Understanding and Mitigating the Effect of Outliers in Fair Ranking
WSDM(2022)
摘要
ABSTRACTTraditional ranking systems are expected to sort items in the order of their relevance and thereby maximize their utility. In fair ranking, utility is complemented with fairness as an optimization goal. Recent work on fair ranking focuses on developing algorithms to optimize for fairness, given position-based exposure. In contrast, we identify the potential of outliers in a ranking to influence exposure and thereby negatively impact fairness. An outlier in a list of items can alter the examination probabilities, which can lead to different distributions of attention, compared to position-based exposure. We formalize outlierness in a ranking, show that outliers are present in realistic datasets, and present the results of an eye-tracking study, showing that users scanning order and the exposure of items are influenced by the presence of outliers. We then introduce OMIT, a method for fair ranking in the presence of outliers. Given an outlier detection method, OMIT improves fair allocation of exposure by suppressing outliers in the top-k ranking. Using an academic search dataset, we show that outlierness optimization leads to a fairer policy that displays fewer outliers in the top-k, while maintaining a reasonable trade-off between fairness and utility.
更多查看译文
关键词
Fair ranking, Outliers
AI 理解论文
溯源树
样例
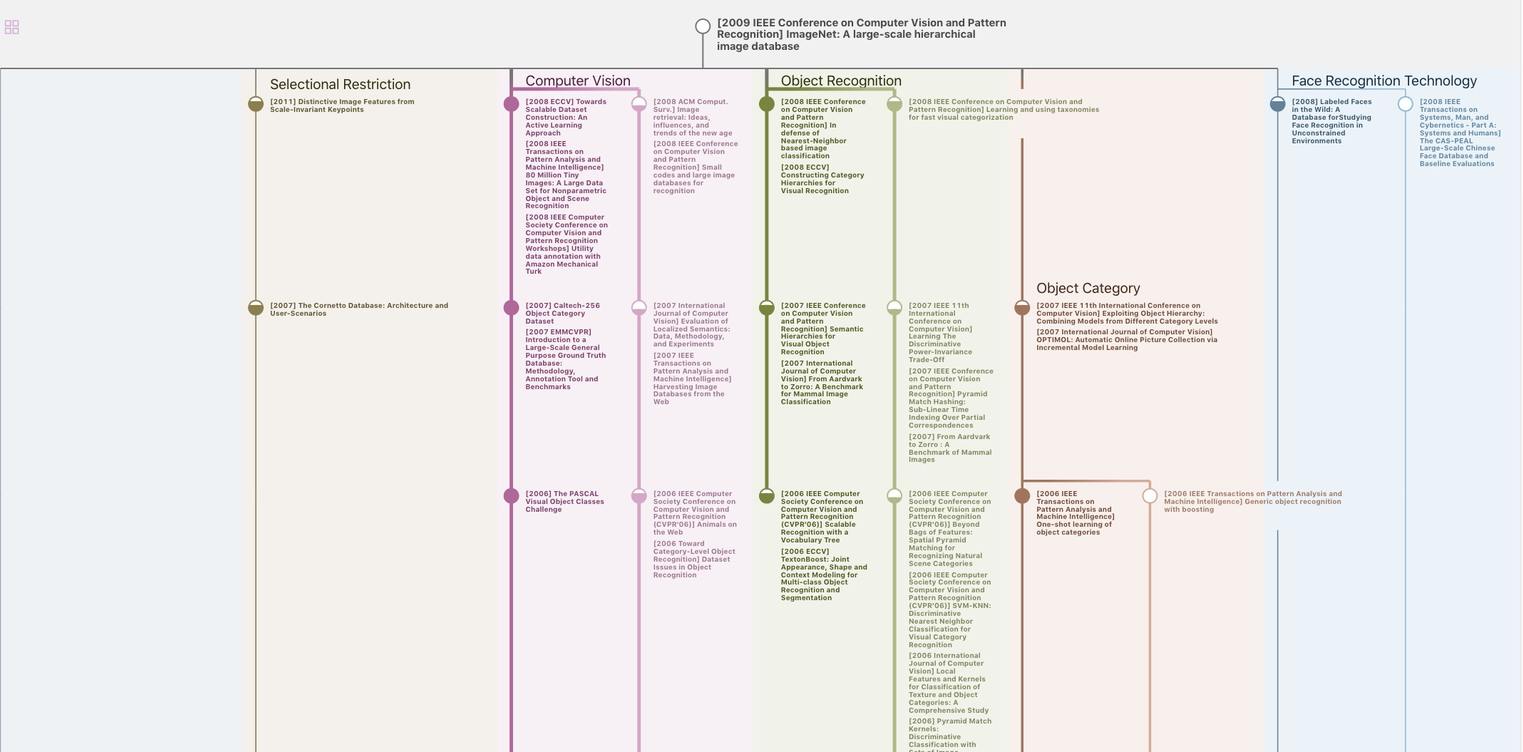
生成溯源树,研究论文发展脉络
Chat Paper
正在生成论文摘要