Dynamic prediction using joint models of longitudinal and recurrent event data: A Bayesian perspective.
Biostatistics & epidemiology(2021)
摘要
In cardiovascular disease (CVD) studies, the events of interest may be recurrent (multiple occurrences from the same individual). During the study follow-up, longitudinal measurements are often available and these measurements are highly predictive of event recurrences. It is of great clinical interest to make personalized prediction of the next occurrence of recurrent events using the available clinical information, because it enables clinicians to make more informed and personalized decisions and recommendations. To this end, we propose a joint model of longitudinal and recurrent event data. We develop a Bayesian approach for model inference and a dynamic prediction framework for predicting target subjects' future outcome trajectories and risk of next recurrent event, based on their data up to the prediction time point. To improve computation efficiency, embarrassingly parallel MCMC (EP-MCMC) method is utilized. It partitions the data into multiple subsets, runs MCMC sampler on each subset, and applies random partition trees to combine the posterior draws from all subsets. Our method development is motivated by and applied to the Antihypertensive and Lipid-Lowering Treatment to Prevent Heart Attack Trial (ALLHAT), one of the largest CVD studies to compare the effectiveness of medications to treat hypertension.
更多查看译文
关键词
ALLHAT study,cardiovascular disease,parallel EP-MCMC,personalized prediction
AI 理解论文
溯源树
样例
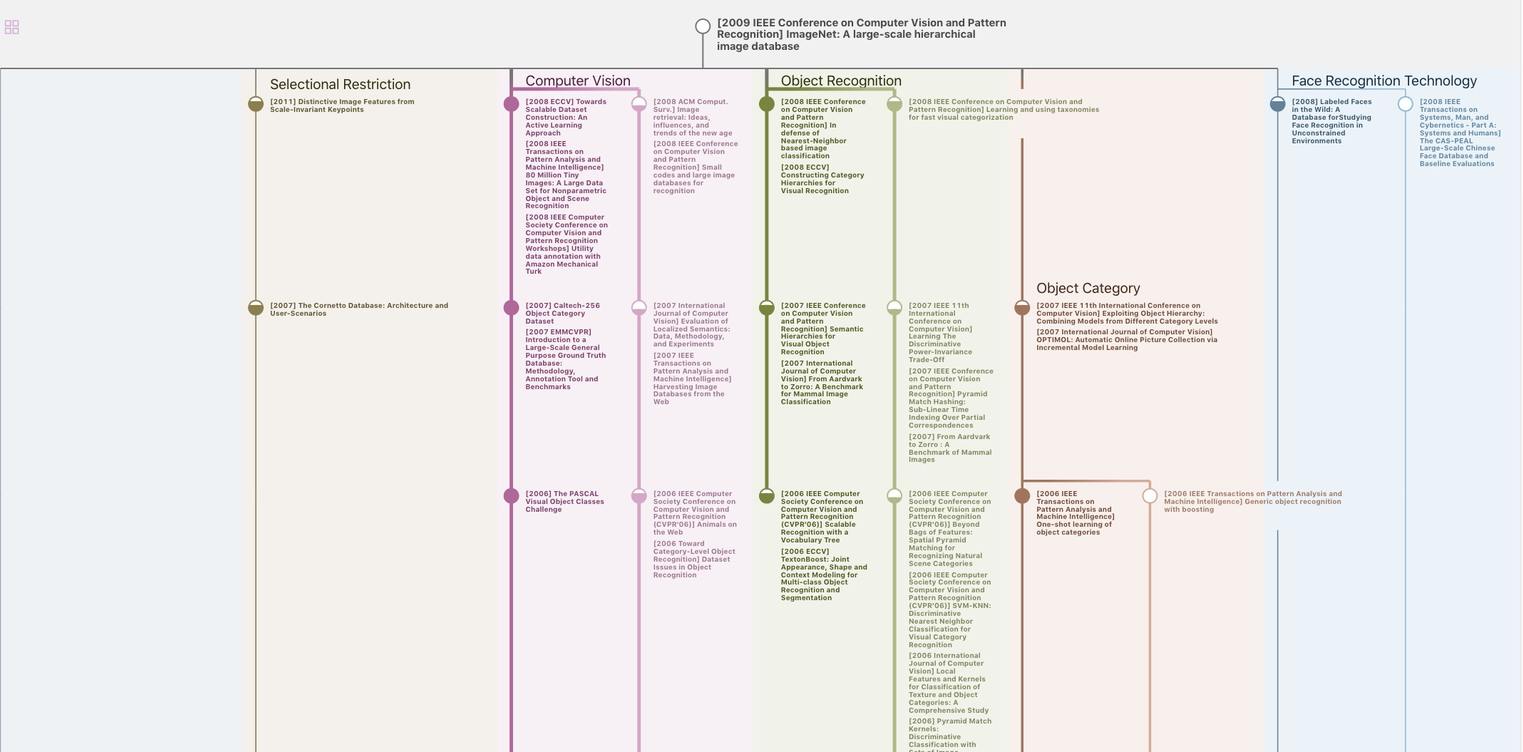
生成溯源树,研究论文发展脉络
Chat Paper
正在生成论文摘要