Optimization for Master-UAV-Powered Auxiliary-Aerial-IRS-Assisted IoT Networks: An Option-Based Multi-Agent Hierarchical Deep Reinforcement Learning Approach
IEEE Internet of Things Journal(2022)
摘要
This article investigates a master unmanned aerial vehicle (MUAV)-powered Internet of Things (IoT) network, in which we propose using a rechargeable auxiliary UAV (AUAV) equipped with an intelligent reflecting surface (IRS) to enhance the communication signals from the MUAV and also leverage the MUAV as a recharging power source. Under the proposed model, we investigate the optimal collaboration strategy of these energy-limited UAVs to maximize the accumulated throughput of the IoT network. Depending on whether there is charging between the two UAVs, two optimization problems are formulated. To solve them, two multi-agent deep reinforcement learning (DRL) approaches are proposed, which are centralized training multi-agent deep deterministic policy gradient (CT-MADDPG) and multi-agent deep deterministic policy option critic (MADDPOC). It is shown that the CT-MADDPG can greatly reduce the complexity of optimization, and the proposed MADDPOC is able to support low-level multi-agent cooperative learning in the continuous action domains, which has great advantages over the existing option-based hierarchical DRL that only supports single-agent learning and discrete actions.
更多查看译文
关键词
Hierarchical,intelligent reflecting surface (IRS),multi-agent deep reinforcement learning (DRL),rechargeable,unmanned aerial vehicle (UAV)
AI 理解论文
溯源树
样例
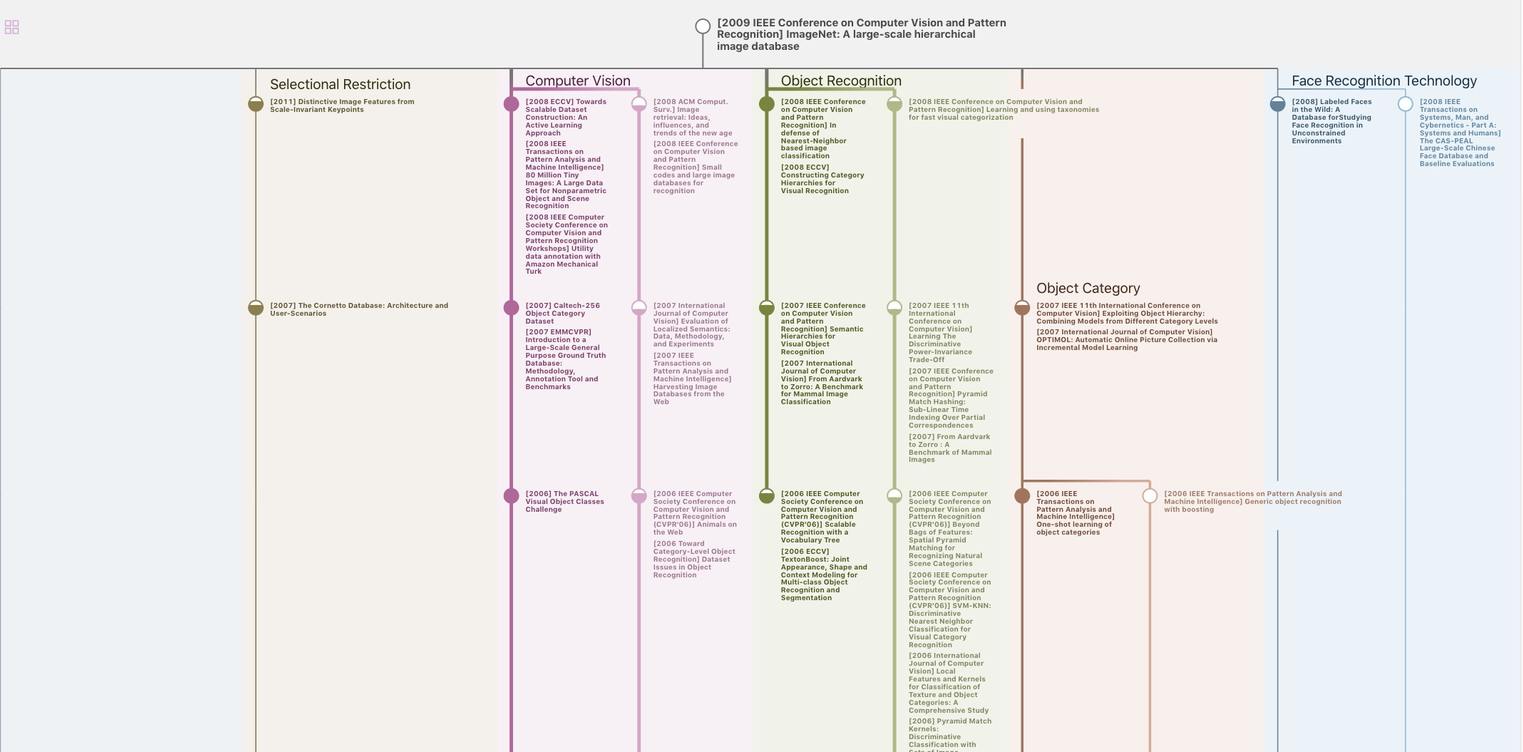
生成溯源树,研究论文发展脉络
Chat Paper
正在生成论文摘要