Accuracy vs. Efficiency: Achieving both Through Hardware-Aware Quantization and Reconfigurable Architecture with Mixed Precision
2021 IEEE Intl Conf on Parallel & Distributed Processing with Applications, Big Data & Cloud Computing, Sustainable Computing & Communications, Social Computing & Networking (ISPA/BDCloud/SocialCom/SustainCom)(2021)
摘要
We propose a hardware/software co-design framework, which leverages hardware-aware quantization and a reconfigurable processor to improve the computational efficiency of convolutional neural networks (CNNs) on tiny IoT devices based on reconfigurable platforms. Firstly, we proposed a multi-objective optimization value function that can weigh accuracy, the size of CNN models, and computational delay, to improve the efficiency of the mixed-precision quantization algorithm based on deep reinforcement learning. Secondly, we propose a reconfigurable CNN processor that can adapt to the computing characteristics of various quantized CNN models, as well as a reconfigurable computing array and an on-chip elastic buffer, to improve the performance and computing efficiency on edge equipment. Finally, we demonstrate the effectiveness of the proposed co-design method through an extensive evaluation of the Ultra96-V2 platform. With respect to the well-known CNNs VGG-16, ResNet-50, and MobileNet-V2, the experimental result shows that the throughput of 216.6 GOPS, 214.0 GOPS, and 53.6 GOPS, the computing efficiency of 0.63GOPS/DSP, 0.64GOPS/DSP, and 0.24 GOPS/DSP, respectively. In addition, achieving a better optimized trade-off between the computing efficiency and accuracy compared with the recently proposed CNN processor with fixed bit-width and mixed-precision.
更多查看译文
关键词
hardware/software co-design,quantization,reconfigurable CNN processor
AI 理解论文
溯源树
样例
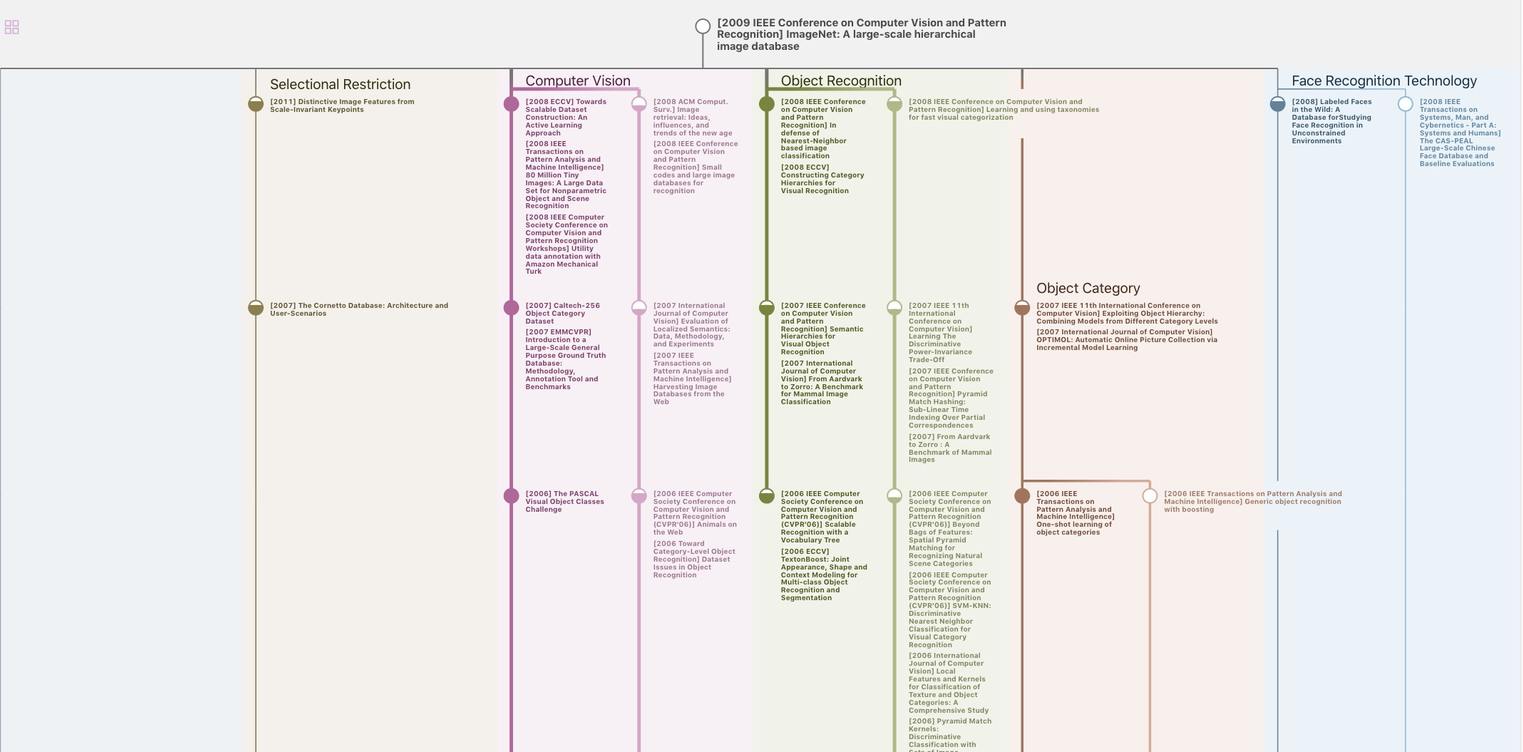
生成溯源树,研究论文发展脉络
Chat Paper
正在生成论文摘要