MIAAIM: Multi-omics image integration and tissue state mapping using topological data analysis and cobordism learning
biorxiv(2021)
摘要
High-parameter tissue imaging enables detailed molecular analysis of single cells in their spatial environment. However, the comprehensive characterization and mapping of tissue states through multimodal imaging across different physiological and pathological conditions requires data integration across multiple imaging systems. Here, we introduce MIAAIM (Multi-omics Image Alignment and Analysis by Information Manifolds) a modular, reproducible computational framework for aligning data across bioimaging technologies, modeling continuities in tissue states, and translating multimodal measures across tissue types. We demonstrate MIAAIM's workflows across diverse imaging platforms, including histological stains, imaging mass cytometry, and mass spectrometry imaging, to link cellular phenotypic states with molecular microenvironments in clinical biopsies from multiple tissue types with high cellular complexity. MIAAIM provides a robust foundation for the development of computational methods to integrate multimodal, high-parameter tissue imaging data and enable downstream computational and statistical interrogation of tissue states.
### Competing Interest Statement
D.S. is a scientific consultant to Roche Glycart AG; none of these relationships are directly related to the topic of this study. R.F.S., J.M.H., P.M.R, and M.C.P. are authors on patent PCT/US21/48928 applied for by the General Hospital Corporation covering the described methods.
更多查看译文
关键词
topological data analysis,state mapping,tissue,multi-omics
AI 理解论文
溯源树
样例
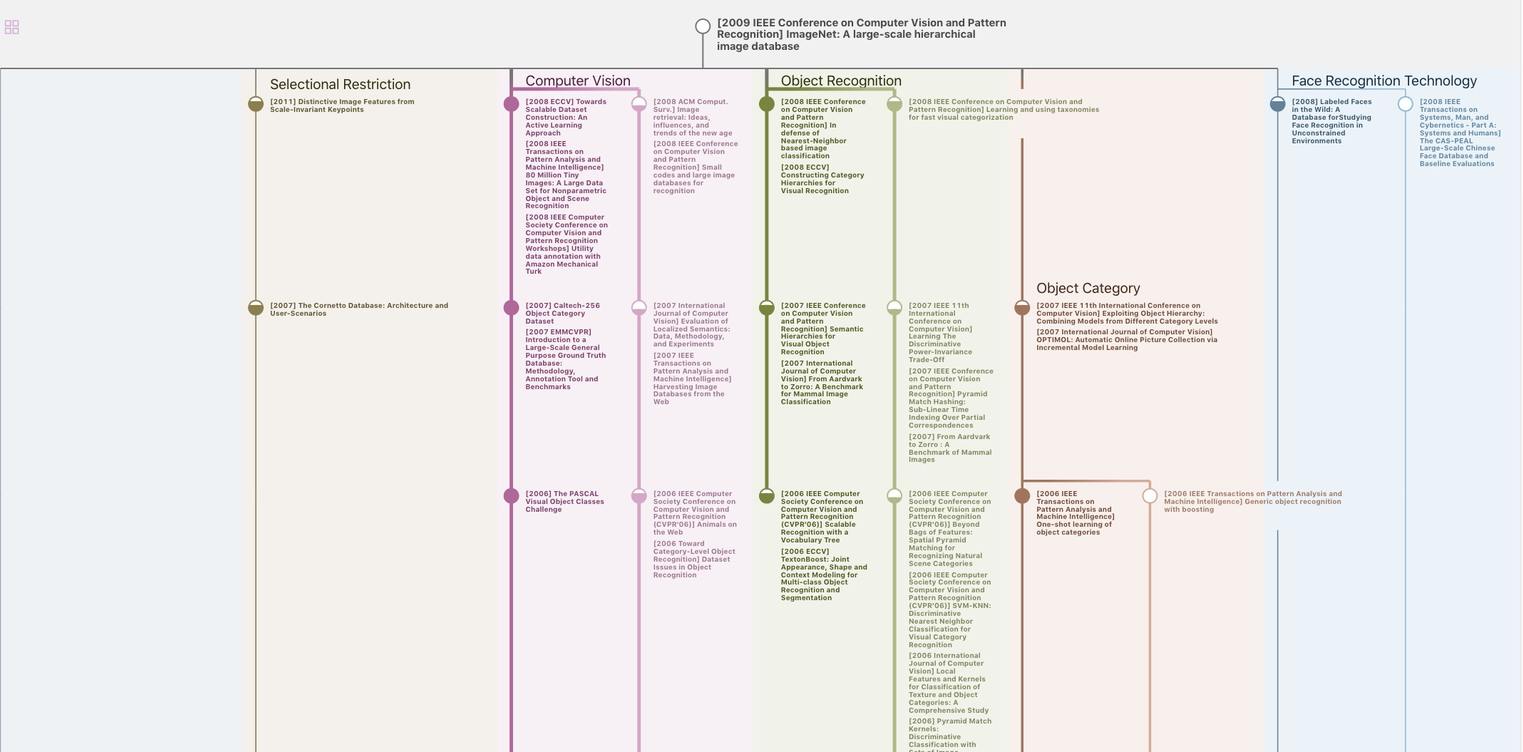
生成溯源树,研究论文发展脉络
Chat Paper
正在生成论文摘要