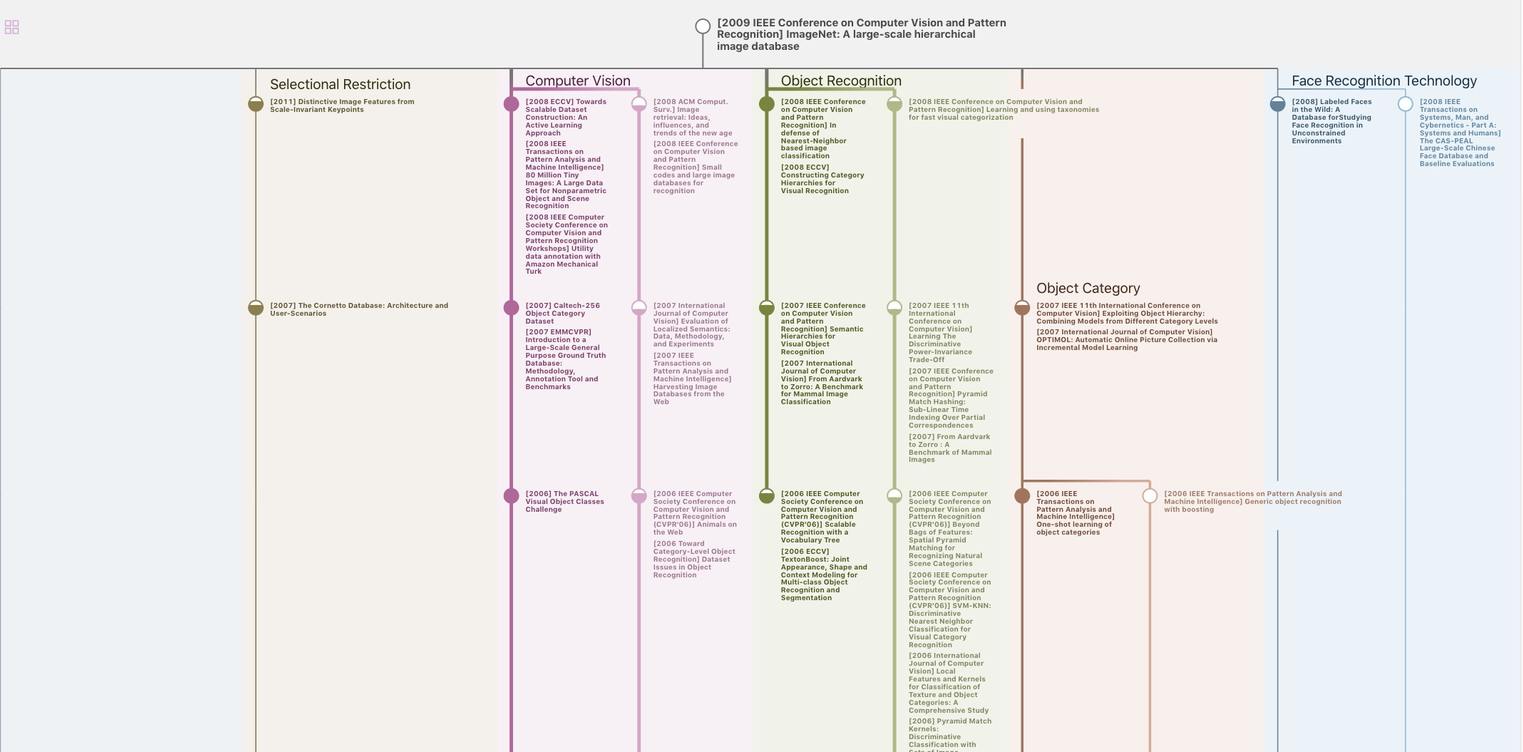
生成溯源树,研究论文发展脉络
Chat Paper
正在生成论文摘要