DB-BERT: A Database Tuning Tool that "Reads the Manual"
PROCEEDINGS OF THE 2022 INTERNATIONAL CONFERENCE ON MANAGEMENT OF DATA (SIGMOD '22)(2022)
摘要
DB-BERT is a database tuning tool that exploits information gained via natural language analysis of manuals and other relevant text documents. It uses text to identify database system parameters to tune as well as recommended parameter values. DB-BERT applies large, pre-trained language models (specifically, the BERT model) for text analysis. During an initial training phase, it fine-tunes model weights in order to translate natural language hints into recommended settings. At run time, DB-BERT learns to aggregate, adapt, and prioritize hints to achieve optimal performance for a specific database system and benchmark. Both phases are iterative and use reinforcement learning to guide the selection of tuning settings to evaluate (penalizing settings that the database system rejects while rewarding settings that improve performance). In our experiments, we leverage hundreds of text documents about database tuning as input for DB-BERT. We compare DB-BERT against various baselines, considering different benchmarks (TPC-C and TPC-H), metrics (throughput and run time), as well as database systems (Postgres and MySQL). In all cases, DB-BERT finds the best parameter settings among all compared methods. The code of DB-BERT is available online at https://itrummer.github.io/dbbert/.
更多查看译文
关键词
database performance tuning, text mining, reinforcement learning
AI 理解论文
溯源树
样例
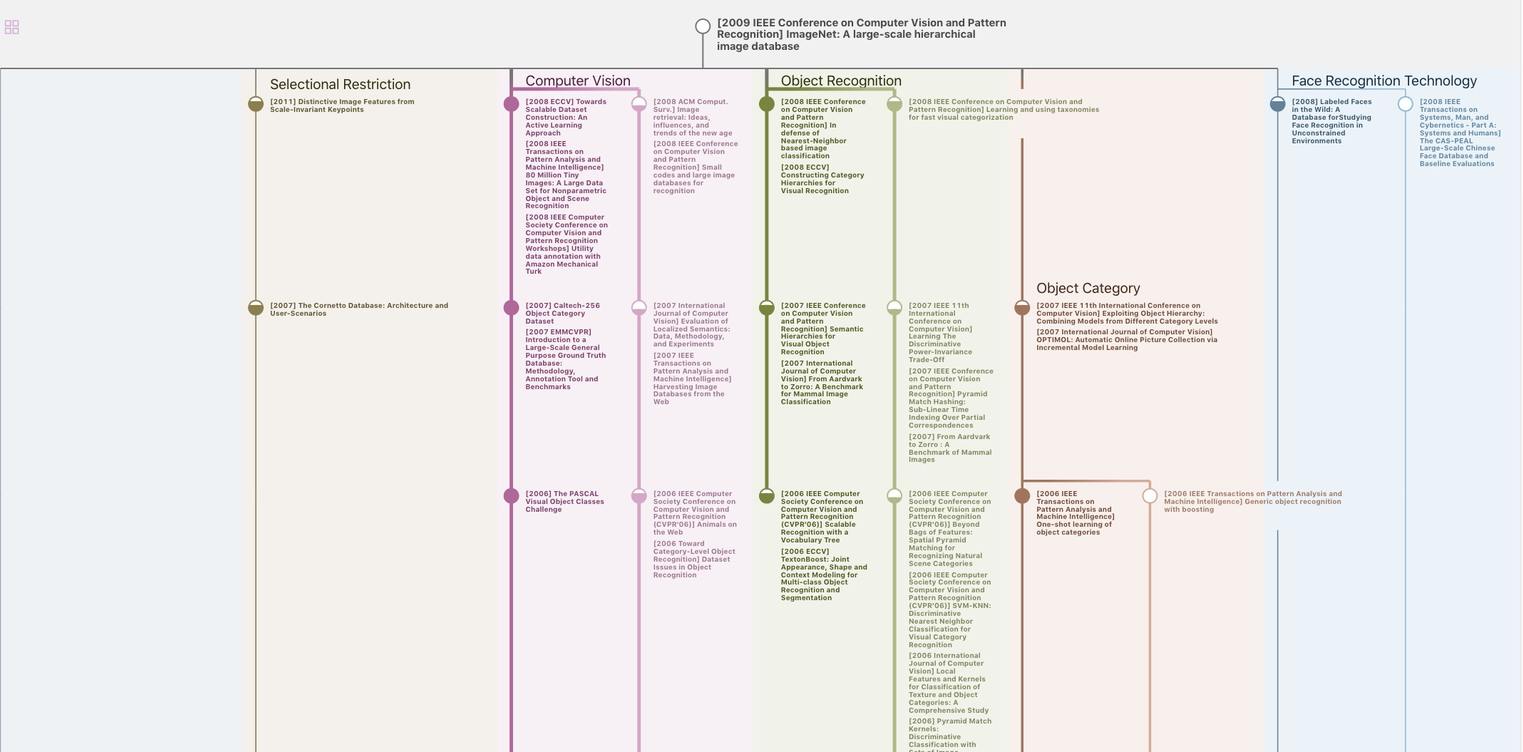
生成溯源树,研究论文发展脉络
Chat Paper
正在生成论文摘要